Unsupervised Domain Adaptation for Semantic Segmentation with Symmetric Adaptation Consistency
ICASSP 2020 - 2020 IEEE International Conference on Acoustics, Speech and Signal Processing (ICASSP)(2020)
摘要
Unsupervised domain adaptation, which leverages label information from other domains to solve tasks on a domain without any labels, can alleviate the problem of the scarcity of labels and expensive labeling costs faced by supervised semantic segmentation. In this paper, we utilize adversarial learning and semi-supervised learning simultaneously to solve the task of unsupervised domain adaptation in semantic segmentation. We propose a new approach that trains two segmentation models with the adversarial learning symmetrically and further introduces the consistency between the outputs of the two models into the semi-supervised learning to improve the accuracy of pseudo labels which significantly affect the final adaptation performance. We achieve state-of-the-art semantic segmentation performance on the GTA5-to-Cityscapes scenario, a widely used benchmark setting in unsupervised domain adaptation.
更多查看译文
关键词
Unsupervised domain adaptation,urban scene semantic segmentation,adversarial learning,semi-supervised learning,symmetric adaptation consistency
AI 理解论文
溯源树
样例
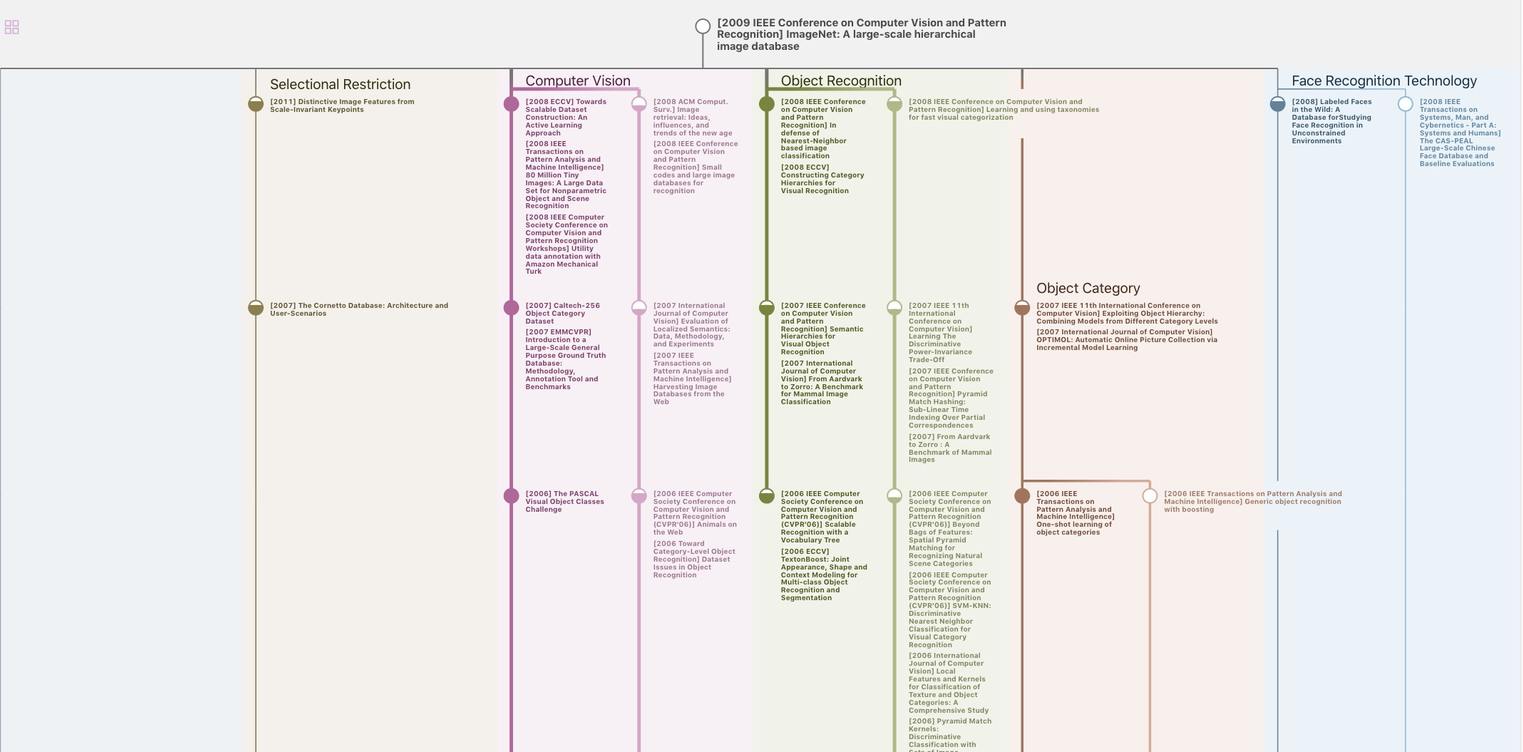
生成溯源树,研究论文发展脉络
Chat Paper
正在生成论文摘要