SPECTRANET-SO(3): Learning Satellite Orientation from Optical Spectra by Implicitly Modeling Mutually Exclusive Probability Distributions on The Rotation Manifold
ICASSP 2023 - 2023 IEEE International Conference on Acoustics, Speech and Signal Processing (ICASSP)(2023)
摘要
In the space domain, remotely measuring the rotational state of a spacecraft provides critical information for assessing its operational health. For the large family of space assets that lie in regions of space too distant to resolve spatial features with ground sensors, measurement of the energy spectrum of reflected sunlight has shown recent promise in probing their material and spatial properties. In this work, we explore the utility of using the recently proposed Implicit-PDF network to implicitly learn challenging probability distributions associated with satellite orientations using only raw optical spectra as input. Originally designed for computer vision applications, we detail how the Implicit-PDF architecture can be applied directly to spectra and further extended to a broad class of signal inputs. We discuss key aspects to improving performance and demonstrate the generalizability of the implicit framework by showing how a single network can efficiently learn mutually exclusive probability distributions over multiple satellite classes without meaningful performance loss.
1
更多查看译文
关键词
spectroscopy,Space Domain Awareness (SDA),Implicit-PDF,SO(3),non-resolved object characterization
AI 理解论文
溯源树
样例
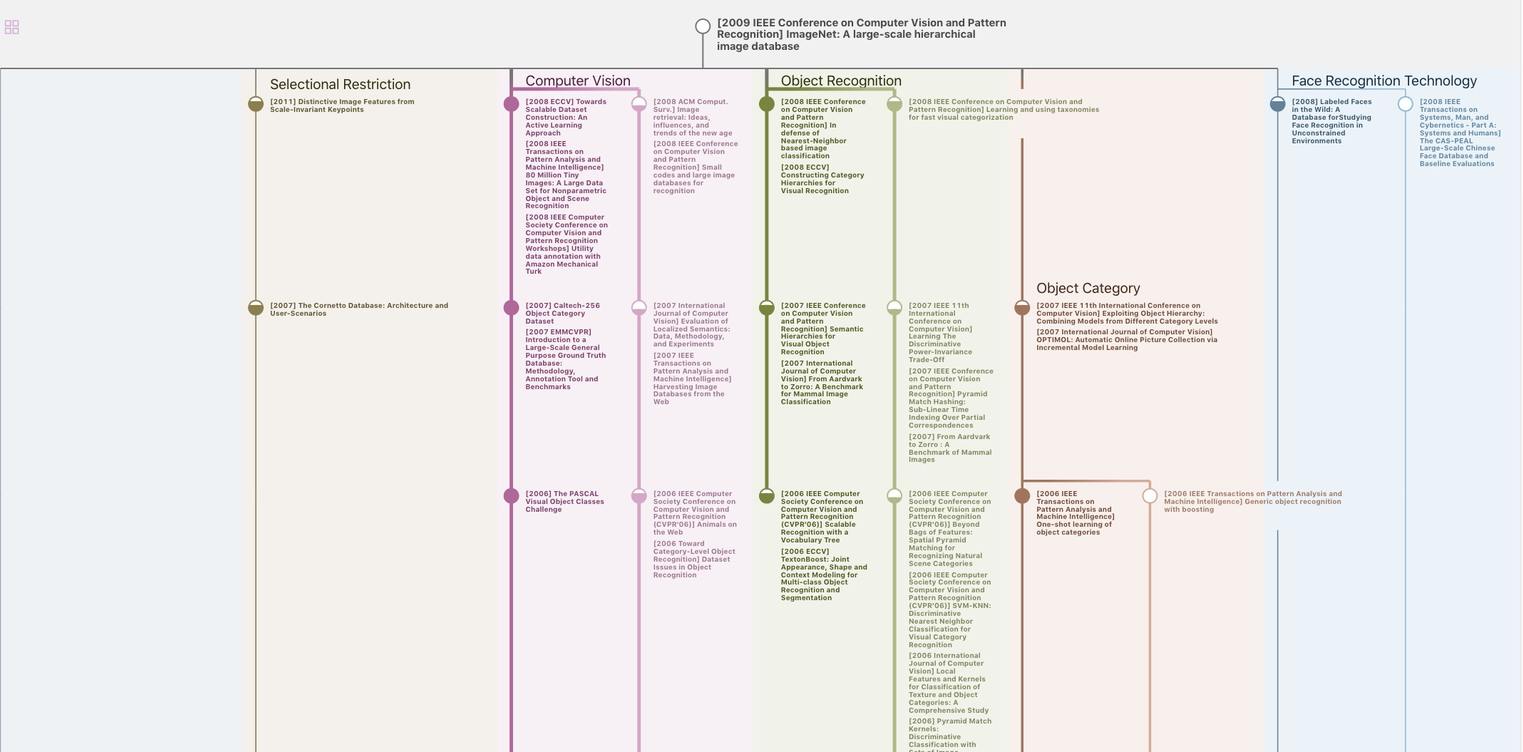
生成溯源树,研究论文发展脉络
Chat Paper
正在生成论文摘要