Learning Silhouettes with Group Sparse Autoencoders
ICASSP 2023 - 2023 IEEE International Conference on Acoustics, Speech and Signal Processing (ICASSP)(2023)
摘要
Sparse coding has been extensively used in neuroscience to model brain-like computation by drawing analogues between neurons’ firing activity and the nonzero elements of sparse vectors. Contemporary deep learning architectures have been used to model neural activity, inspired by signal processing algorithms; however sparse coding architectures are not able to explain the higher-order categorization that has been empirically observed at the neural level. In this work, we propose a novel model-based architecture, termed group-sparse autoencoder, that produces sparse activity patterns in line with neural modeling, but showcases a higher-level order in its activation maps. We evaluate a dense model of our architecture on MNIST and CIFAR-10 and show that it learns dictionaries that resemble silhouettes of the given class, while its activations have a significantly higher level order compared to sparse architectures. Source code is available at: https://github.com/manosth/silhouette-learning.
更多查看译文
关键词
Unrolled optimization,group sparsity,neural categorical representation,silhouette learning
AI 理解论文
溯源树
样例
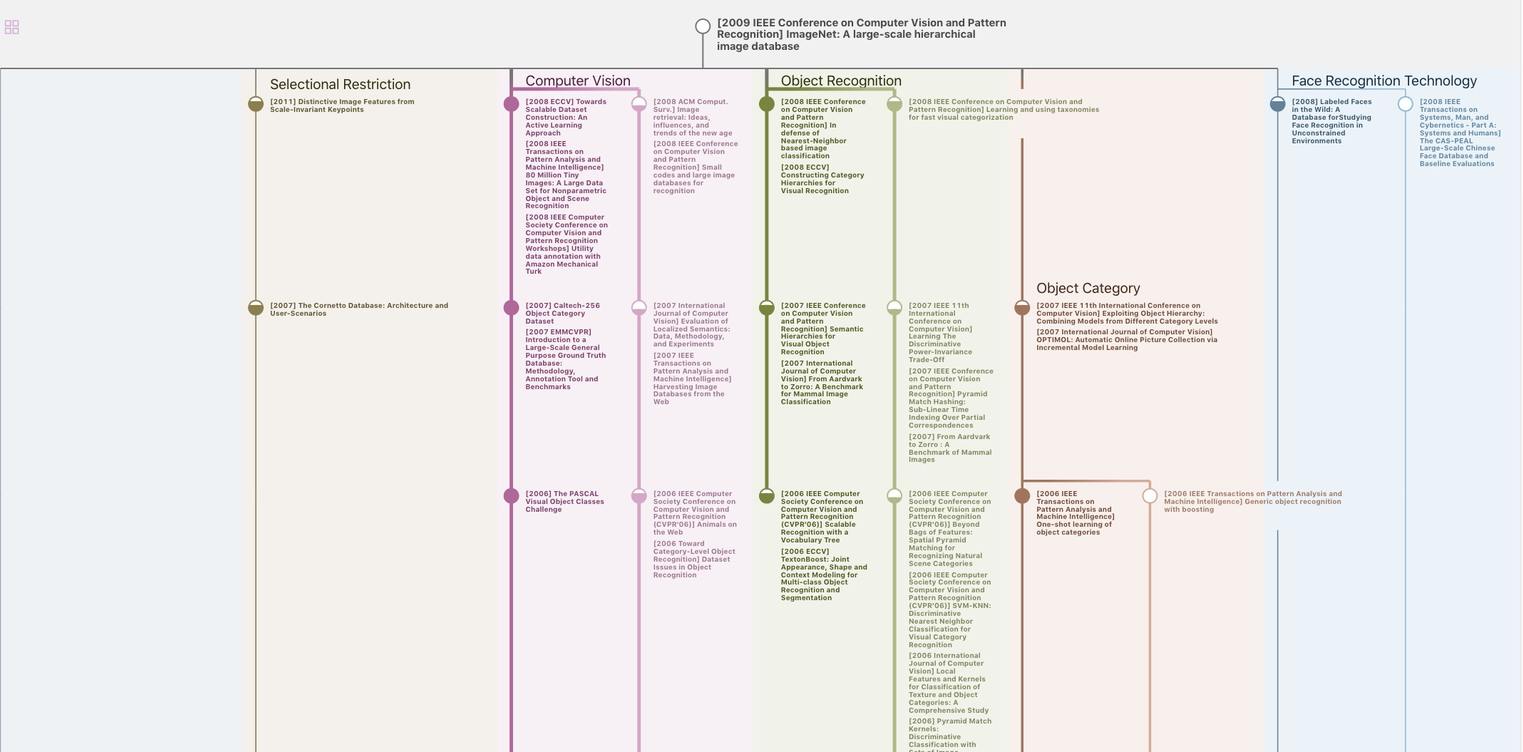
生成溯源树,研究论文发展脉络
Chat Paper
正在生成论文摘要