Higher-Order Spatio-Temporal Neural Networks for Covid-19 Forecasting
ICASSP 2023 - 2023 IEEE International Conference on Acoustics, Speech and Signal Processing (ICASSP)(2023)
摘要
Coronavirus Disease 2019 (COVID-19) pneumonia started in December 2019 and cases have been reported in 240 countries/regions with more than 570 million confirmed cases and more than 6 million deaths which caused large casualties and huge economic losses. To enhance the understanding of the levels of COVID-19 transmission and infection, and the effects of treatments and interventions, high-quality spatio-temporal COVID-19 datasets and accurate multivariate time-series forecasting models for COVID-19 case prediction play crucial roles. In this paper, we present the COVID-19 spatio-temporal graph (COV19-STG) datasets, i.e., spatio-temporal United States COVID-19 graph datasets on the county-level. By using these datasets, we propose Higher-order Spatio-temporal Neural Networks (HOST-NETs) to further improve the accuracy of predicting COVID-19 trends. Specifically, we incorporate higher-order structure to build a simplicial complex representation learning module, and integrate it into a spatio-temporal neural network architecture, thus leveraging both global and local topological information. Experimental results show that our model consistently outperforms previous state-of-the-art models.
更多查看译文
关键词
Simplicial Complex,COVID-19,Deep Learning
AI 理解论文
溯源树
样例
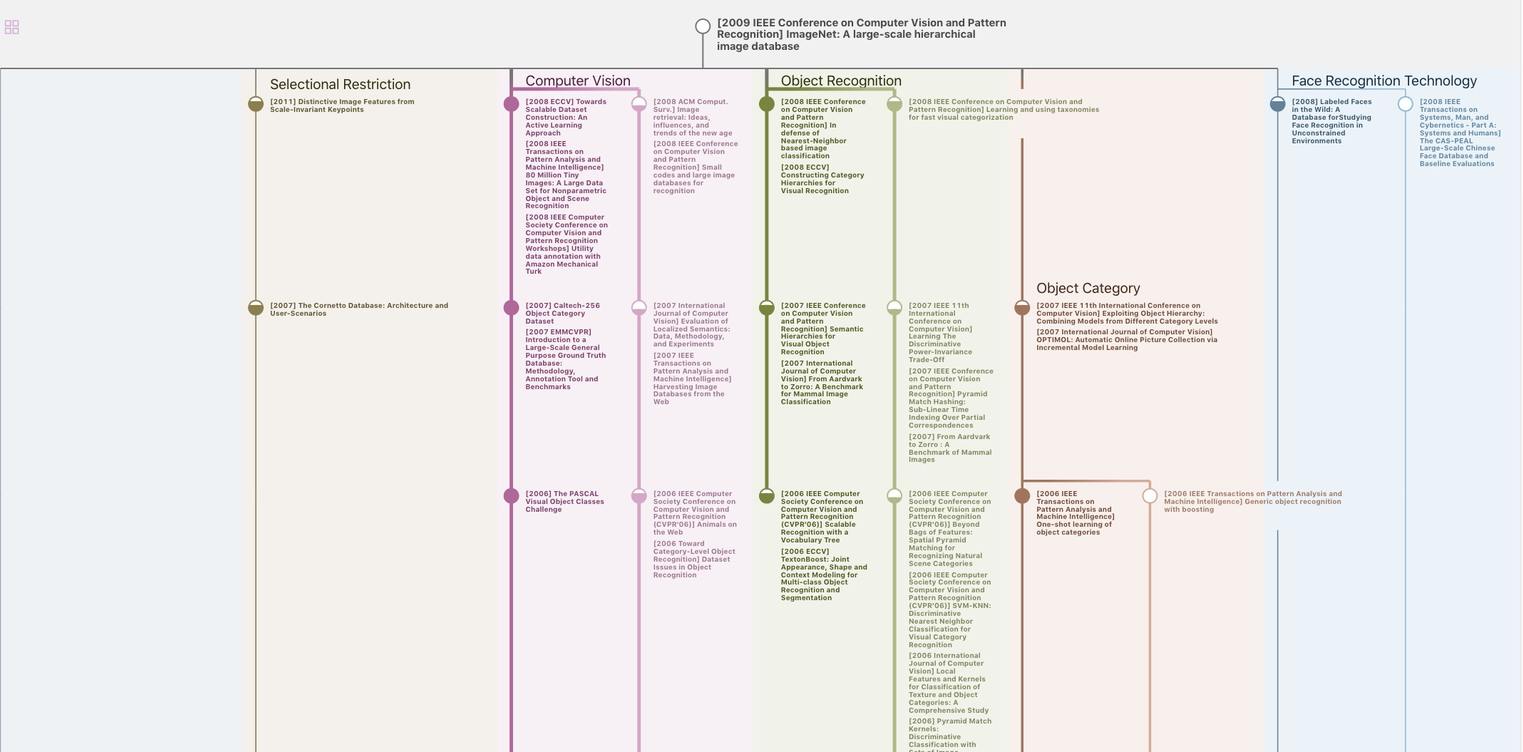
生成溯源树,研究论文发展脉络
Chat Paper
正在生成论文摘要