Accelerated Distributed Stochastic Non-Convex Optimization over Time-Varying Directed Networks
ICASSP 2023 - 2023 IEEE International Conference on Acoustics, Speech and Signal Processing (ICASSP)(2023)
摘要
We study non-convex optimization problems where the data is distributed across nodes of a time-varying directed network; this describes dynamic settings in which the communication between network nodes is affected by delays or link failures. The network nodes, which can access only their local objectives and query a stochastic first-order oracle for the gradient estimates, collaborate by exchanging messages with their neighbors to minimize a global objective function. We propose an algorithm for non-convex optimization problems in such settings that leverages stochastic gradient descent with momentum and gradient tracking. We further prove, by analyzing dynamic network systems with gradient acceleration, that the oracle complexity of the proposed algorithm is $\mathcal{O}\left( {1/{\varepsilon ^{1.5}}} \right)$. The results demonstrate superior performance of the proposed framework compared to state-of-the-art related methods used in a variety of machine learning tasks.
更多查看译文
关键词
distributed non-convex optimization,stochastic non-convex optimization,time-varying directed networks
AI 理解论文
溯源树
样例
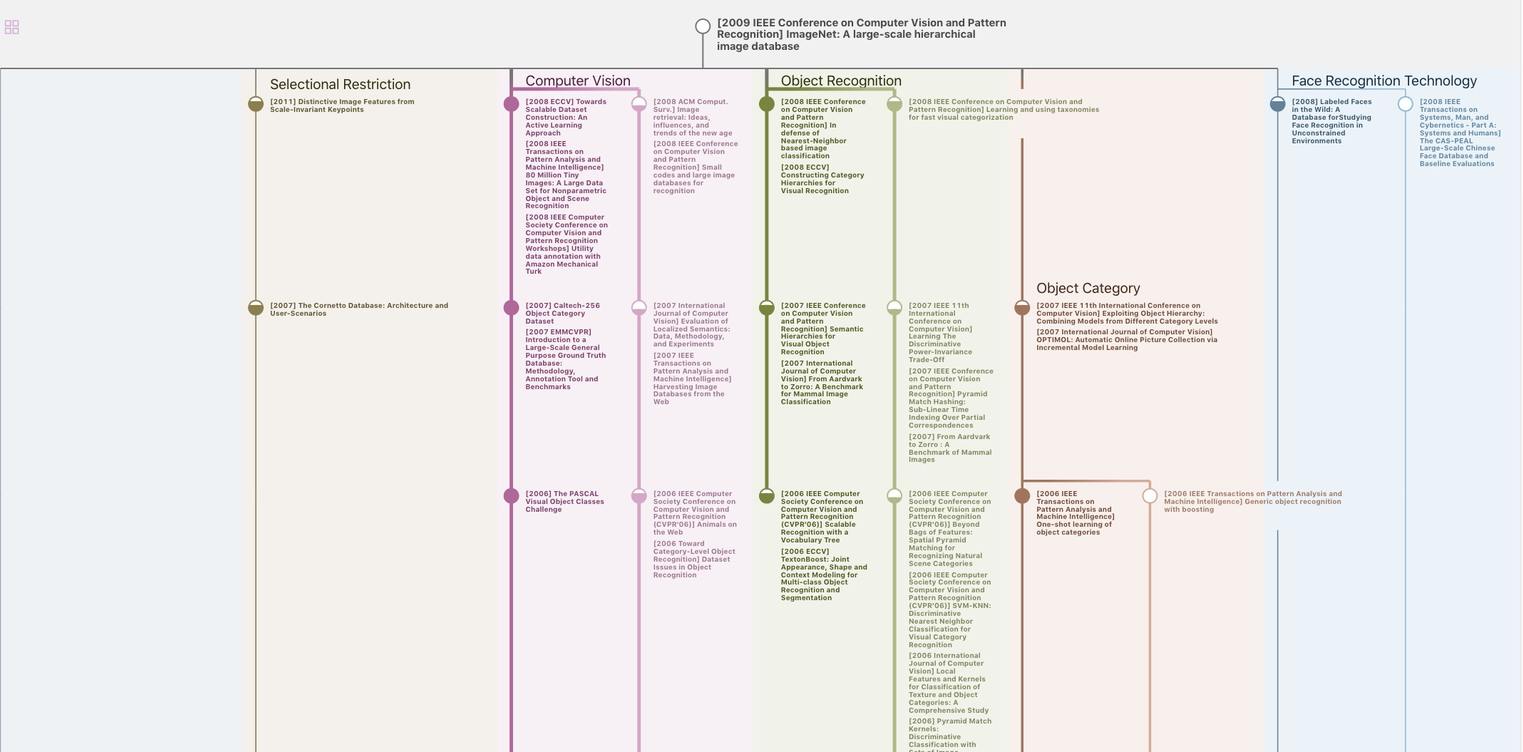
生成溯源树,研究论文发展脉络
Chat Paper
正在生成论文摘要