Sign Language Recognition via Deformable 3D Convolutions and Modulated Graph Convolutional Networks
ICASSP 2023 - 2023 IEEE International Conference on Acoustics, Speech and Signal Processing (ICASSP)(2023)
摘要
Automatic sign language recognition (SLR) remains challenging, especially when employing RGB video alone (i.e., with no depth or special glove-based input) and under a signer-independent (SI) framework, due to inter-personal signing variation. In this paper, we address SI isolated SLR from RGB video, proposing an innovative deep-learning framework that leverages multi-modal appearanceand skeleton-based information. Specifically, we propose three components for the first time in SLR: (i) a modified version of the ResNet2+1D network to capture signing appearance information, where spatial and temporal convolutions are substituted by their deformable counterparts, accomplishing both prevalent spatial modeling potential and motion-aware modeling adaptability; (ii) a novel spatio-temporal graph convolutional network (ST-GCN) that integrates a GCN variant, involving weight and affinity modulation for modeling diverse correlations between different body joints beyond the physical human skeleton structure, followed by a self-attention layer and a temporal convolution; and (iii) the “PIXIE” 3D human pose and shape regressor to generate 3D joint-rotation parameterization used for ST-GCN graph construction. Both appearance- and skeleton-based streams are ensembled in the proposed system and evaluated on two datasets of isolated signs, one in Turkish and one in Greek. Our system outperforms the state-of-the-art on the second set, yielding 53% relative error rate reduction (2.45% absolute), while it performs on par with the best reported system on the first.
更多查看译文
关键词
SI isolated sign language recognition,deformable 3D-CNN,ST-GCN,modulated GCN,"PIXIE"
AI 理解论文
溯源树
样例
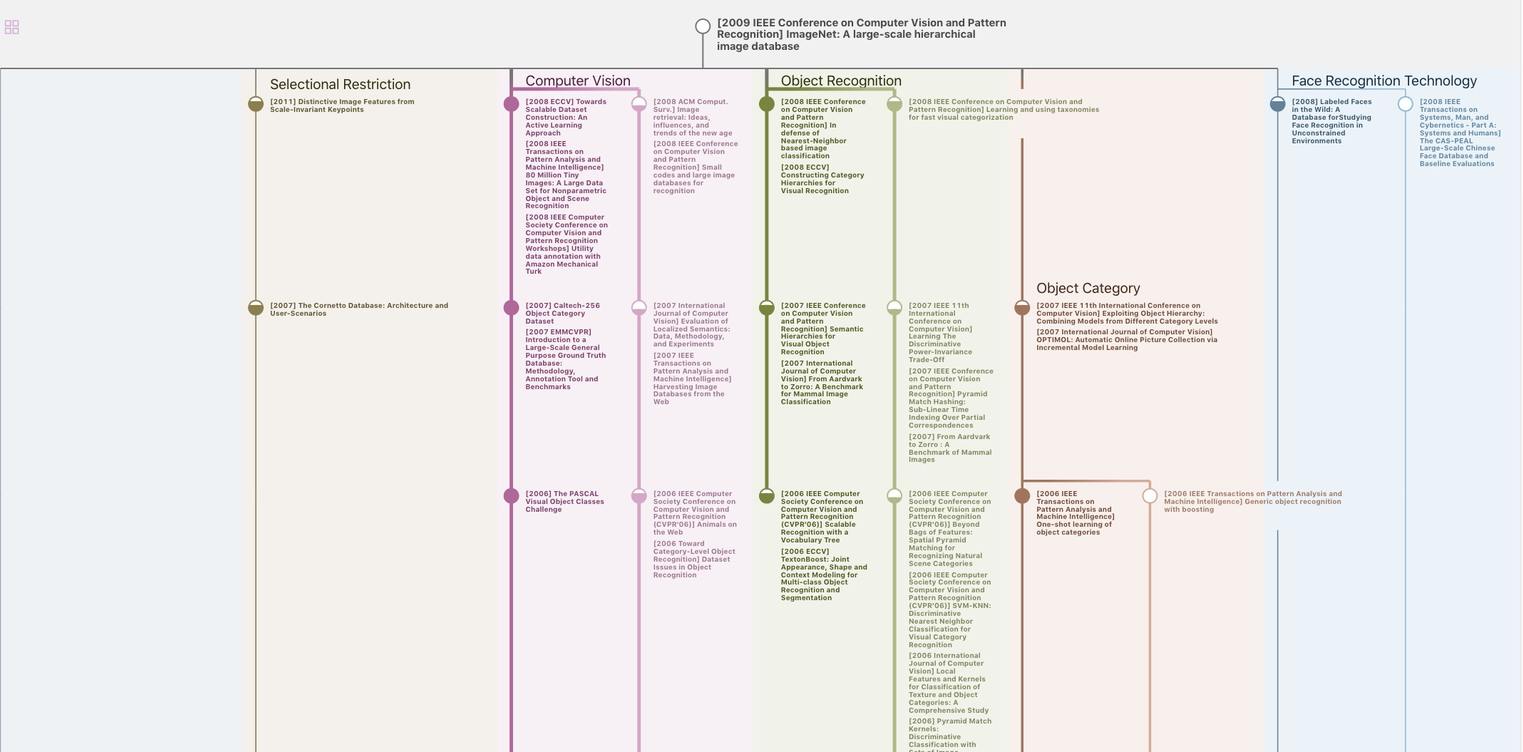
生成溯源树,研究论文发展脉络
Chat Paper
正在生成论文摘要