Clean Sample Guided Self-Knowledge Distillation for Image Classification
ICASSP 2023 - 2023 IEEE International Conference on Acoustics, Speech and Signal Processing (ICASSP)(2023)
摘要
For two-stage knowledge distillation, the combination with Data Augmentation (DA) is straightforward and effective. Yet, for online Self-knowledge Distillation (SD), DA is not always beneficial because of the absence of a trustworthy teacher model. To address this issue, this paper proposes an SD method named Clean sample guided Self-knowledge Distillation (CleanSD), in which the original clean sample is used as a guide when the model is trained with the augmented samples. The implementation of the CleanSD comes with two DA techniques, namely Mixup (for label-mixing) and Cutout (for label-preserving). Results on CIFAR-100 demonstrate that error rates obtained by the proposed CleanSD are reduced by 2.59%, 1.39%, and 0.47-1.20%, compared to that obtained by the baseline, the vanilla DA techniques, and other peer SD methods, respectively. In addition, the effectiveness and robustness of the CleanSD are verified across multiple DA methods and datasets.
更多查看译文
关键词
self-knowledge distillation,mixup,cutout,clean sample,data augmentation
AI 理解论文
溯源树
样例
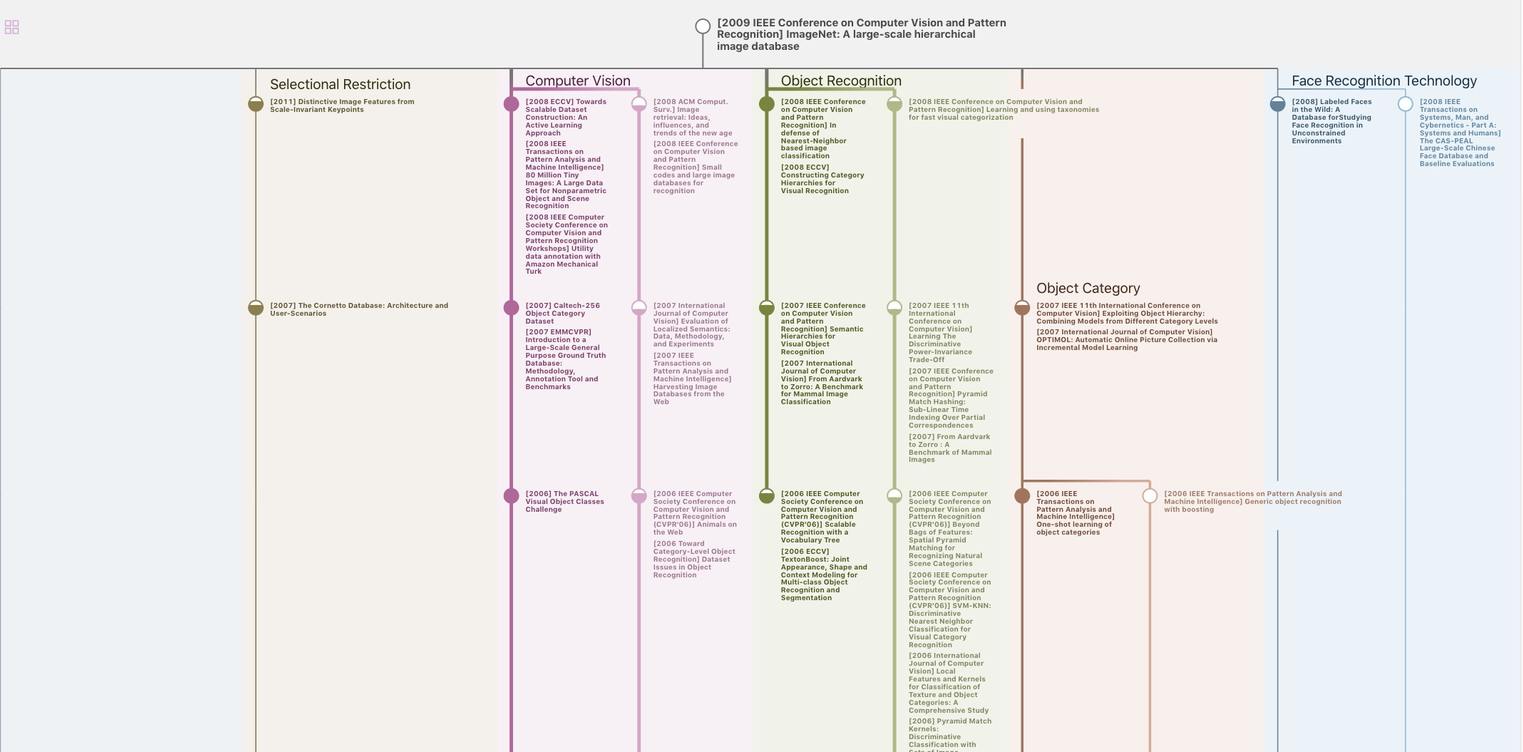
生成溯源树,研究论文发展脉络
Chat Paper
正在生成论文摘要