Incorporating Reliability in Graph Information Propagation by Fluid Dynamics Diffusion: A case of Multimodal Semisupervised Deep Learning
ICASSP 2023 - 2023 IEEE International Conference on Acoustics, Speech and Signal Processing (ICASSP)(2023)
摘要
Classic graph neural networks show some limitations in information extraction performance when applied to multimodal datasets. This is primarily due to such datasets having high volume, variety, and variability. In this paper, we propose structuring graph neural networks on a new graph representation based on fluid dynamics diffusion that allows us to incorporate the reliability of the features used to characterise each sample within the graph structure itself. This approach aims to address some of the major limitations of the classic graph-based learning structures, so to improve accuracy and robustness of the estimates. We show how this approach can help to strongly improve the quality of the analysis of classic graph neural networks. Experimental results are reported to support this point.
更多查看译文
关键词
Graph-based data analysis,fluid diffusion,multimodal data,semisupervised deep learning
AI 理解论文
溯源树
样例
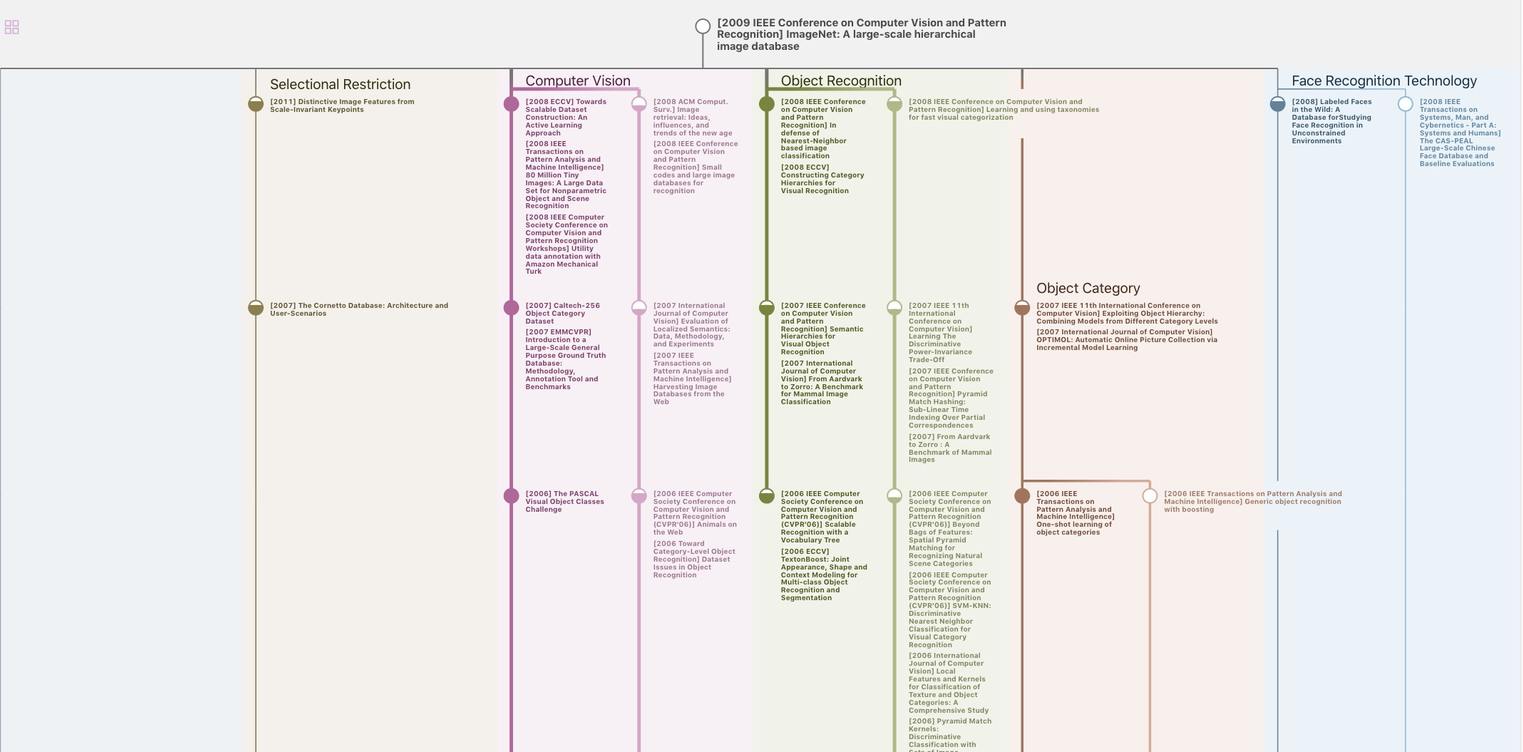
生成溯源树,研究论文发展脉络
Chat Paper
正在生成论文摘要