SafeDeep: A Scalable Robustness Verification Framework for Deep Neural Networks
ICASSP 2023 - 2023 IEEE International Conference on Acoustics, Speech and Signal Processing (ICASSP)(2023)
摘要
The state-of-the-art machine learning techniques come with limited, if at all any, formal correctness guarantees. This has been demonstrated by adversarial examples in the deep learning domain. To address this challenge, here, we propose a scalable robustness verification framework for Deep Neural Networks (DNNs). The framework relies on Linear Programming (LP) engines and builds on decades of advances in the field for analyzing convex approximations of the original network. The key insight is in the on-demand incremental refinement of these convex approximations. This refinement can be parallelized, making the framework even more scalable. We have implemented a prototype tool to verify the robustness of a large number of DNNs in epileptic seizure detection. We have compared the results with those obtained by two state-of-the-art tools for the verification of DNNs. We show that our framework is consistently more precise than the over-approximation-based tool ERAN and more scalable than the SMT-based tool Reluplex.
更多查看译文
关键词
DNNs,verification,approximation,refinement,linear programming,robustness
AI 理解论文
溯源树
样例
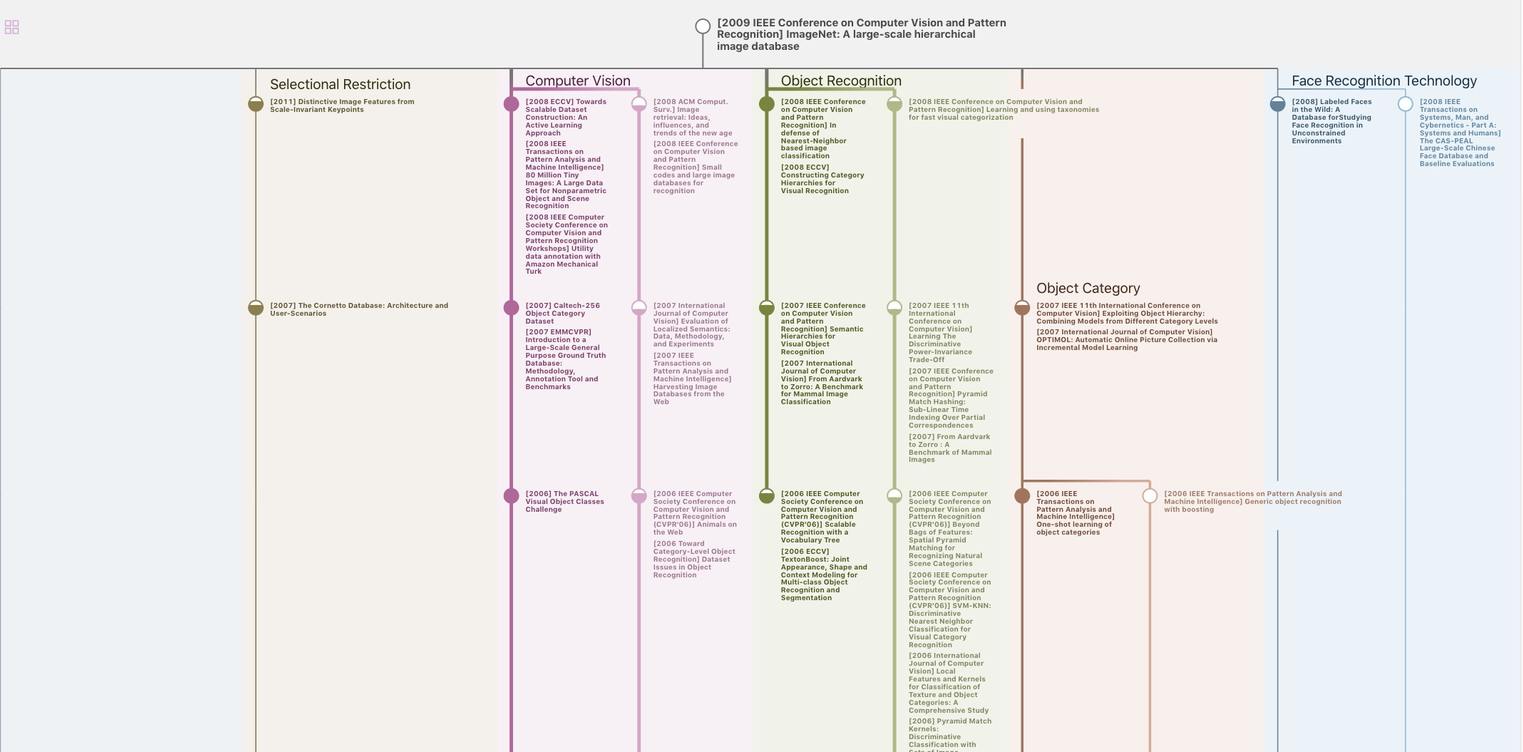
生成溯源树,研究论文发展脉络
Chat Paper
正在生成论文摘要