Graph Contrastive Learning with Learnable Graph Augmentation
ICASSP 2023 - 2023 IEEE International Conference on Acoustics, Speech and Signal Processing (ICASSP)(2023)
摘要
Graph contrastive learning has gained popularity due to its success in self-supervised graph representation learning. Augmented views in contrastive learning greatly determine the quality of the learned representations. Handcrafted data augmentations in previous work require tedious trial-and- errors per dataset, which is time-consuming and resource-intensive. Here, we propose a Graph Contrastive learning framework with Learnable graph Augmentation called GraphCLA. Specifically, learnable graph augmentation trains augmented views by minimizing the mutual information (MI) between the input graphs and their augmented graphs. This paper designs a kernel contrastive loss function based on an end-to-end differentiable graph kernel to learn augmented views. In addition, this paper utilizes a min-max optimization strategy to learn challenging augmented graphs and to learn discriminative representations adversarially. Finally, we compared GraphCLA with state-of-the-art self-supervised learning baselines and experimentally validate the effectiveness of GraphCLA.
更多查看译文
关键词
Contrastive learning,graph representation learning,data augmentation
AI 理解论文
溯源树
样例
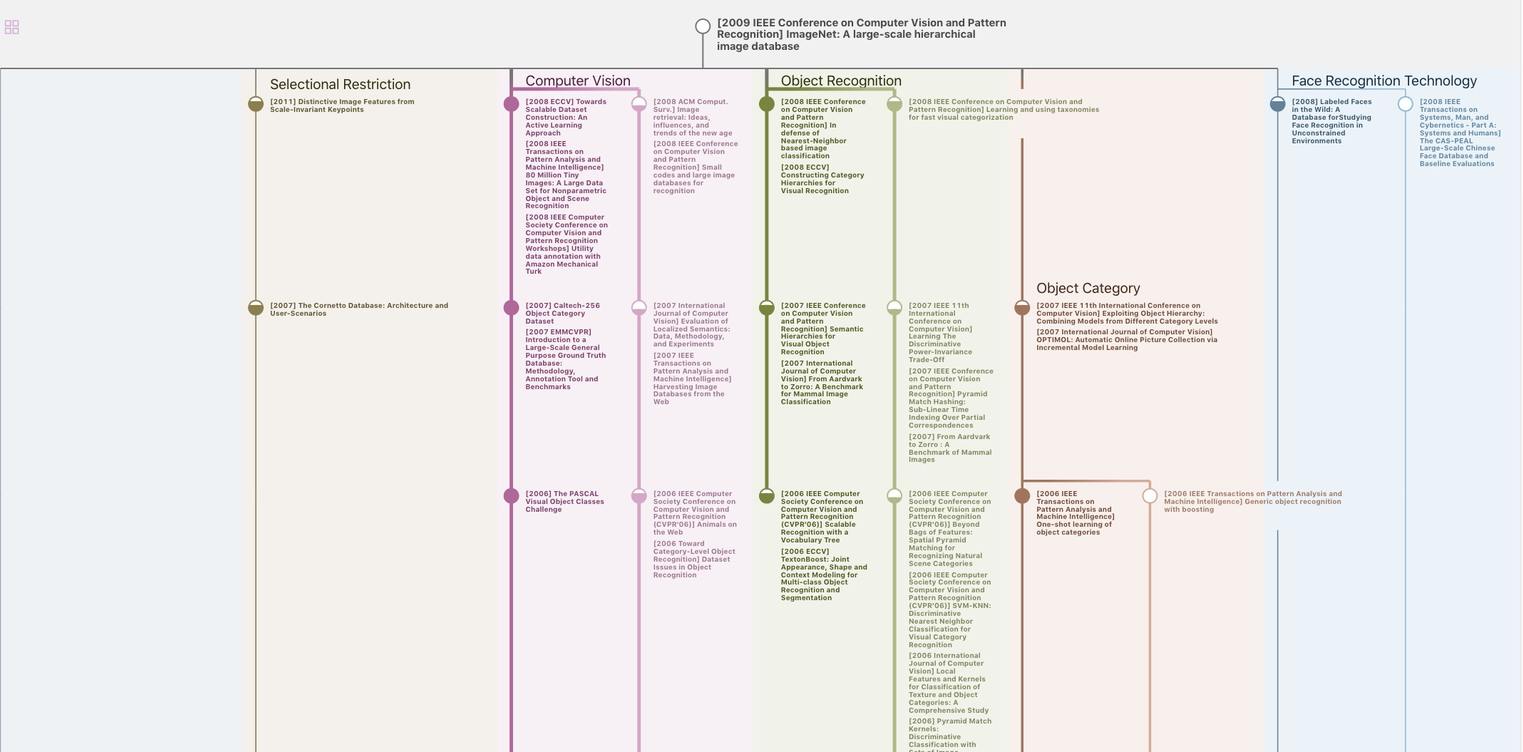
生成溯源树,研究论文发展脉络
Chat Paper
正在生成论文摘要