Joint Discriminator and Transfer Based Fast Domain Adaptation For End-To-End Speech Recognition
ICASSP 2023 - 2023 IEEE International Conference on Acoustics, Speech and Signal Processing (ICASSP)(2023)
摘要
Adapting End-to-End (E2E) models to unseen domains is still a big challenge since training E2E models requires lots of paired audio and text training data. We propose a novel domain adaptation framework for the E2E model, which only uses the text of the target domain. Moreover, the proposed methods can keep the performance on the source domain intact while greatly improving the performance on the target domain. The proposed framework consists of two parts: the discriminator and the transfer which were optimized separately. Finally, optimized discriminator and transfer were combined and evaluated on two domain adaption tasks. In the experiments of adapting the English Librispeech to Gigaspeech, we obtained an average relative 11.6% and 11.8% on word error rate (WER) reduction for the target domain dev and test sets, respectively, while almost without WER degradation on the source domain. For the inhouse Chinese corpus aviation and TV, the character error rate (CER) of the source domain increased within 5%, while the CER on the target domain achieved around relative 85% and 42% improvement, respectively. In addition, our approach is also more effective in the mixed domain scenarios in the evaluation.
更多查看译文
关键词
end-to-end speech recognition,domain adaptation,discriminator and transfer,log-likelihood ratio
AI 理解论文
溯源树
样例
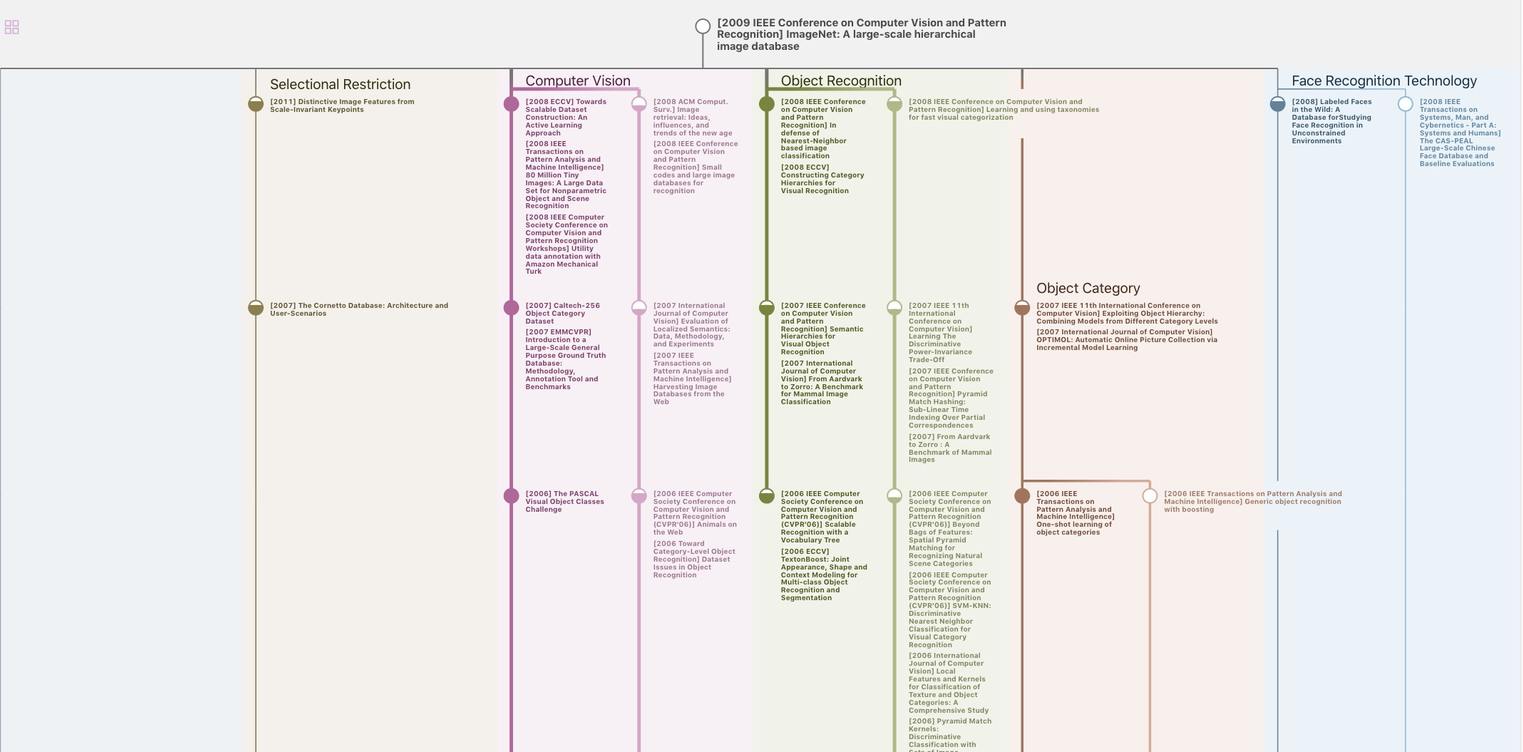
生成溯源树,研究论文发展脉络
Chat Paper
正在生成论文摘要