Eigen-Decomposition-Free Directed Graph Sampling via Gershgorin Disc Alignment
ICASSP 2023 - 2023 IEEE International Conference on Acoustics, Speech and Signal Processing (ICASSP)(2023)
摘要
Graph sampling is the problem of choosing a node subset via sampling matrix H ∈ {0, 1}
K×N
to collect samples y = Hx ∈ℝ
K
, K < N, so that the target signal x ∈ ℝ
N
can be reconstructed in high fidelity. While sampling on undirected graphs is well studied, we propose the first eigen-decomposition-free sampling scheme tailored specifically for directed graphs, leveraging a previous undirected graph sampling method based on Gershgorin disc alignment (GDAS). Concretely, given a directed positive graph ${{\mathcal{G}}^d}$ specified by random-walk graph Laplacian matrix L
rw
, we first define reconstruction of a smooth signal x
∗
from samples y using graph shift variation (GSV) $\left\| {{{\mathbf{L}}_{rw}}{\mathbf{x}}} \right\|_2^2$ as a signal prior. To minimize the worst-case reconstruction error of the linear system solution x
∗
= C
−1
H
⊤
y with symmetric coefficient matrix${\mathbf{C}} = {{\mathbf{H}}^ \top }{\mathbf{H}} + \mu {\mathbf{L}}_{rw}^ \top {{\mathbf{L}}_{rw}}$, the E-optimality sampling objective is to choose H to maximize the smallest eigenvalue λ
min
(C) of C. To circumvent eigen-decomposition, we maximize instead a lower bound $\lambda _{\min }^ - \left( {{\mathbf{SC}}{{\mathbf{S}}^{ - 1}}} \right)$ of λ
min
(C)—smallest Gershgorin disc left-end of a similarity transform of C—via a variant of GDAS based on Gershgorin circle theorem (GCT). Experimental results show that our sampling method yielded smaller signal reconstruction errors at a faster speed compared to competing schemes.
更多查看译文
关键词
Graph signal processing,signal sampling,Gershgorin circle theorem
AI 理解论文
溯源树
样例
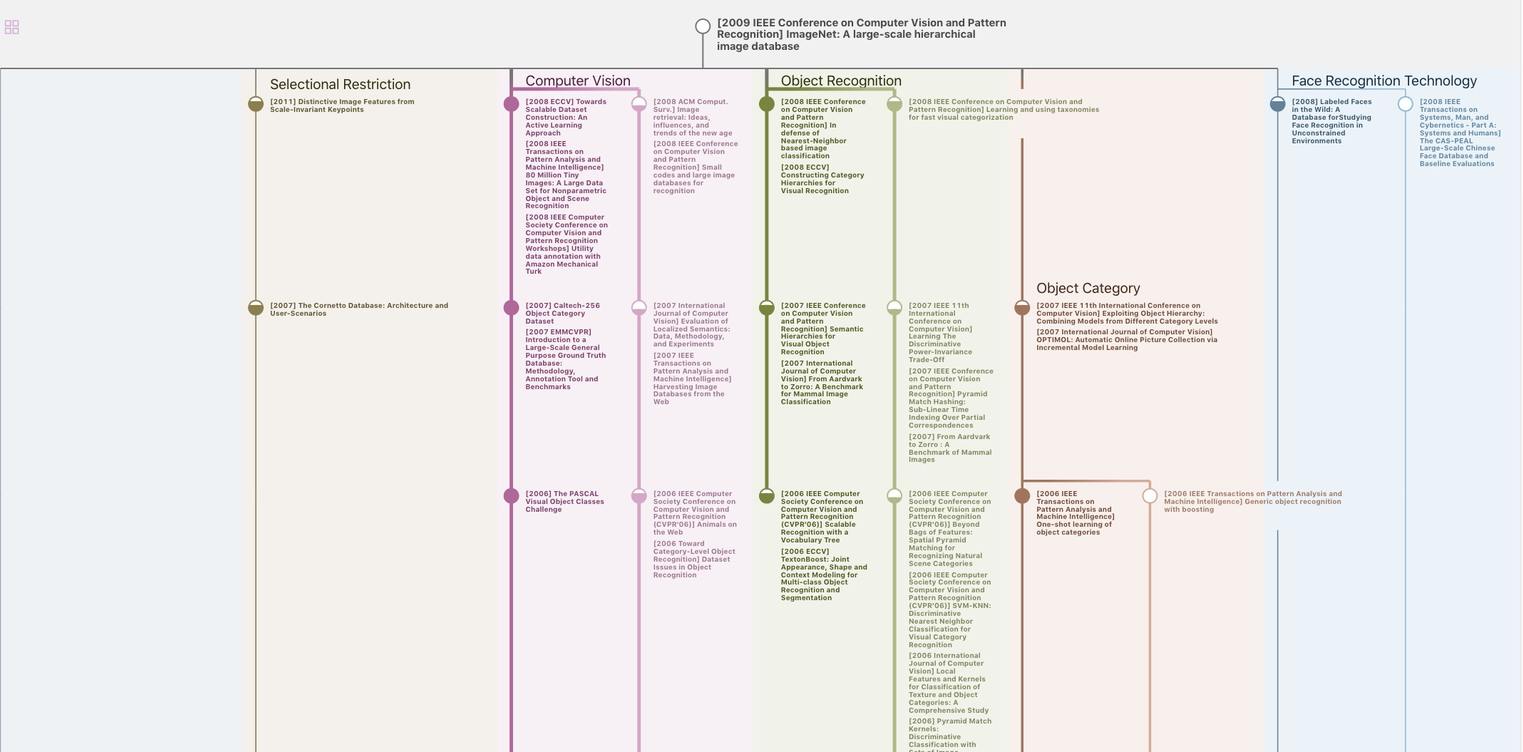
生成溯源树,研究论文发展脉络
Chat Paper
正在生成论文摘要