Fine-Grained Textual Knowledge Transfer to Improve RNN Transducers for Speech Recognition and Understanding
ICASSP 2023 - 2023 IEEE International Conference on Acoustics, Speech and Signal Processing (ICASSP)(2023)
摘要
RNN Tranducer (RNN-T) technology is very popular for building deployable models for end-to-end (E2E) automatic speech recognition (ASR) and spoken language understanding (SLU). Since these are E2E models operating on speech directly, there remains a potential to improve their performance using purely text based models like BERT, which have strong language understanding capabilities. In this paper, we propose a new training criteria for RNN-T based E2E ASR and SLU to transfer BERT’s knowledge into these systems. In the first stage of our proposed mechanism, we improve ASR performance by using a fine-grained, tokenwise knowledge transfer from BERT. In the second stage, we fine-tune the ASR model for SLU such that the above knowledge is explicitly utilized by the RNN-T model for improved performance. Our techniques improve ASR performance on the Switchboard and CallHome test sets of the NIST Hub5 2000 evaluation and on the recently released SLURP dataset on which we achieve a new state-of-the-art performance. For SLU, we show significant improvements on the SLURP slot filling task, outperforming HuBERT-base and reaching a performance close to HuBERTlarge. Compared to large transformer based speech models like HuBERT, our model is significantly more compact and uses only 300 hours of speech pretraining data.
更多查看译文
关键词
automatic speech recognition,spoken language understanding,knowledge transfer
AI 理解论文
溯源树
样例
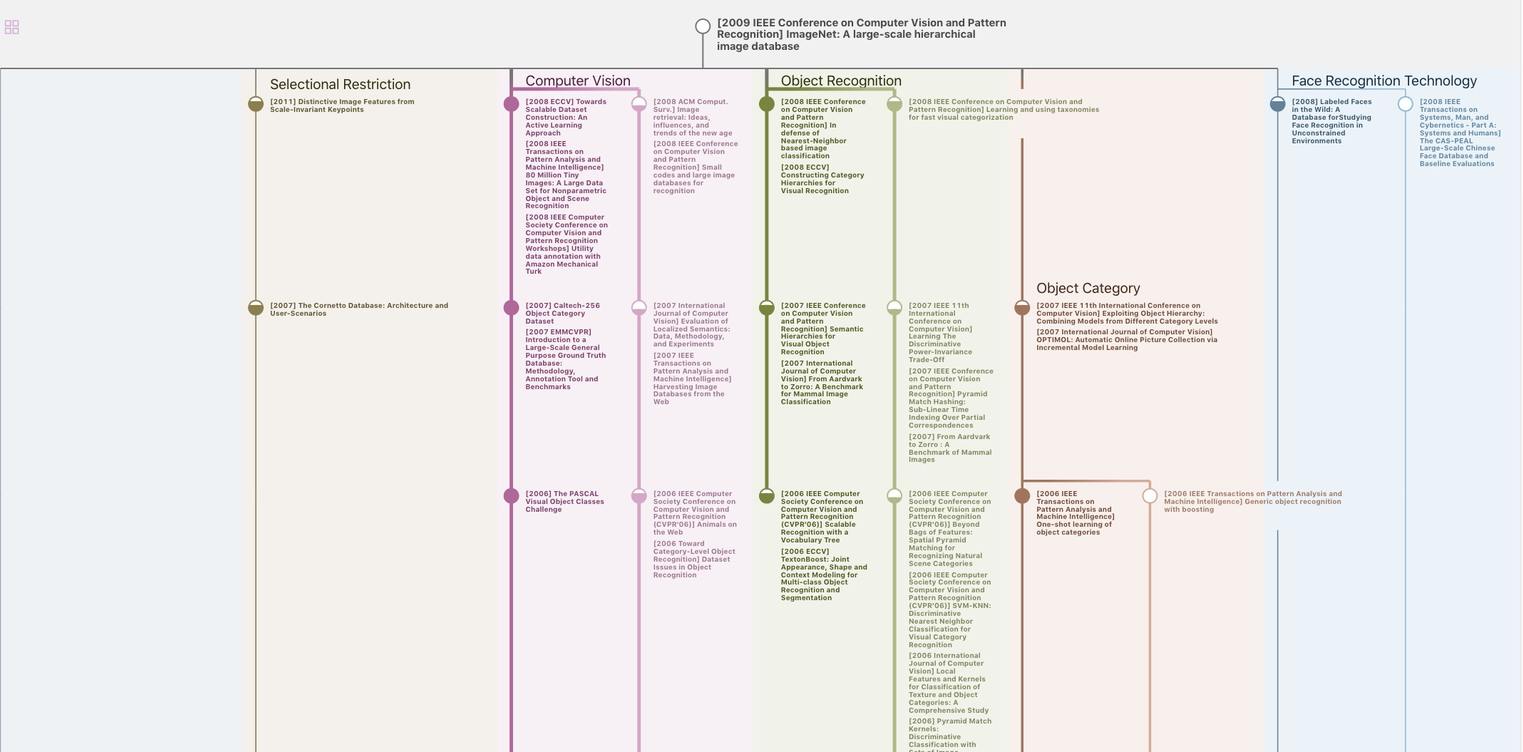
生成溯源树,研究论文发展脉络
Chat Paper
正在生成论文摘要