Boosting Signal Modulation Few-Shot Learning with Pre-Transformation
ICASSP 2023 - 2023 IEEE International Conference on Acoustics, Speech and Signal Processing (ICASSP)(2023)
摘要
The recent flourish of deep learning on various tasks is largely accredited to the rich and high-quality labeled data. Nonetheless, collecting sufficient labeled samples is not very practical for many real applications. Few-shot Learning (FSL) provides a promising solution that allows a model to learn the concept of novel classes with a few labeled samples. However, many existing FSL methods are only designed for computer vision tasks and are not suitable for radio signal recognition. This paper calls for a radically different approach to FSL: in contrast to developing a new FSL model, we should focus on transforming the radio signal to be better processed by the state-of-the-art (SOTA) FSL model. We propose Modulated Signal Pre-transformation (MSP), a parameterized radio signal transformation framework that encourages the signals having the same semantics to have similar representations. MSP currently adapts to various SOTA FSL models for signal modulation recognition and can support the mainstream deep learning backbone. Evaluation results show that MSP improves the performance gains for many SOTA FSL models while maintaining flexibility.
更多查看译文
关键词
Signal Processing,Machine Learning,Few Shot Learning
AI 理解论文
溯源树
样例
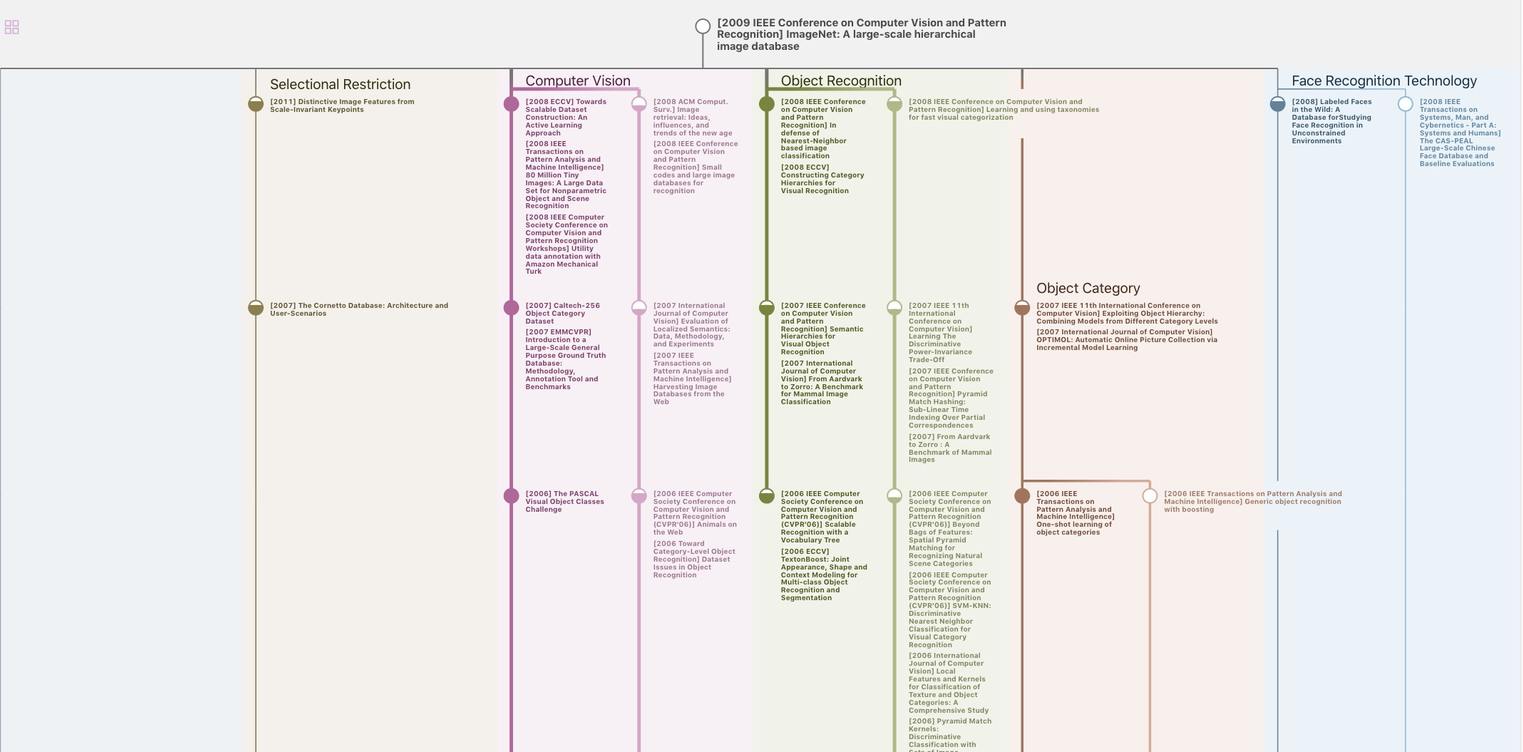
生成溯源树,研究论文发展脉络
Chat Paper
正在生成论文摘要