Stacking-Based Attention Temporal Convolutional Network for Action Segmentation
ICASSP 2023 - 2023 IEEE International Conference on Acoustics, Speech and Signal Processing (ICASSP)(2023)
摘要
Action segmentation plays an important role in video understanding, which is implemented by frame-wise action classification. Recent works on action segmentation capture long-term dependencies by increasing temporal convolution layers in Temporal Convolution Networks (TCNs). However, high layers in TCNs are more coarse access to video features, resulting in the loss of fine-grained information for frame-wise action classification. To address the above issues, we propose a novel Attention-based Temporal Convolution (ATC) block to capture fine-grained information of temporal dependencies for frame-wise action classification by self-attention mechanism. Via stacking ATC blocks, we design a Stacking-based Attention Temporal Convolutional Network (SATC) to adaptively capture long-term and short-term dependencies, according to the semantic similarity of features on different temporal receptive fields simultaneously. The experimental results demonstrate that our SATC outperforms other baselines on all three challenging datasets: GTEA, 50Salads and Breakfast.
更多查看译文
关键词
action segmentation,frame-wise action classification,temporal convolution network,deep learning
AI 理解论文
溯源树
样例
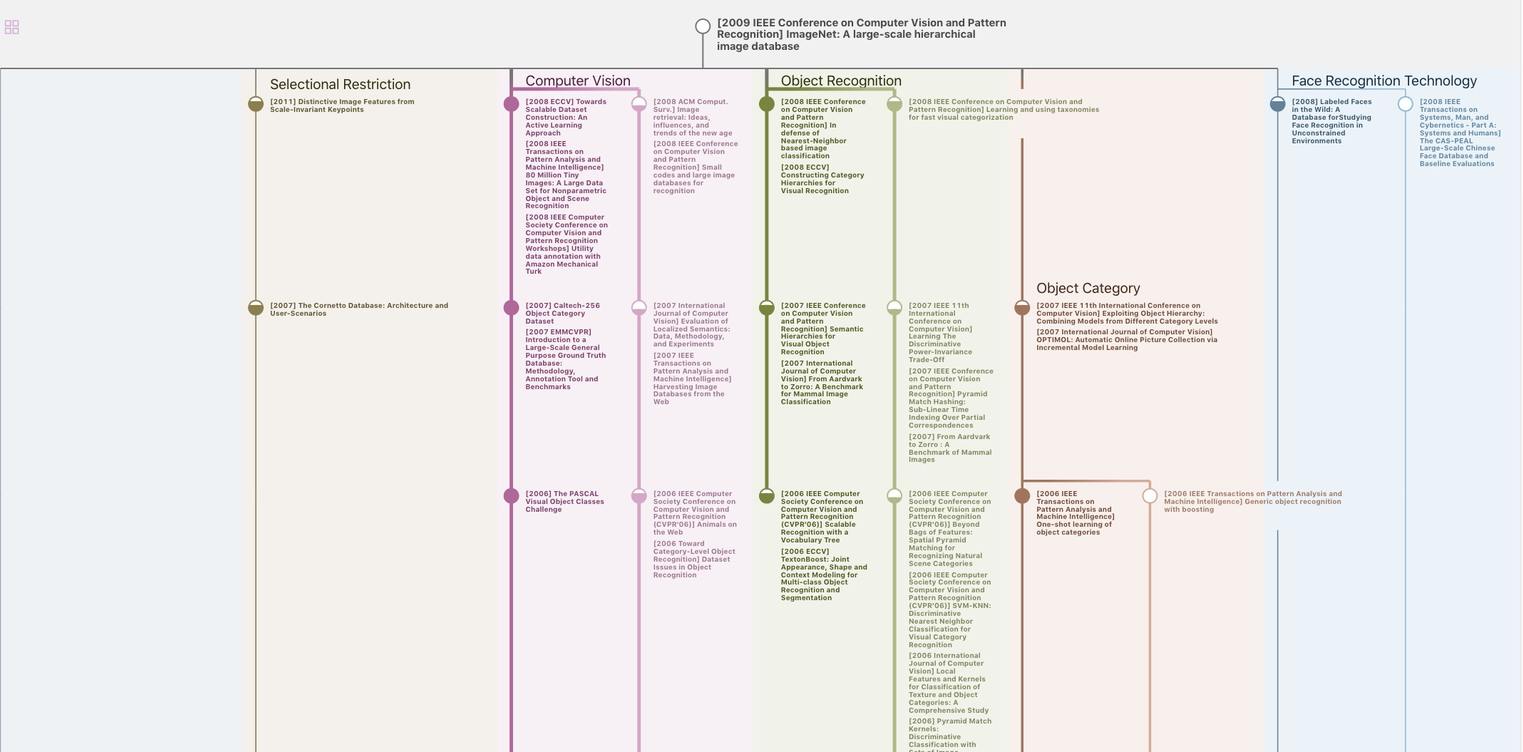
生成溯源树,研究论文发展脉络
Chat Paper
正在生成论文摘要