Neural Network Models with Integrated Training and Adaptation For Nonlinear Acoustic System Identification
ICASSP 2023 - 2023 IEEE International Conference on Acoustics, Speech and Signal Processing (ICASSP)(2023)
摘要
System identification is instrumental in various tasks of acoustics, including acoustic measurement and acoustic echo cancellation. Adaptive filtering is proven to be successful for the identification of linear parts with variable impulse responses. Neural network frameworks are recently considered for nonlinear modeling, but the desire for variable system identification on individual sequences may be incompatible with the general concept of training across batch data. This paper therefore pursues architectures mixed from trainable and non-trainable adaptive sections. By seamlessly integrating adaptive FIR filters into end-to-end neural network architectures, the desired flexibility in the network is achieved. In-network adaptivity is here implemented as a recurrent computational layer according to the normalized least mean-square (NLMS) algorithm. Altogether six architectures are explored in order to study what part of a system better relies on training or adaptation. The proposed implementations are thus verified on synthetic data with dedicated variations of speech or noise input, linear or nonlinear system components, and fixed or variant acoustic impulse responses.
更多查看译文
关键词
Acoustic system identification,nonlinear modeling,adaptive filters,neural networks
AI 理解论文
溯源树
样例
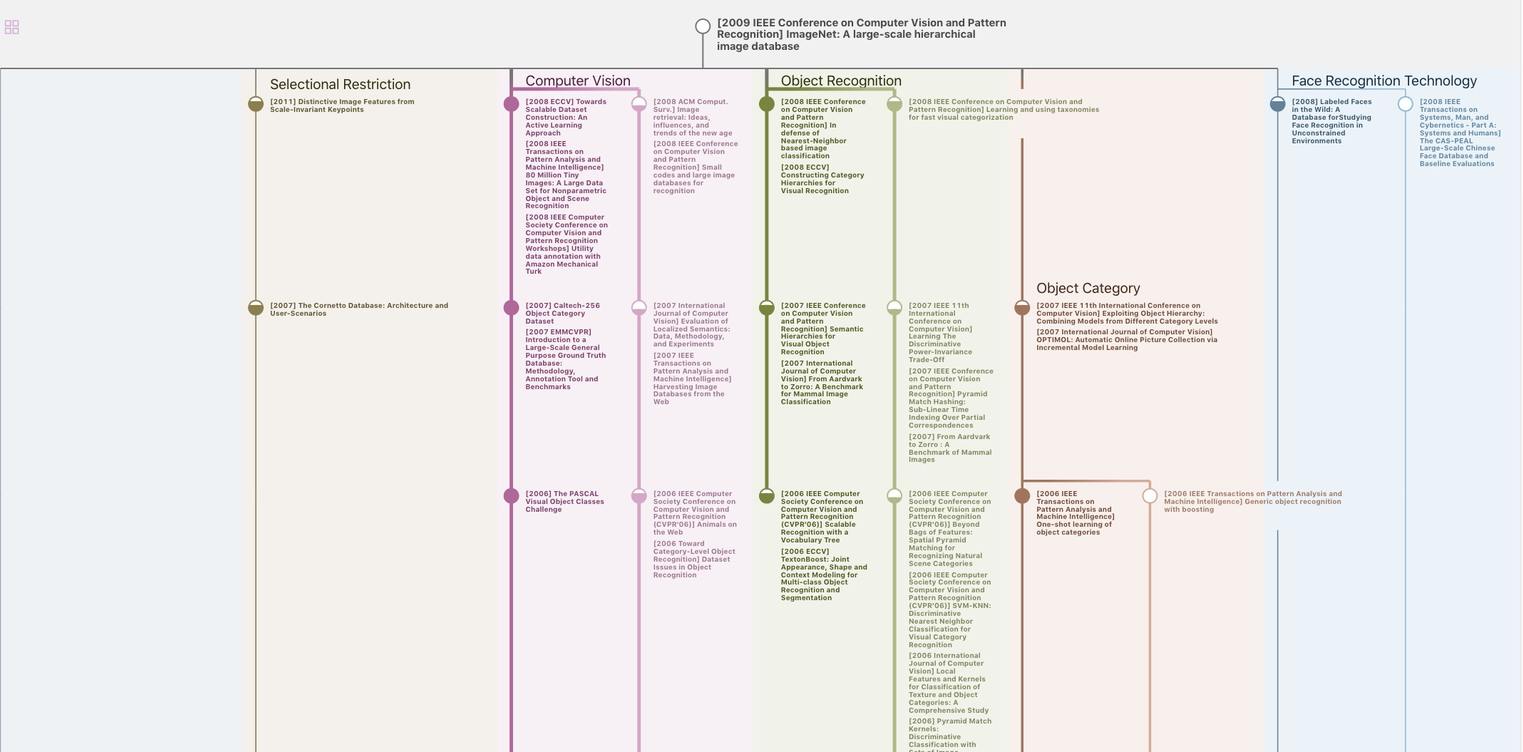
生成溯源树,研究论文发展脉络
Chat Paper
正在生成论文摘要