SS-ADMM: Stationary and Sparse Granger Causal Discovery for Cortico-Muscular Coupling
ICASSP 2023 - 2023 IEEE International Conference on Acoustics, Speech and Signal Processing (ICASSP)(2023)
摘要
Cortico-muscular communication patterns reveal important information about motor control. However, inferring significant causal relationships between motor cortex electroencephalogram (EEG) and surface electromyogram (sEMG) of concurrently active muscles is challenging since relevant processes involved in muscle control are relatively weak compared to additive noise and background activities. In this paper, a framework for identification of cortico-muscular linear time invariant communication is proposed that simultaneously estimates model order and its parameters by enforcing sparsity and stationarity conditions in a convex optimization program. The experimental results demonstrate that our proposed algorithm outperforms existing techniques for autoregressive model estimation, in terms of computational speed and model identification for causality estimation.
更多查看译文
关键词
Autoregressive model,Granger causality,stationarity,sparsity
AI 理解论文
溯源树
样例
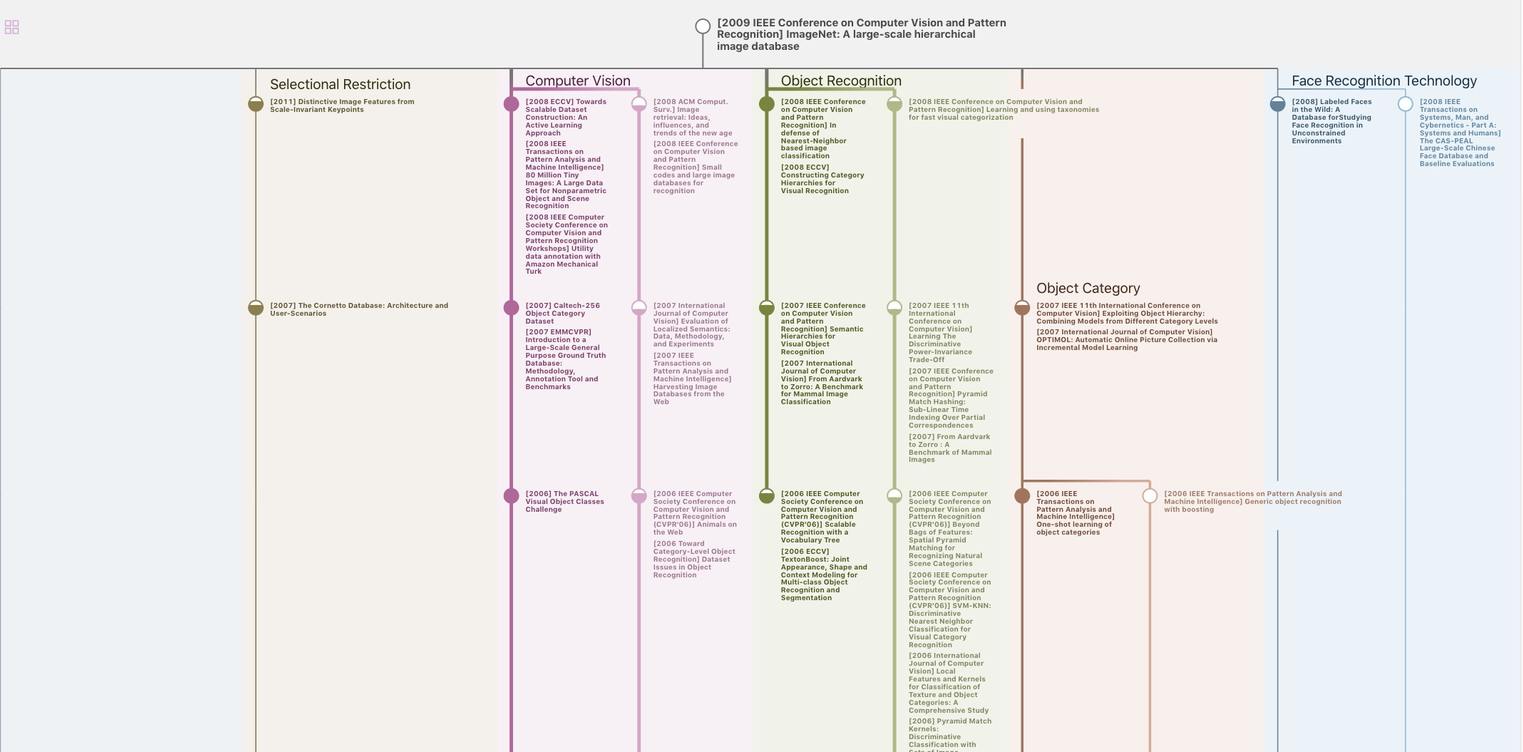
生成溯源树,研究论文发展脉络
Chat Paper
正在生成论文摘要