Tensor Decomposition Based Latent Feature Clustering for Hyperspectral Band Selection
ICASSP 2023 - 2023 IEEE International Conference on Acoustics, Speech and Signal Processing (ICASSP)(2023)
摘要
Hyperspectral band selection has been proved to be effective in reducing redundant information for hyperspectral images (HSIs). Most existing band selection methods simply consider the relationship between bands by reshaping them into vectors and destroying the spatial structure. Moreover, the converted band vectors are usually high-dimensional, making the learning processing very time-consuming. To solve these problems, we propose a tensor decomposition based latent feature clustering (TDLFC) model for band selection. We maintain the tensor structure of the HSI and use CANDECOMP/PARAFAC (CP) decomposition to learn the latent low-dimensional representation of the bands to preserve spatial and spectral information. To avoid overfitting, we introduce a regularization term for the CP decomposition model. To solve the proposed model, we present an effective optimization algorithm as solution. Finally, the k-means algorithm is applied to the latent representation to get the band clustering results for band selection. Extensive experiments on three public HSI datasets show the superiority of our proposed model over the state-of-the-art methods.
更多查看译文
关键词
Hyperspectral image,band selection,tensor decomposition,latent feature clustering
AI 理解论文
溯源树
样例
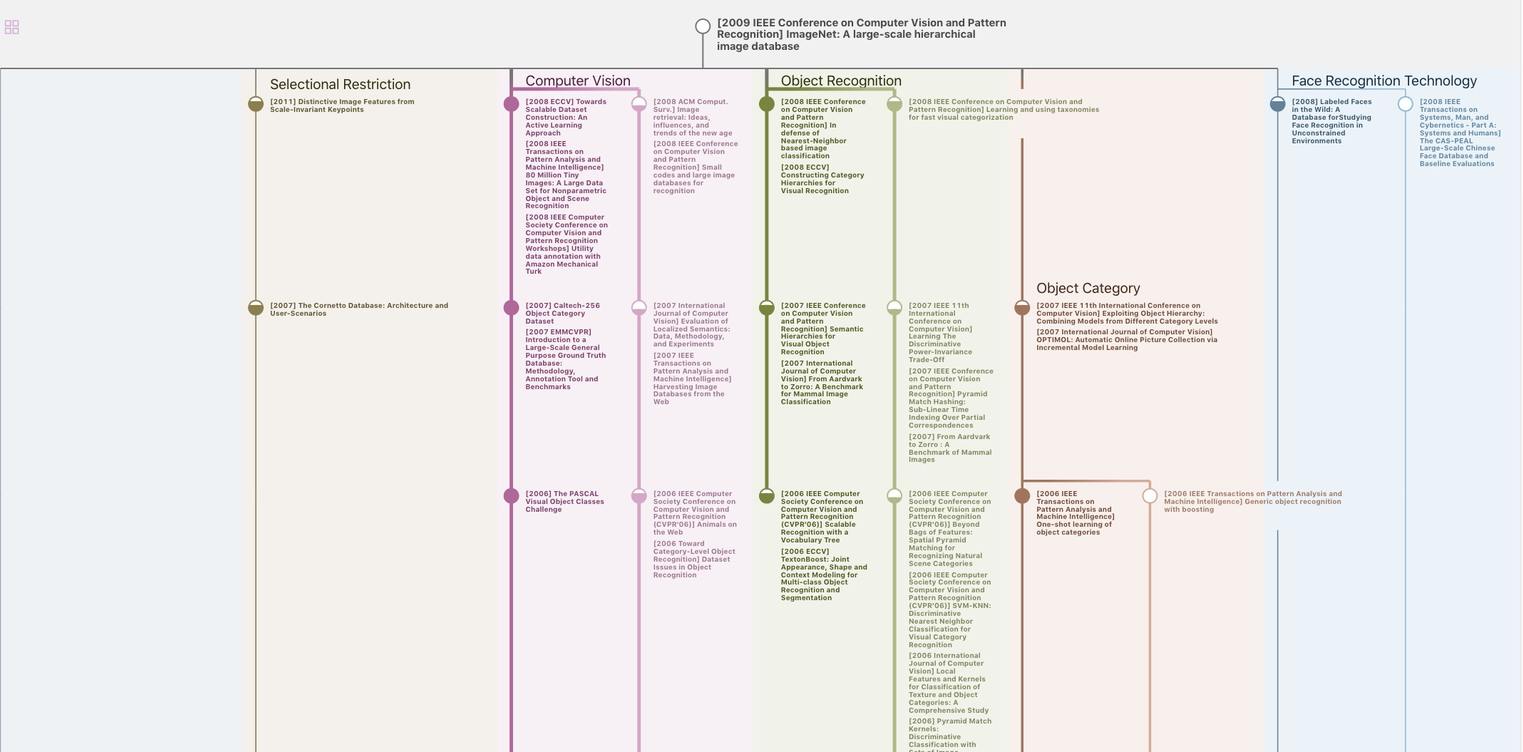
生成溯源树,研究论文发展脉络
Chat Paper
正在生成论文摘要