Low-Rank Constrained Memory Autoencoder for Hyperspectral Anomaly Detection
ICASSP 2023 - 2023 IEEE International Conference on Acoustics, Speech and Signal Processing (ICASSP)(2023)
摘要
Hyperspectral anomaly detection (HAD) aims to discern the objects deviated dramatically from their surrounding pixels. Some deep learning-based models integrating with the low-rank representation (LRR) have been proposed recently. The process of constructing dictionary in these methods is complex and the stability of the models is hard to maintain. To address these problems, in this paper, we propose a low-rank constrained memory autoencoder (LRMAE) for HAD. Specifically, we first train the memory autoencoder with a sparsity regularizer and a spectral consistency constraint in an unsupervised learning fashion and the embedded memory module is used to acquire the dictionary atoms for the construction of dictionary. The low-rank optimization process is conducted in the low-dimensional manifold space to obtain the final detection map. Substantial experiments are performed on three different datasets, and the final detection results demonstrate the superiority of the proposed model over other state-of-the-art methods.
更多查看译文
关键词
Hyperspcetral anomaly detection,remote sensing,memory autoencoder,low-rank representation
AI 理解论文
溯源树
样例
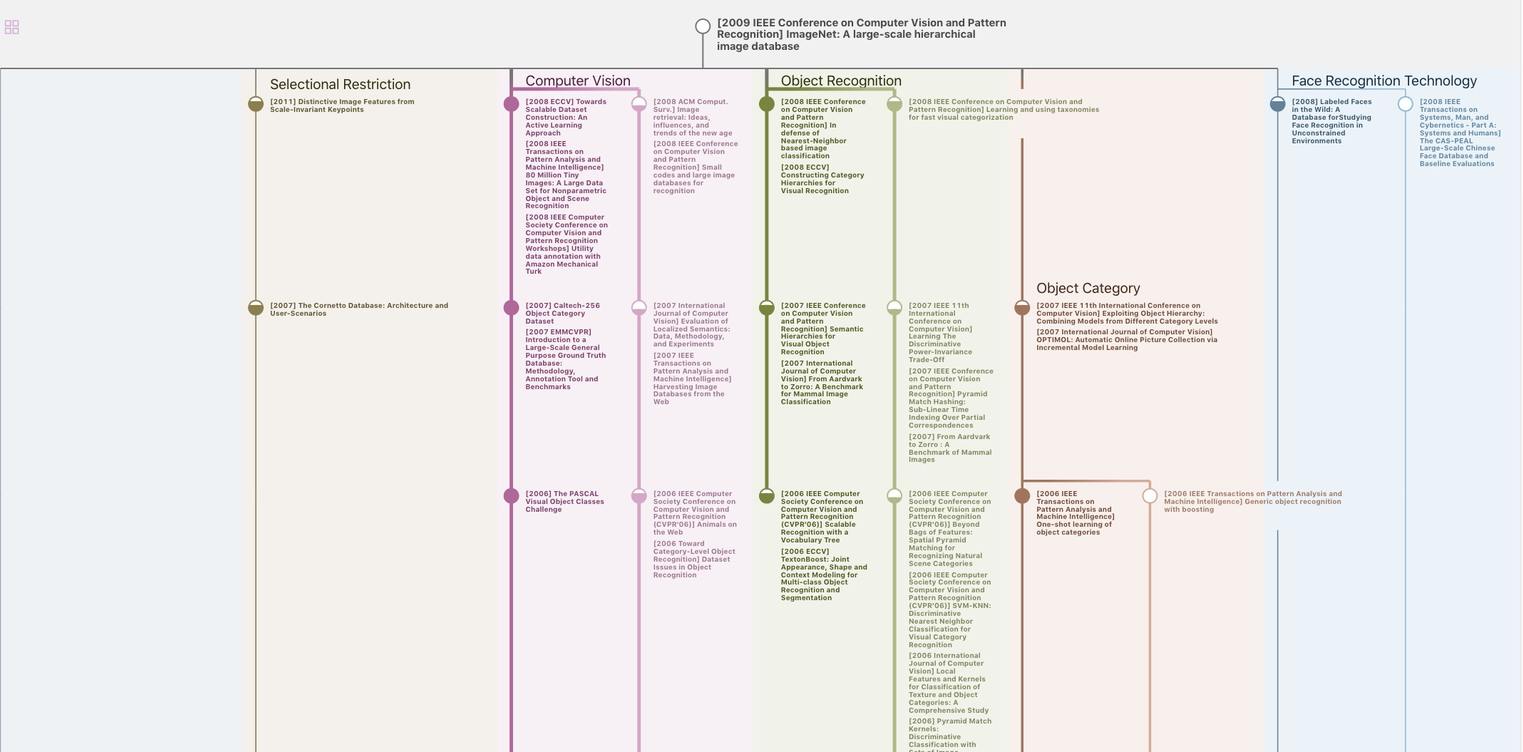
生成溯源树,研究论文发展脉络
Chat Paper
正在生成论文摘要