Balanced Mixup Loss for Long-Tailed Visual Recognition
ICASSP 2023 - 2023 IEEE International Conference on Acoustics, Speech and Signal Processing (ICASSP)(2023)
摘要
In the real world, the data collected naturally are often long-tailed, which inevitably leads to class-imbalanced prediction and performance degradation. As a simple and effective data augmentation method, Mixup has been proven to be beneficial for the tail class in recent long-tail learning studies. However, samples selected by Mixup are still imbalanced, which could exacerbate the imbalance problem. Existing work always considers adjusting the input distribution to alleviate this problem, while this may lead to over-fitting in the tail class. In this paper, we detail the theoretical analysis of the data imbalance caused by Mixup, and propose a novel Balanced Mixup (BaMix) loss function from the output perspective. By adding a balance term, we theoretically prove the BaMix loss can overcome the imbalance caused by Mixup. In the experiments, our solution achieves the state-of-the-art performance on CIFAR-LT, ImageNet-LT, and iNaturalist 2018.
更多查看译文
关键词
long-tail learning,Mixup,visual recognition
AI 理解论文
溯源树
样例
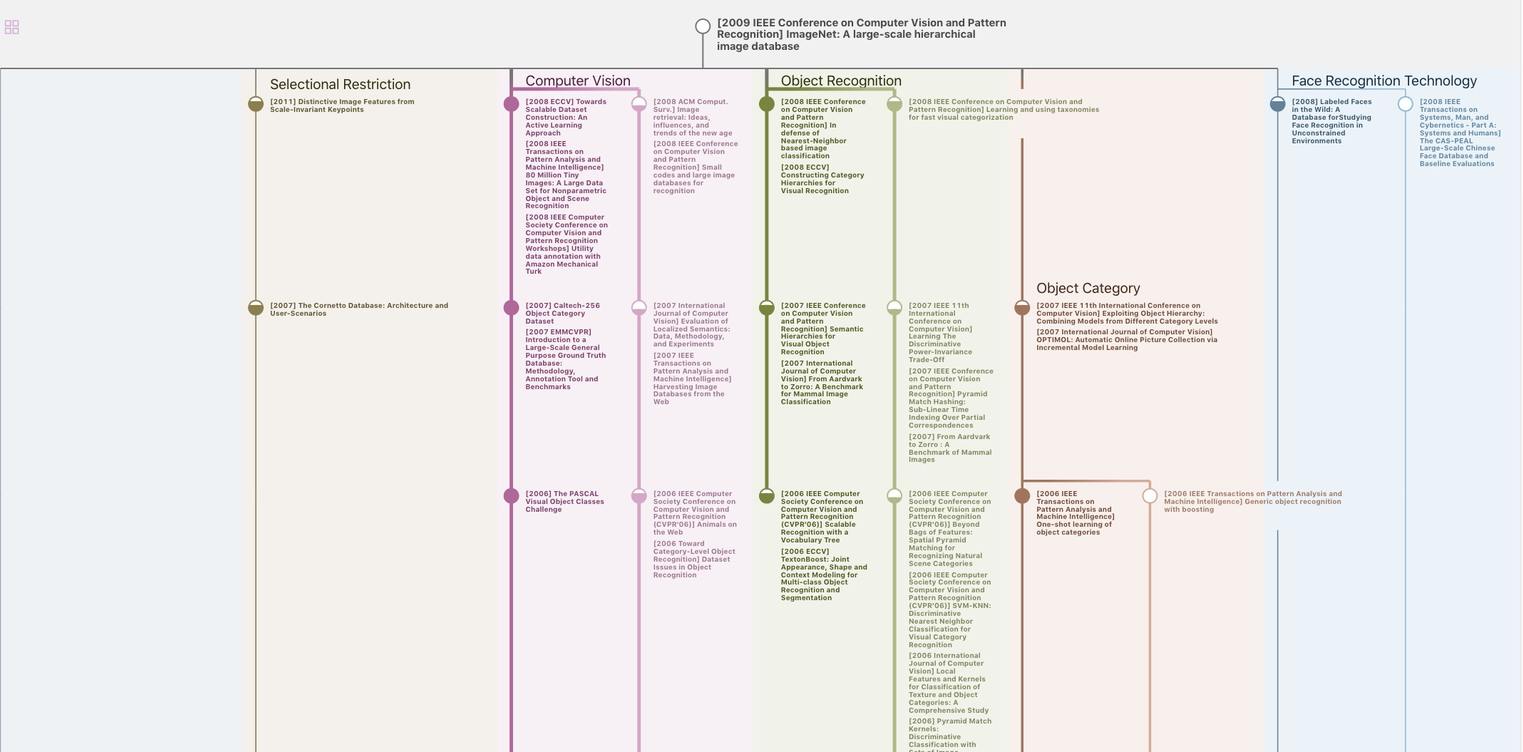
生成溯源树,研究论文发展脉络
Chat Paper
正在生成论文摘要