Deep Survival Analysis and Counterfactual Inference Using Balanced Representations
ICASSP 2023 - 2023 IEEE International Conference on Acoustics, Speech and Signal Processing (ICASSP)(2023)
摘要
Clinical decision making and diagnosis in healthcare is enabled by learning causal relationships among entities. In order to answer the question, ’Will changing the treatment regimen lead to quicker recovery of a patient?’ requires predicting counterfactuals using survival analysis. We seek to reliably estimate the average treatment effect in terms of survival probabilities of the factual outcomes as compared to survival probabilities of the unobserved counterfactual population. Due to the nature of observational data, this framework is prone to treatment selection bias and censoring bias. We address these issues and propose the novel SurvCI framework which consists of a counterfactual inference (CI) algorithm for data with time-to-event outcomes. We investigate the performance of the proposed framework on synthetic and semi-synthetic data and employ survival and causal metrics to empirically confirm that our method outperforms baselines.
更多查看译文
关键词
Counterfactual Inference,Survival Analysis,Average Treatment Effect,Deep Learning
AI 理解论文
溯源树
样例
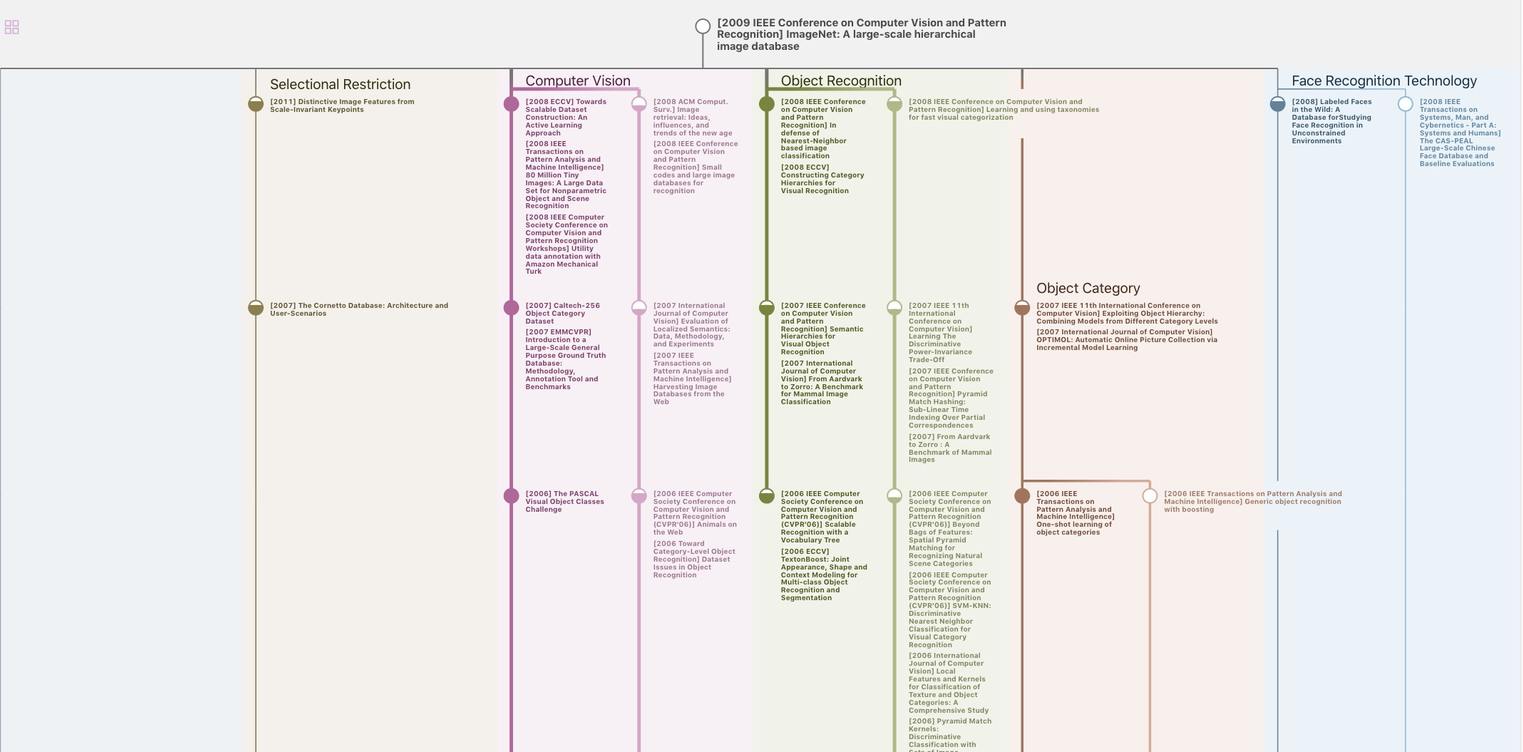
生成溯源树,研究论文发展脉络
Chat Paper
正在生成论文摘要