Ultimate Negative Sampling for Contrastive Learning
ICASSP 2023 - 2023 IEEE International Conference on Acoustics, Speech and Signal Processing (ICASSP)(2023)
摘要
Unsupervised learning has received more attention due to the superior performance of contrastive learning methods. Most contrastive methods use data augmentation techniques to construct positive and negative pairs. The augmented view of the same sample is regarded as a positive sample, while the rest are negative samples. This negative sampling strategy has strong randomness and ignores samples that are semantically similar to anchors, namely sampling bias. This problem has been addressed by weighting the similarity of negative samples. In this paper, we propose a novel ultimate negative sampling for contrastive learning. Unlike random sampling, we set a more extreme negative sample selection mechanism based on the ideal representation of the sample. Furthermore, we constrain the consistency between samples across the space. Experiment results demonstrate the proposed method’s superiority on multiple benchmark datasets.
更多查看译文
关键词
Representation Learning,Contrastive Learning,Negative Sampling
AI 理解论文
溯源树
样例
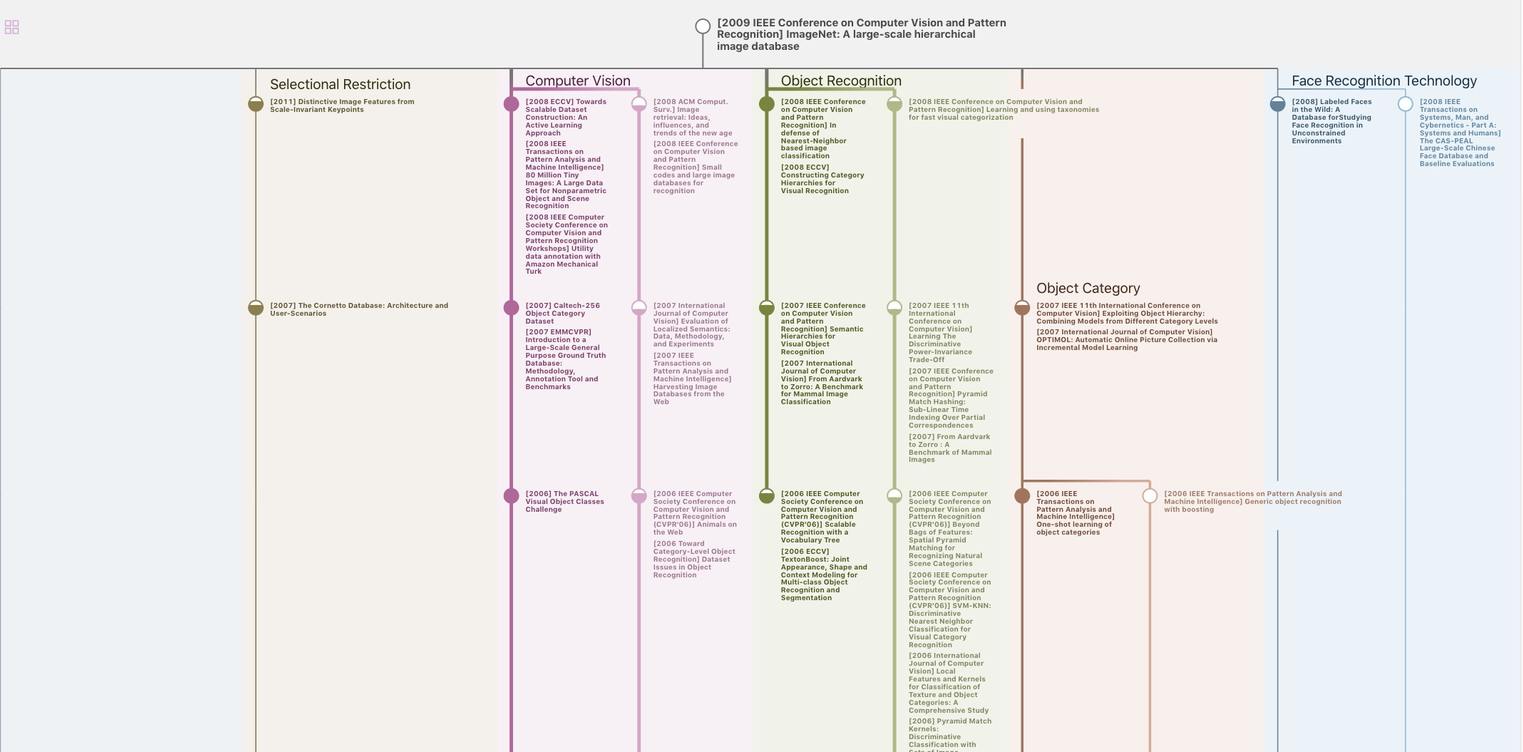
生成溯源树,研究论文发展脉络
Chat Paper
正在生成论文摘要