Towards the Interpretation of Multi-label Image Classification using Transformers and Fuzzy Cognitive Maps
2023 IEEE INTERNATIONAL CONFERENCE ON FUZZY SYSTEMS, FUZZ(2023)
摘要
Multi-label image classification is a challenging task in the field of computer vision. Recently, many deep learning approaches have been proposed to deal with this task; however, despite their effectiveness, they lack interpretability, in the sense that they are unable to explain or justify their outcomes. To address this limitation, in this paper, we propose a novel framework for interpretable multi-label image classification, based on Fuzzy Cognitive Maps (FCMs) and Transformers. The introduced framework distinguishes the most representative image semantics and analyzes them using cause-and-effect relationships. It provides understandable classification interpretations, using linguistic terms, in a way compatible to human perception, while being simple and easy to implement. The main contributions of this paper include: a) a novel FCM model enabling the interpretation of the outcomes of transformers; b) the first FCM approach developed to perform multi-label image classification; c) a mechanism to automatically define the weights of the FCM graph and the fuzzy sets needed, thus limiting human intervention in the definition of the FCM structure. Experiments on publicly available datasets demonstrates the effectiveness of the proposed framework, with competitive classification results in comparison to other transformer-based multi-label classifiers, while offering the advantage of interpretability.
更多查看译文
关键词
fuzzy cognitive maps,fuzzy logic,multi-label image classification,transformers,interpretability
AI 理解论文
溯源树
样例
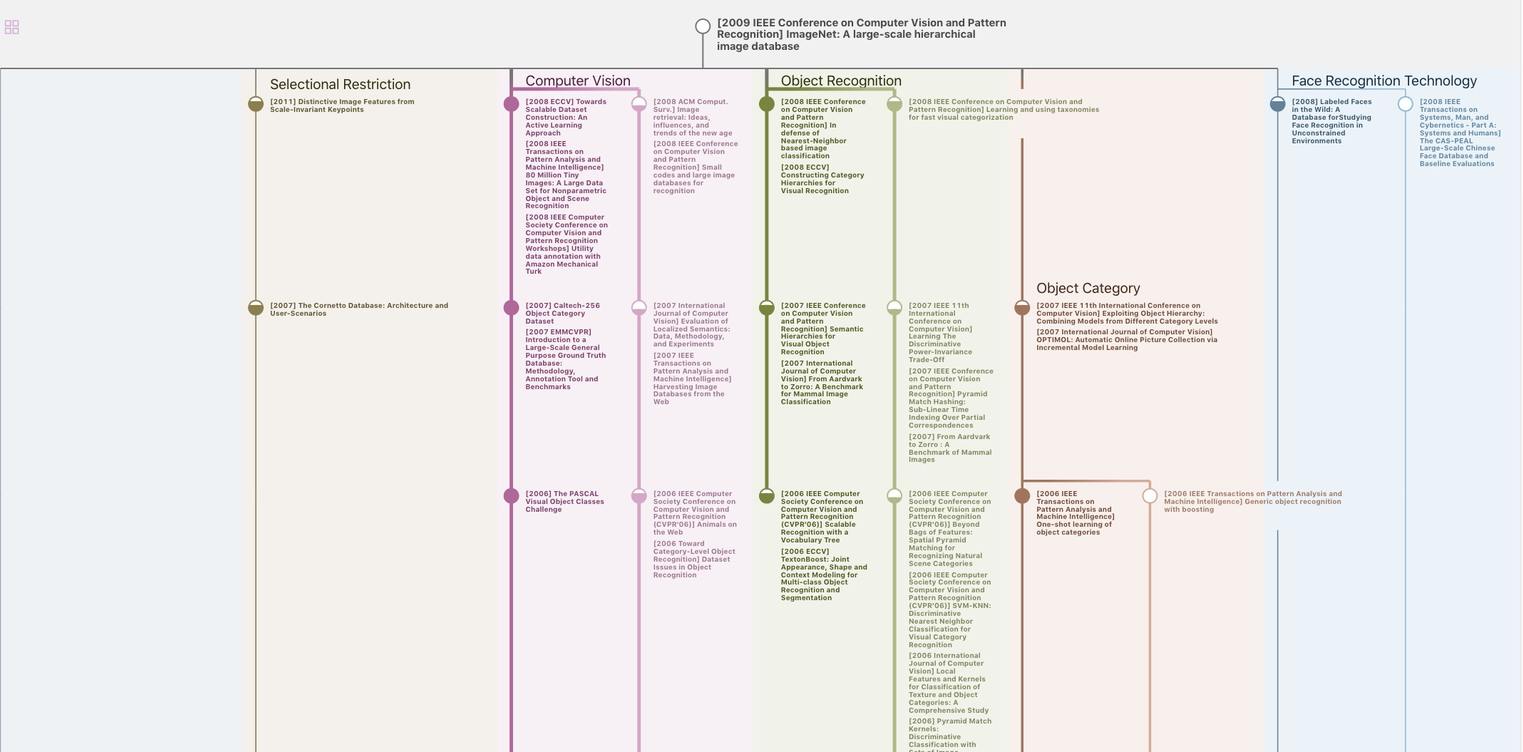
生成溯源树,研究论文发展脉络
Chat Paper
正在生成论文摘要