An Initial Step Towards Stable Explanations for Multivariate Time Series Classifiers with LIME
2023 IEEE INTERNATIONAL CONFERENCE ON FUZZY SYSTEMS, FUZZ(2023)
摘要
LIME, or 'Local Interpretability Model-agnostic Explanations' is a well-known post-hoc explanation technique for the interpretation of black-box models. While very useful, recent studies show that LIME suffers from stability problems: explanations provided for the same process can be different, making it difficult to trust their reliability. This paper investigates the stability of LIME when explaining multivariate time series classification problems. We demonstrate that due to the temporal dependency in time series data, the traditional artificial neighbour generation methods used in LIME have a higher risk of creating out-of-distribution inputs. We disucss how this behavior is one of the reasons resulting in unstable explanations. In addition, LIME weights neighbours based on user-defined hyperparameters which are problem-dependent and hard to tune, and we show how unsuitable hyperparameters can contribute to the generation of unstable explanations. As a preliminary step towards addressing these issues, we propose to employ a generative approach with an adaptive weighting method in the LIME framework. Specifically, we adopt a generative model based on variational autoencoder to create within-distribution neighbours, reducing the out-of-distribution problem, while the adaptive weight method eliminates the need for user-defined hyperparameters. Experiments on real-world datasets demonstrate the effectiveness of the proposed method in providing more stable explanations using the LIME framework.
更多查看译文
关键词
Multivariate Time Series Classification,Explainable Artificial Intelligence,LIME,Stability,Trustworthiness
AI 理解论文
溯源树
样例
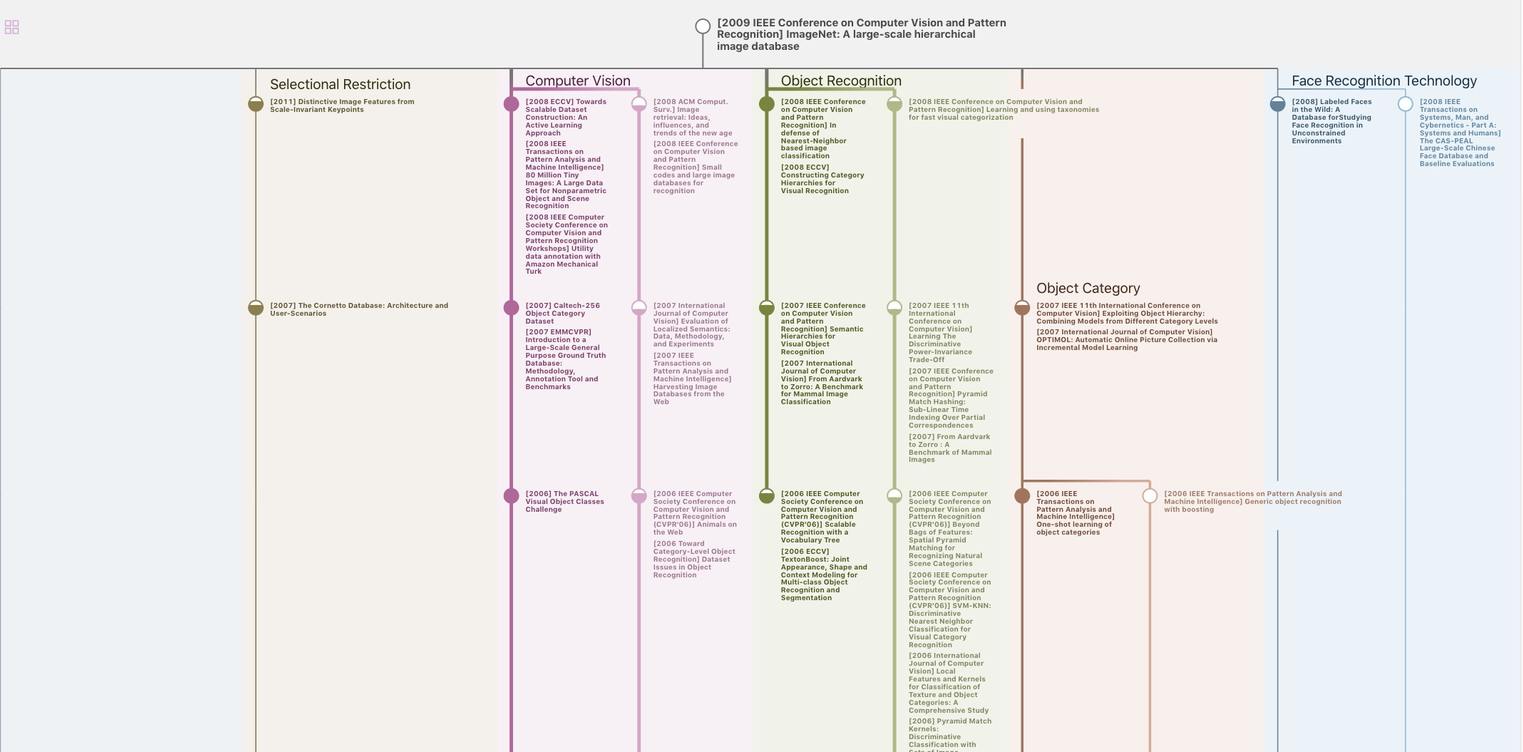
生成溯源树,研究论文发展脉络
Chat Paper
正在生成论文摘要