Towards Reliable Uncertainty Quantification and High Precision with General Type-2 Fuzzy Systems
2023 IEEE INTERNATIONAL CONFERENCE ON FUZZY SYSTEMS, FUZZ(2023)
摘要
Deep learning models have been successfully developed to solve complex problems with the main focus on high precision. Yet, accurately assessing uncertainty and prediction is essential for making informed decisions, especially in high-risk tasks. In this paper, we present a step towards learning reliable uncertainty quantification and high precision performance via alpha-plane based General Type-2 Fuzzy Logic Systems (GT2-FLSs). To balance between accuracy and uncertainty quantification, we propose a novel composite loss function consisting of an accuracyfocused and uncertainty-focused loss term that exploits the parameters of the Secondary Membership Functions (SMFs). For the uncertainty-focused term, we use only the type-reduced set of alpha(0) = 0 plane of the GT2-FLS, i.e. the size of the SMFs, which does not contribute to the output calculation directly. In the accuracyfocused part, we present two options for the error terms. One uses the aggregated output while the other uses only the output alpha(k) = 1 plane of the GT2-FLS. In both terms, we make the SMF shape parameters responsible for learning pointwise prediction. We present statistical comparisons and demonstrate that the learned GT2-FLSs generate reliable prediction intervals while also resulting in high-precision performance. The results show the potential of the proposed approach for GT2-FLS as a promising solution for making reliable predictions in real-world applications.
更多查看译文
关键词
general type-2 fuzzy logic systems,accuracy,uncertainty,prediction interval,deep learning
AI 理解论文
溯源树
样例
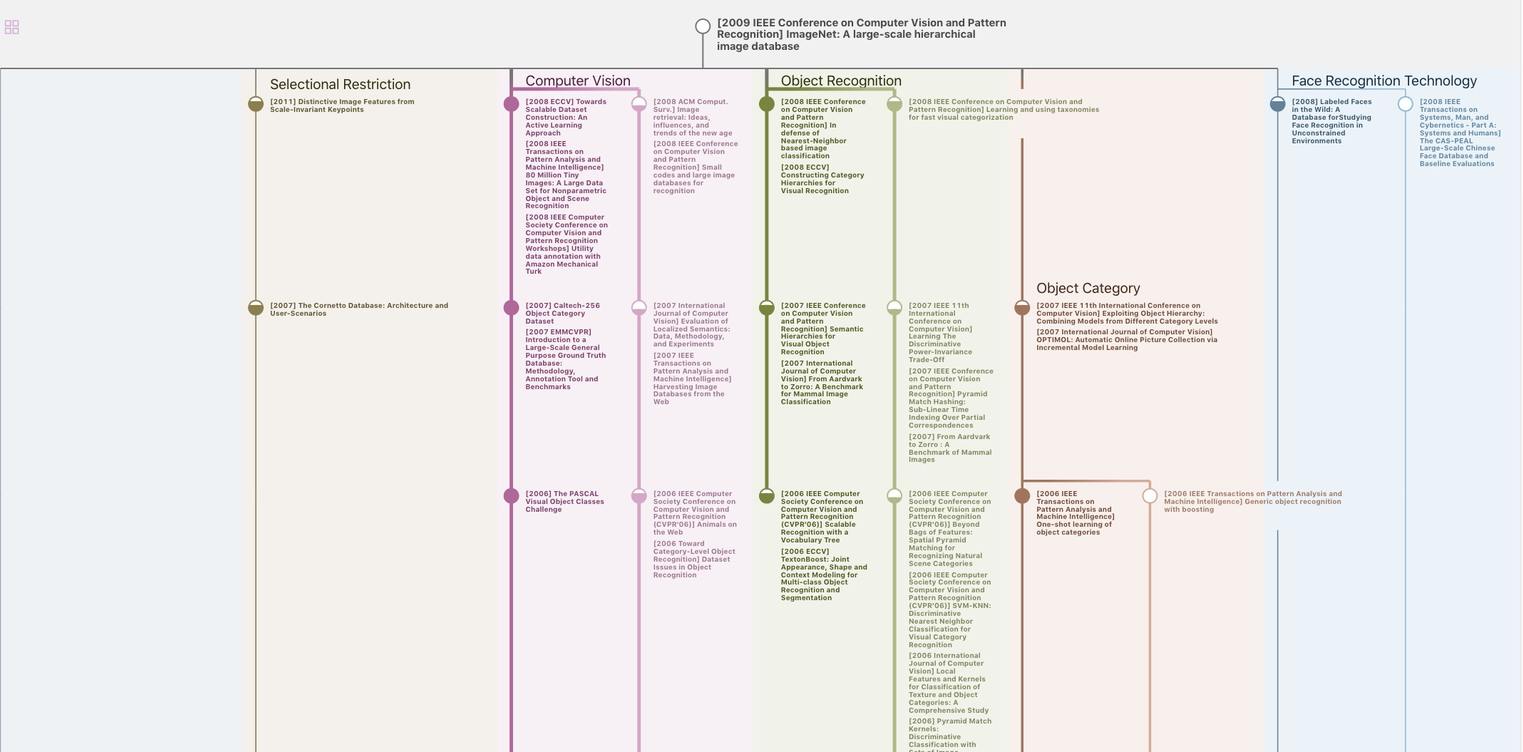
生成溯源树,研究论文发展脉络
Chat Paper
正在生成论文摘要