An Encoder-Sequencer-Decoder Network for Lane Detection to Facilitate Autonomous Driving
2023 23rd International Conference on Control, Automation and Systems (ICCAS)(2023)
摘要
Lane detection in all weather conditions is a pressing necessity for autonomous driving. Accurate lane detection ensures the safe operation of autonomous vehicles, enabling advanced driver assistance systems to effectively track and maintain the vehicle within the lanes. Traditional lane detection techniques heavily rely on a single image frame captured by the camera, posing limitations. Moreover, these conventional methods demand a constant stream of pristine images for uninterrupted lane detection, resulting in degraded performance when faced with challenges such as low brightness, shadows, occlusions, and deteriorating environmental conditions. Recognizing that continuous sequence patterns on the road represent lanes, our approach leverages a sequential model to process multiple images for lane detection. In this study, we propose a deep neural network model to extract crucial lane information from a sequence of images. Our model adopts a convolutional neural network in an encoder/decoder architecture and incorporates an extended short-term memory model for sequential feature extraction. We evaluate the performance of our proposed model using the TuSimple and CuLane datasets, showcasing its superiority across various lane detection scenarios. Comparative analysis with state-of-the-art lane detection methods further substantiates our model's effectiveness.
更多查看译文
关键词
Convolutional LSTM,Encoder and Decoder Network,TuSimple,Autonomous Driving and Robotics
AI 理解论文
溯源树
样例
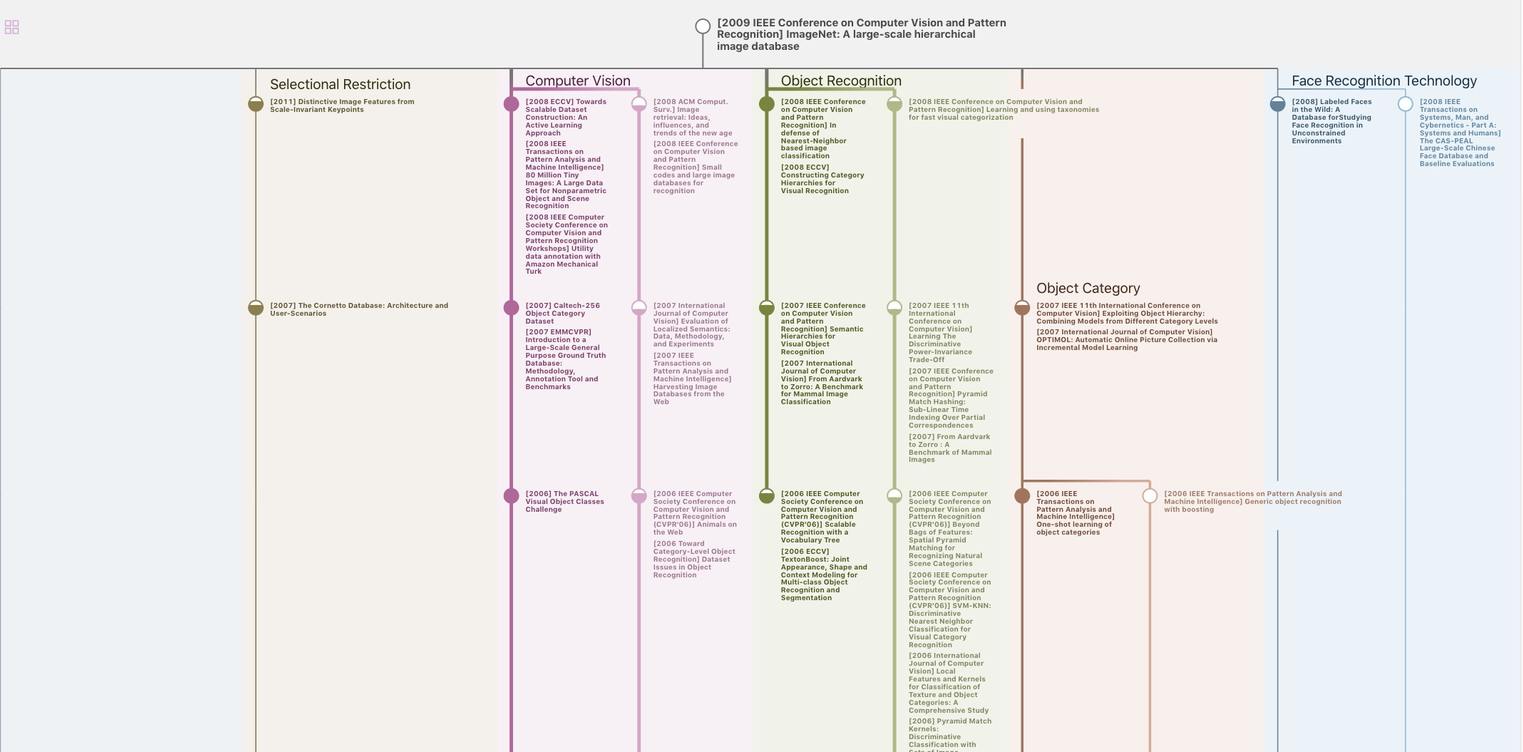
生成溯源树,研究论文发展脉络
Chat Paper
正在生成论文摘要