Semantic Data Augmentation for Deep Learning Testing using Generative AI
2023 38TH IEEE/ACM INTERNATIONAL CONFERENCE ON AUTOMATED SOFTWARE ENGINEERING, ASE(2023)
摘要
The performance of state-of-the-art Deep Learning models heavily depends on the availability of well-curated training and testing datasets that sufficiently capture the operational domain. Data augmentation is an effective technique in alleviating data scarcity, reducing the time-consuming and expensive data collection and labelling processes. Despite their potential, existing data augmentation techniques primarily focus on simple geometric and colour space transformations, like noise, flipping and resizing, producing datasets with limited diversity. When the augmented dataset is used for testing the Deep Learning models, the derived results are typically uninformative about the robustness of the models. We address this gap by introducing GENFUZZER, a novel coverage-guided data augmentation fuzzing technique for Deep Learning models underpinned by generative AI. We demonstrate our approach using widely-adopted datasets and models employed for image classification, illustrating its effectiveness in generating informative datasets leading up to a 26% increase in widely-used coverage criteria.
更多查看译文
关键词
Generative AI,Deep Learning Testing,Coverage Guided Fuzzing,Data Augmentation,Safe AI
AI 理解论文
溯源树
样例
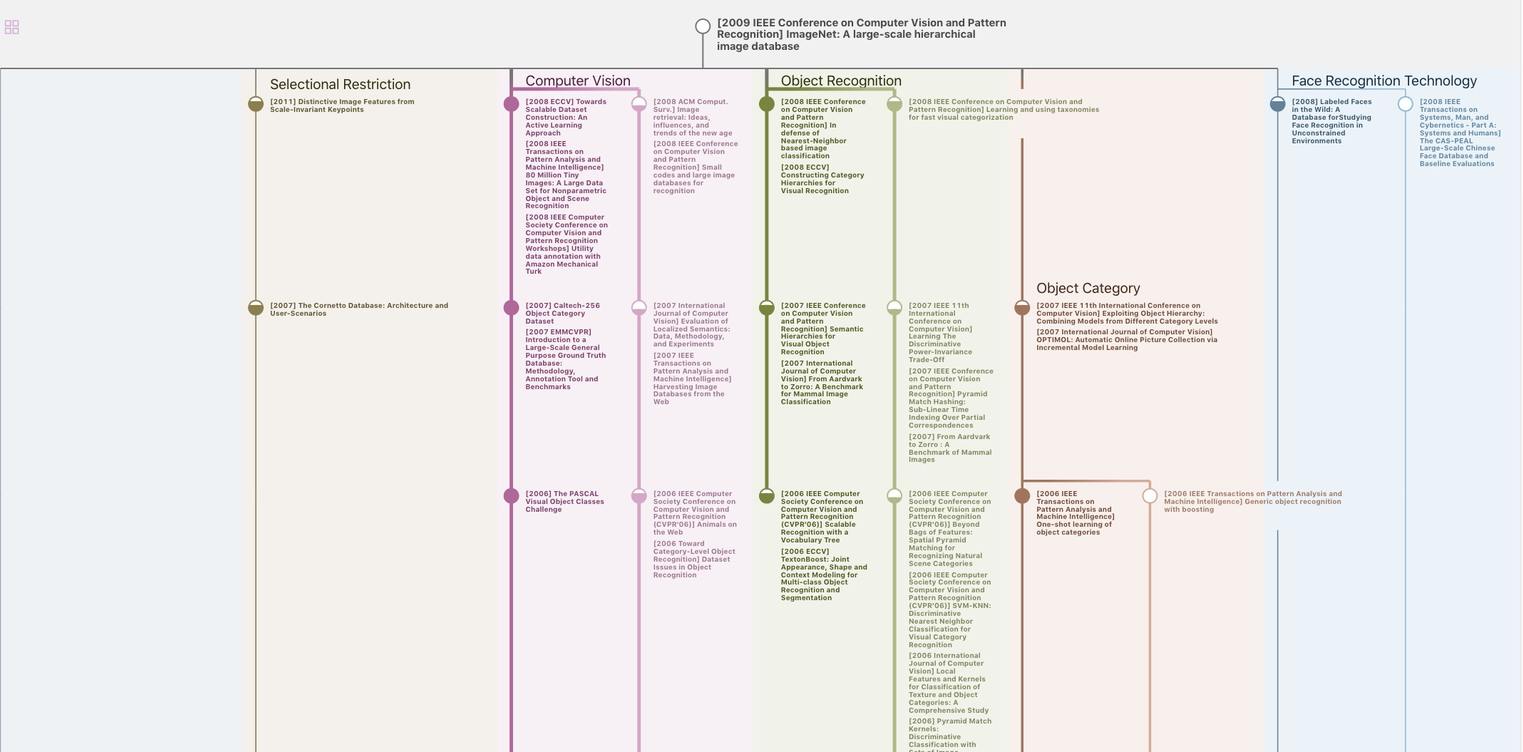
生成溯源树,研究论文发展脉络
Chat Paper
正在生成论文摘要