Application of Wafer Defect Pattern Classification Model in the Semiconductor Industry
2023 ASIA PACIFIC SIGNAL AND INFORMATION PROCESSING ASSOCIATION ANNUAL SUMMIT AND CONFERENCE, APSIPA ASC(2023)
摘要
Deep learning (DL) methods are widely employed in the semiconductor manufacturing process to enhance pattern recognition and classification accuracy, specifically for addressing defect patterns. However, the classification performance of the current models is hindered by the imbalanced distribution of defect data within the test dataset. To tackle this issue, this study presents a feature extraction approach utilizing data transformation, and ensemble learning techniques aiming to enhance the model's classification performance. The primary objective of this study is to mitigate selection and imbalance problems in the dataset through random sampling and assigning distinct weights to individual classifiers. The results demonstrate that the proposed method achieves an impressive accuracy rate of 95.09%, thus substantiating its efficacy in improving the robustness of both the classification model and wafer classification.
更多查看译文
AI 理解论文
溯源树
样例
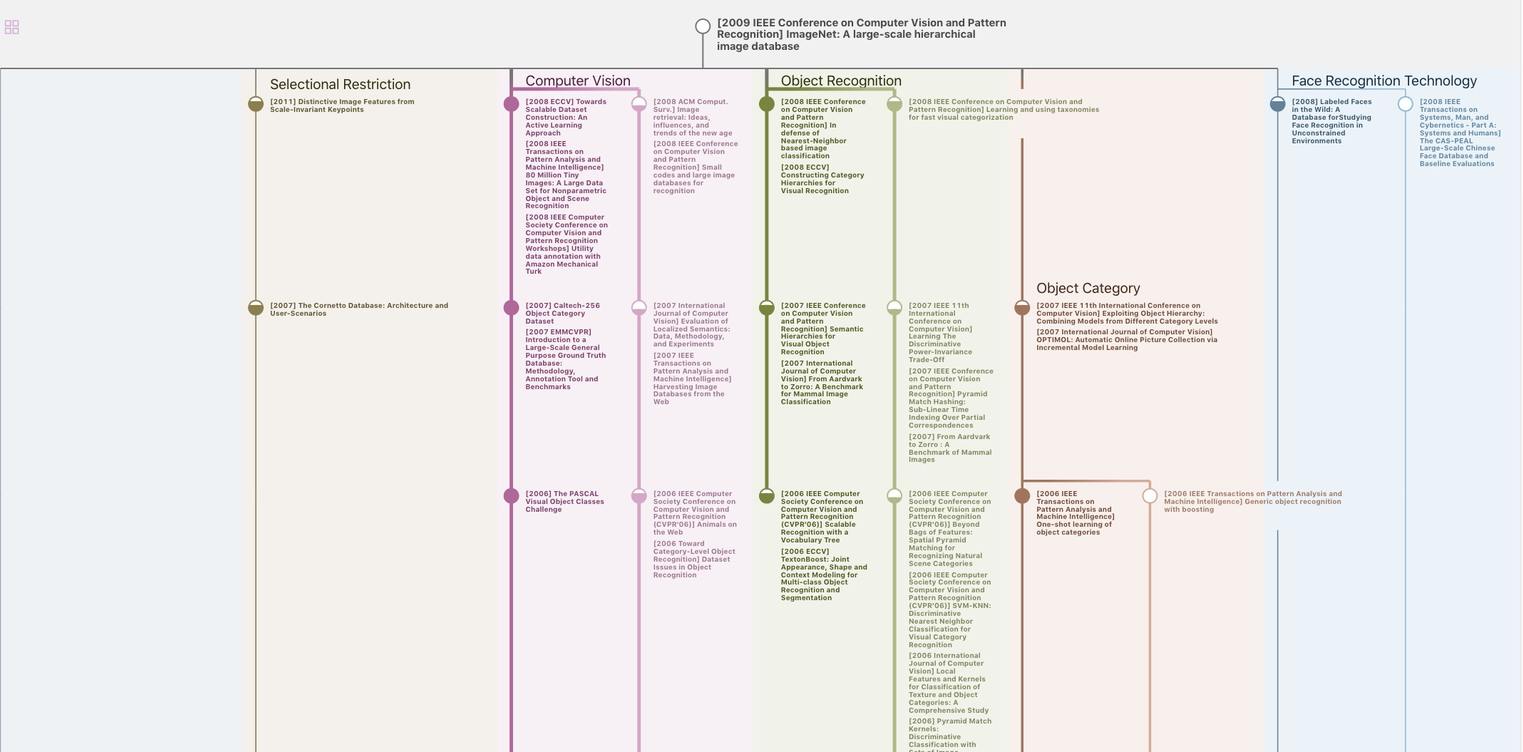
生成溯源树,研究论文发展脉络
Chat Paper
正在生成论文摘要