Improving Sound Event Localization and Detection with Class-Dependent Sound Separation for Real-World Scenarios
2023 ASIA PACIFIC SIGNAL AND INFORMATION PROCESSING ASSOCIATION ANNUAL SUMMIT AND CONFERENCE, APSIPA ASC(2023)
摘要
In this study, we propose a novel approach to sound event localization and detection (SELD) by using sound separation (SS) models to tackle key challenges of a high percentage of overlapped segments between sound events and imbalanced distributions of sound event classes in real-world scenarios. Specifically, we introduce class-dependent SS models to deal with overlapping mixtures and extract features from the SS model as prompts for SELD of a specific event class. The proposed SS-SELD method enhances the overall performance of the SELD system, resulting in improved accuracy and robustness in real-world scenarios. In contrast to many other classification methods that can be affected by the interference events, the proposed class-dependent SS framework enhances the overall performance of the SELD system, resulting in improved accuracies and robustness in real-world scenarios. When evaluated on the Sony-TAu Realistic Spatial Soundscapes 2023 (STARSS23) dataset, we demonstrate significant improvements in both sound event detection (SED) and direction-of-arrival (DOA) estimation. Our findings suggest that sound separation is a promising strategy to enhance the performance of SELD systems, particularly in scenarios with high overlaps between sound events and imbalanced distributions of event classes. In addition, our proposed framework had contributed building to our champion systems submitted to the Challenge of DCASE 2023 Task 3.
更多查看译文
AI 理解论文
溯源树
样例
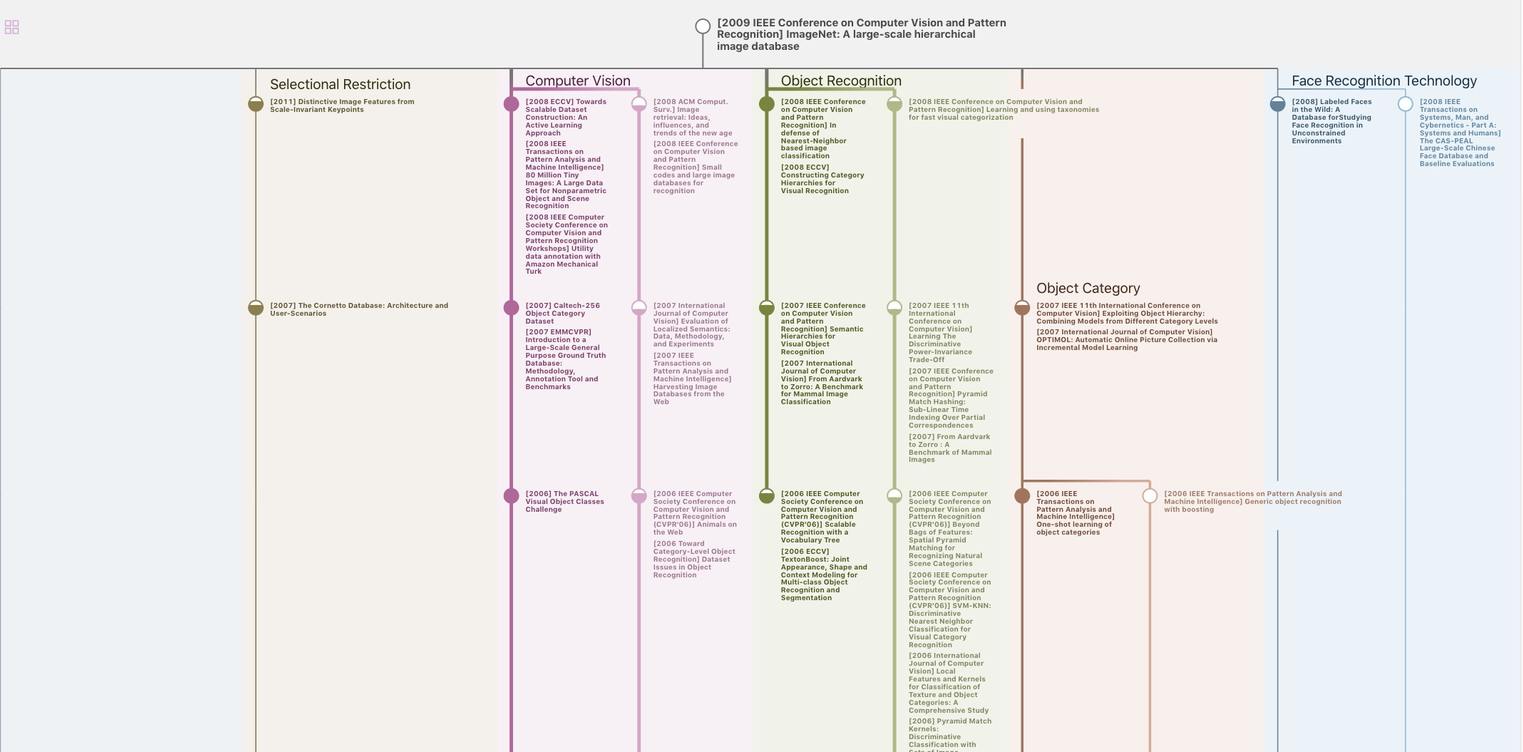
生成溯源树,研究论文发展脉络
Chat Paper
正在生成论文摘要