A Deep Reinforcement Learning Approach for Federated Learning Optimization with UAV Trajectory Planning
2023 IEEE 34TH ANNUAL INTERNATIONAL SYMPOSIUM ON PERSONAL, INDOOR AND MOBILE RADIO COMMUNICATIONS, PIMRC(2023)
摘要
Federated learning (FL) provides an efficient distributed learning framework for computing-constrained Unmanned aerial vehicles (UAVs) swarms. However, due to the dynamic channel condition and limited resources in UAV swarm, the efficiency of FL requires to be further improved. In this paper, by leveraging the motion characteristics of UAVs, we propose an energy-efficient FL framework based on trajectory planning to train machine learning (ML) models. In the proposed framework, a two-level UAV swarm is established, consisting of a high-level UAV (H-UAV) and a group of low-level UAVs (LUAVs). Considering the limited energy resources of the UAVs, our primary objective is to minimize the flight energy consumption of the H-UAV as it is much higher than the communication and computation energy consumption. To achieve this goal, we formulate the optimization problem jointly considering the adjustment of FL training parameters and the trajectory planning of H-UAV. Then, we reformulate the problem as a Markov decision process (MDP) and employ soft actor-critic (SAC) and deep deterministic policy gradient (DDPG) algorithms to tackle it. Simulation results show that the proposed approach and the proposed algorithms have good performance.
更多查看译文
关键词
UAV swarm,federated learning,trajectory optimization,energy efficiency
AI 理解论文
溯源树
样例
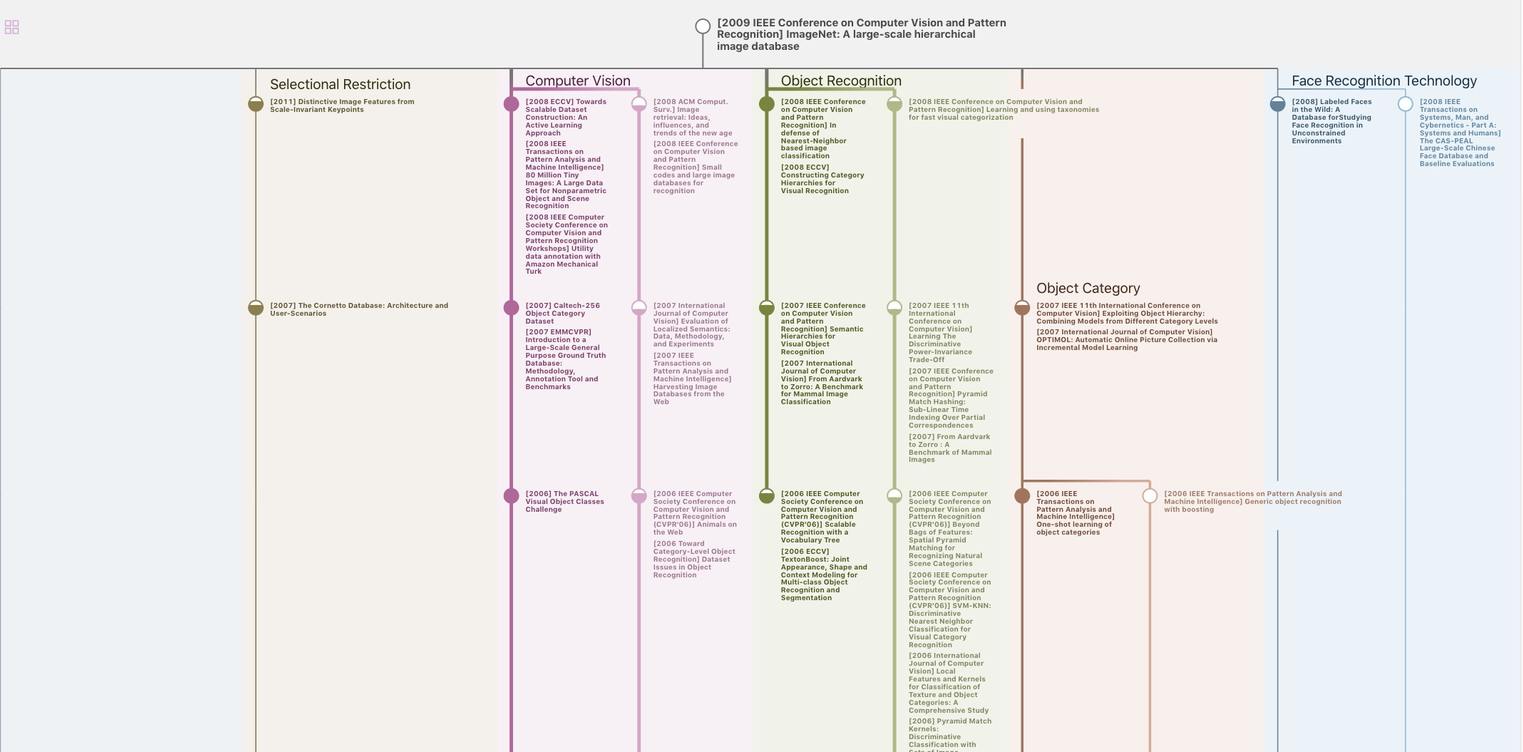
生成溯源树,研究论文发展脉络
Chat Paper
正在生成论文摘要