Radio Map Estimation with Deep Dual Path Autoencoders and Skip Connection Learning
2023 IEEE 34TH ANNUAL INTERNATIONAL SYMPOSIUM ON PERSONAL, INDOOR AND MOBILE RADIO COMMUNICATIONS, PIMRC(2023)
摘要
Radio Map Estimation (RME) is the task of predicting radio power at all locations in a two-dimensional area and at all frequencies in a given band. Accurate estimation is important for applications such as fingerprint based localization, user-cell association and power control in Massive Multiple Input Multiple Output (MIMO) systems, and path planning for Unmanned Aerial Vehicles (UAVs). The problem becomes especially challenging when transmitter locations are unknown and there are obstacles in the environment. In this case the predictions must be based on sampled radio power measurements and environmental information. Recent studies have shown that this can be accomplished using deep learning with Convolutional Neural Network (CNN) based autoencoders. However, these models mix environmental and signal information indiscriminately and do not share information between encoder and decoder. We propose the Dual Path Autoencoder to separate environmental and signal information and Skip Connection Autoencoders to increase information flow between encoder and decoder. We compare and contrast their performance with respect to sampling rate and learning architecture size. We find through experimentation that these two mechanisms independently improve predication accuracy over current state-of-the-art methods but do not appear to work in conjunction with each other.
更多查看译文
关键词
radio map estimation,deep learning,autoencoder,dual path,skip connection
AI 理解论文
溯源树
样例
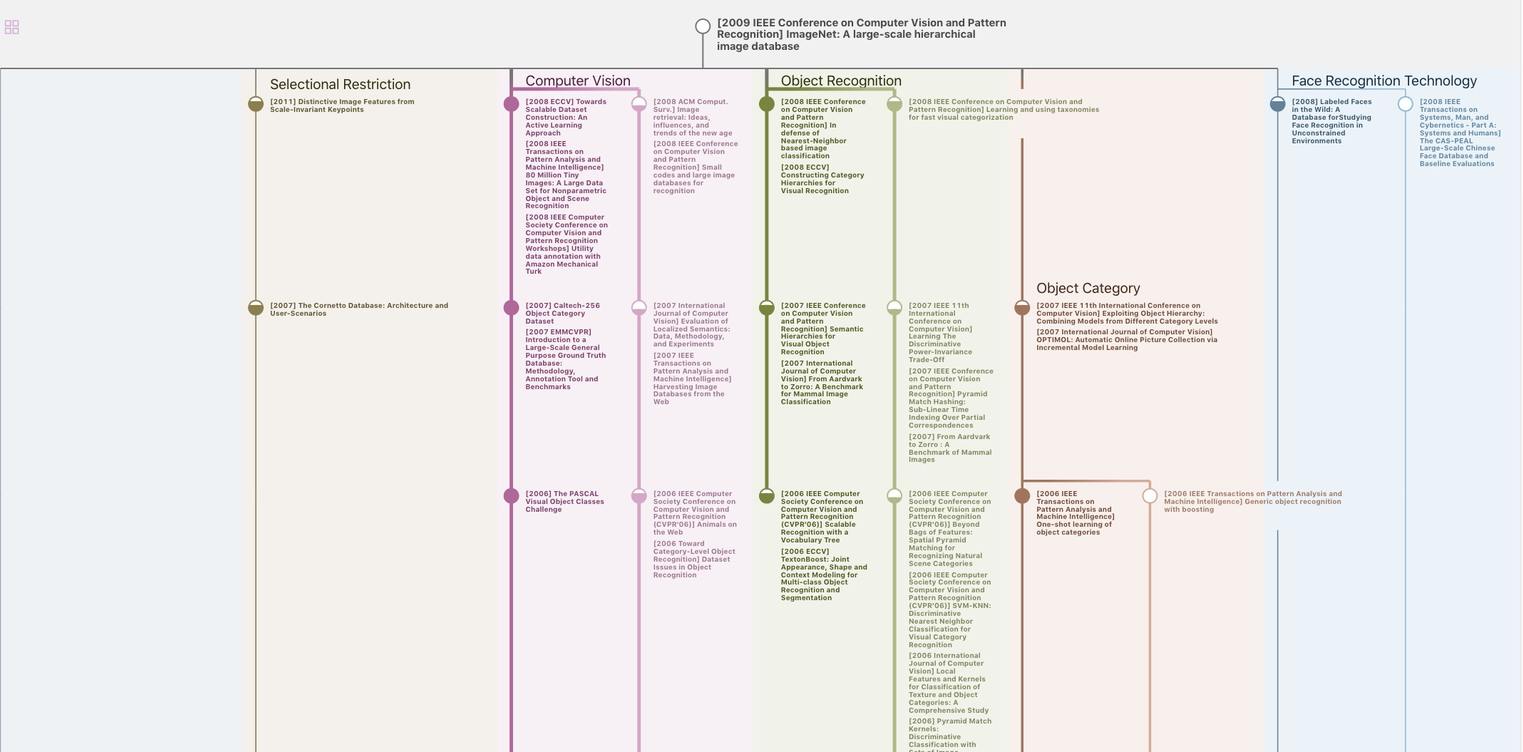
生成溯源树,研究论文发展脉络
Chat Paper
正在生成论文摘要