Novel Probabilistic Reformulation Technique for Unconstrained Discrete RIS Optimization
2023 IEEE 34TH ANNUAL INTERNATIONAL SYMPOSIUM ON PERSONAL, INDOOR AND MOBILE RADIO COMMUNICATIONS, PIMRC(2023)
摘要
Determining optimal phases for a discrete reconfigurable intelligent surface (RIS) in RIS-aided wireless systems is known to be a challenging problem. This paper develops a novel probabilistic reformulation technique to transform such discrete optimization problems into continuous domain problems. The idea is to treat optimization variables as a categorical random vector with independent but non-identically distributed (i.n.i.d.) entries and replace the objective function with its expectation. In the unconstrained case, we rigorously establish the equivalence between the original problem's unique optimal solution and the corresponding degenerate probability density function (PDF) of the transformed problem. Furthermore, we derive key analytical moments and gradients associated with the quadratic form and binary random vectors that are useful in the optimization of RIS-aided wireless systems. In order to concretely demonstrate the benefits of the proposed technique, we reformulate a canonical discrete RIS-aided signal-to-interference-plus-noise ratio (SINR) maximization problem and solve the reformulated problem with the gradient descent (GD) technique. Our solution includes an analytical approach that relies on closed-form approximations for the expectation, incorporating moment results, and a stochastic sampling method based on a log-derivative gradient estimator. Numerical results show that our expectation-based algorithms outperform state-of-the-art conventional algorithms, thereby demonstrating the effectiveness of our approach.
更多查看译文
关键词
Reconfigurable intelligent surface,discrete optimization,categorical random variables
AI 理解论文
溯源树
样例
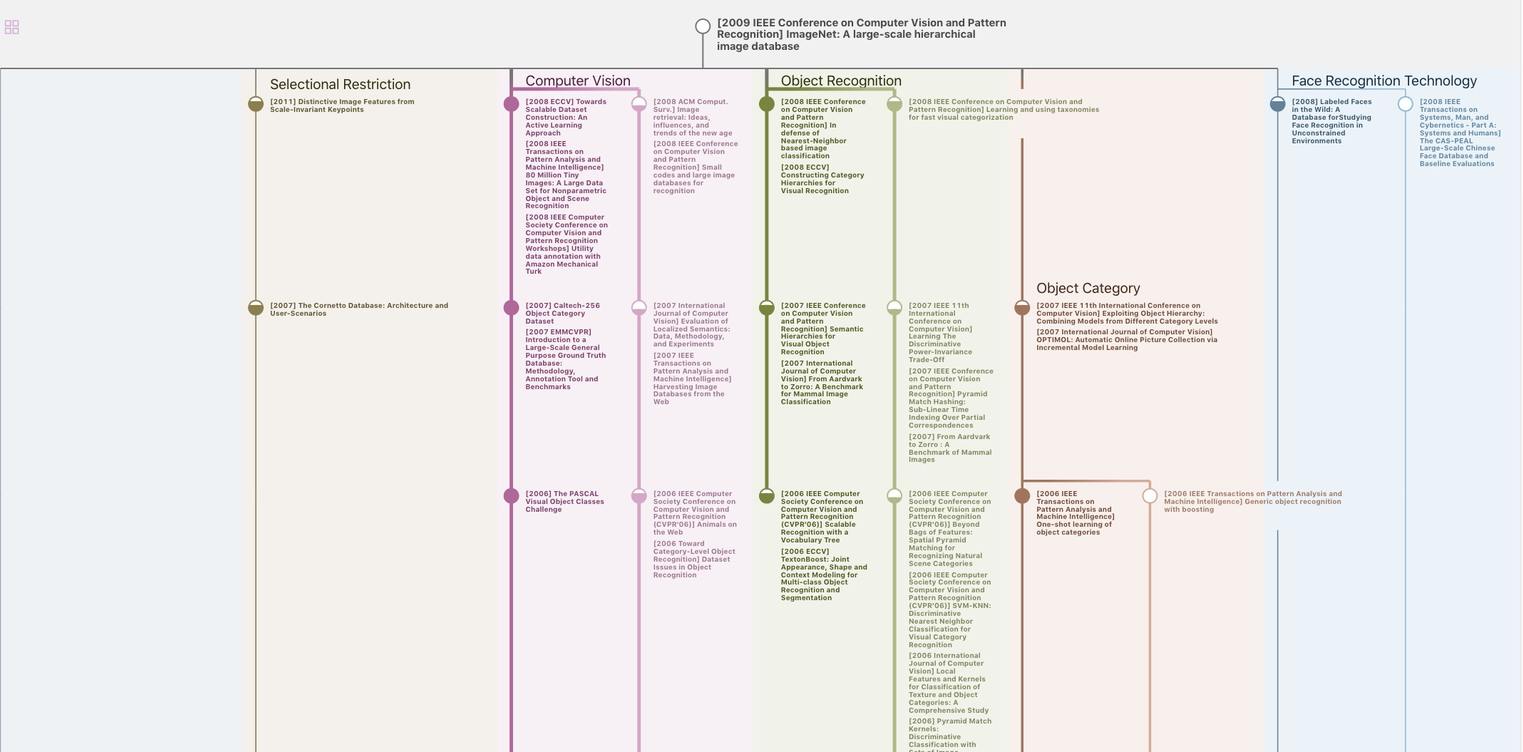
生成溯源树,研究论文发展脉络
Chat Paper
正在生成论文摘要