Multi-Sensor Interactive Perception Graph Attention Networks for Remaining Useful Life Estimation
2023 CAA Symposium on Fault Detection, Supervision and Safety for Technical Processes (SAFEPROCESS)(2023)
摘要
With the increasing intelligence of equipment, prognostics and health management (PHM) has been rapidly developed and highly valued. As more sensors are employed to track the full life of complicated equipment, sensor redundancy becomes a concern. However, most current deep learning-based prediction models cannot effectively perform sensor selection, and do not fully take advantage of the spatiotemporal interaction relationships among the sensors. To address these issues, a Remaining useful life (RUL) estimation method based on multi-sensor and interaction perception, named MultiSensor interaction perception graph attention networks (MIPGATs), is proposed. To achieve effective sensor selection and mining of spatial interaction relationships among sensors, a heterogeneous graph with sensors as nodes is constructed. Based on the graph, the weights of edges and degree centrality of nodes are learned adaptively by attention mechanisms in order to construct interactions between sensors and achieve sensor screening. The remaining edges are then normalized to create the final graph embedding. Finally, graph attention networks (GATs) are employed to implement dynamic learning of the graph embedding over the course of the device's lifespan. The experiments results on the turbofan engine shows that our model achieved effective sensor selection and mining of spatial interaction relationships among them without sacrificing accuracy.
更多查看译文
关键词
Remaining useful life estimation,graph attention networks,interactive perception,multi-sensor signals
AI 理解论文
溯源树
样例
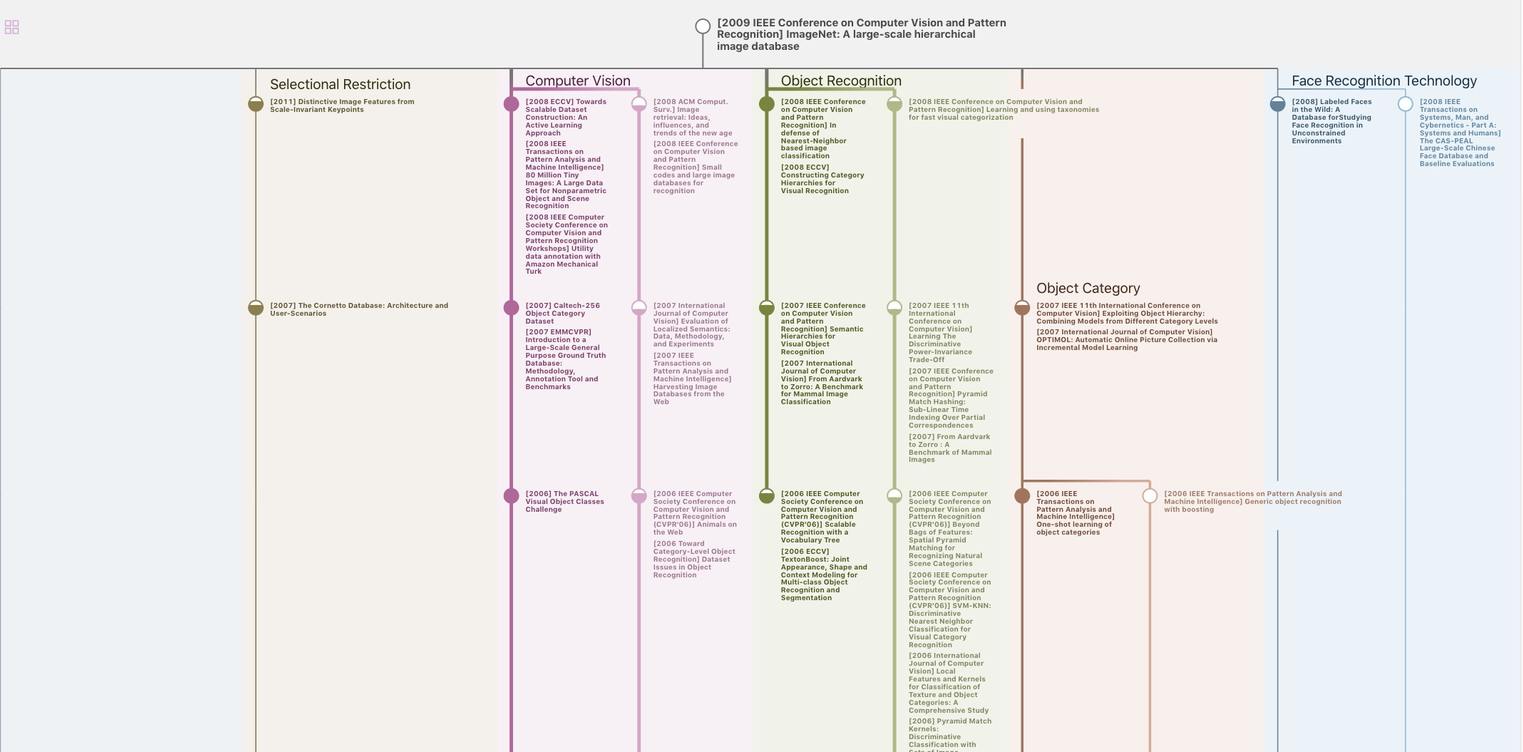
生成溯源树,研究论文发展脉络
Chat Paper
正在生成论文摘要