Adaptive Clustered Federated Learning with Representation Similarity
2023 IEEE 10th International Conference on Data Science and Advanced Analytics (DSAA)(2023)
摘要
Federated learning is a promising machine learning paradigm that enables participating clients to train models collaboratively with privacy restrictions. However, one of the most challenging problems in federated learning is that local data on the clients might come from different distributions. Such data heterogeneity among the clients might influence the performance of federated learning methods. In this paper, we propose FedACRS, an algorithm that deals with heterogeneous data by clustering clients with similar data distributions into groups and then performing federated learning within each group. FedACRS measures the similarity between the clients in every round based on the representation similarity and then adaptively discovers the clustering structure among the clients. In order to cluster the clients appropriately, we provide theoretical analysis to help determine the number of potential clusters. The results of extensive experiments in different settings demonstrate the advantage of FedACRS over the compared methods.
更多查看译文
关键词
federated learning,clustered federated learning,data heterogeneity
AI 理解论文
溯源树
样例
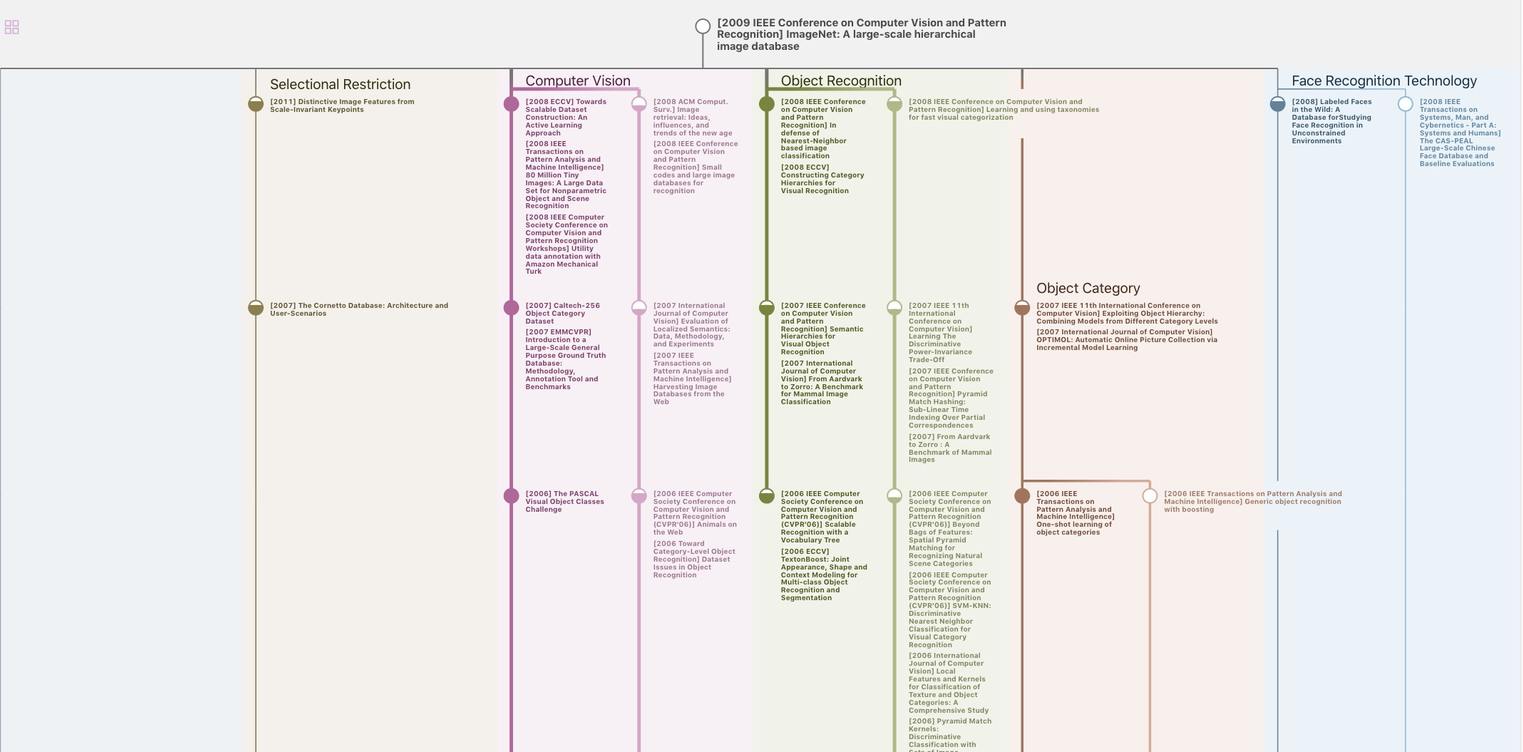
生成溯源树,研究论文发展脉络
Chat Paper
正在生成论文摘要