On the Independence of Adversarial Transferability to Topological Changes in the Dataset
2023 IEEE 10th International Conference on Data Science and Advanced Analytics (DSAA)(2023)
摘要
One curious property of neural networks is the vulnerability to specific attacks, often called adversarial examples. One of the directions adversarial transferability research has taken is to focus on dataset features. The transferability of adversarials is often linked to those common global features being present or not. To validate this theory, we tested if the transferability of attacks occurs when the underlying global features of a dataset remain the same. This is because topology promises to preserve the properties of an object under continuous deformations. In this paper, we test the correlation between topological similarities using the mapper algorithm by Singh et al. to generate an approximation of the topology in a graphical manner and a distance notion provided by the NetLSD algorithm, which promises size, scale, and permutation invariance. These two algorithms allow us to show that adversarial transferability is, in fact, independent of the topological similarity of datasets. We implement our findings in https://github.com/KDD-OpenSource/Topological-Transf. This is an astounding new insight, as former theories have led us to expect that if the assumption is true that global features are relevant for transferability, those should be captured using algorithms that detect global features under only topological change- Unless, of course, the transferability and those global features are explicitly agnostic to topological change. This might point to current research regarding adversarial transferability in different directions. More specifically, we take an experimental approach using topological approximation methods to capture essential features of datasets. Past studies concerning adversarial examples show that attacks can transfer in unforeseen ways and between different neural network architectures and may produce severe vulnerabilities in sophisticated learners. However, when tackling the problem of vulnerabilities to adversarial attacks, only a few approaches find generalizable results, and by no means have we answered when and how to attack transferability can occur. This paper shows that if we limit changes in a dataset to topological permutations, the transferability of adversarial examples generated will stay the same regardless of the amount of topological change. Since acceptance of the paper, we have actually extended our implementation to other adversarial methods by simply including given code from more methods into the general implementation. The code base is also easily extendable to other datasets for further reproducibility.
更多查看译文
关键词
Adversarial examples,Adversarial transferability,Topological Data Analysis
AI 理解论文
溯源树
样例
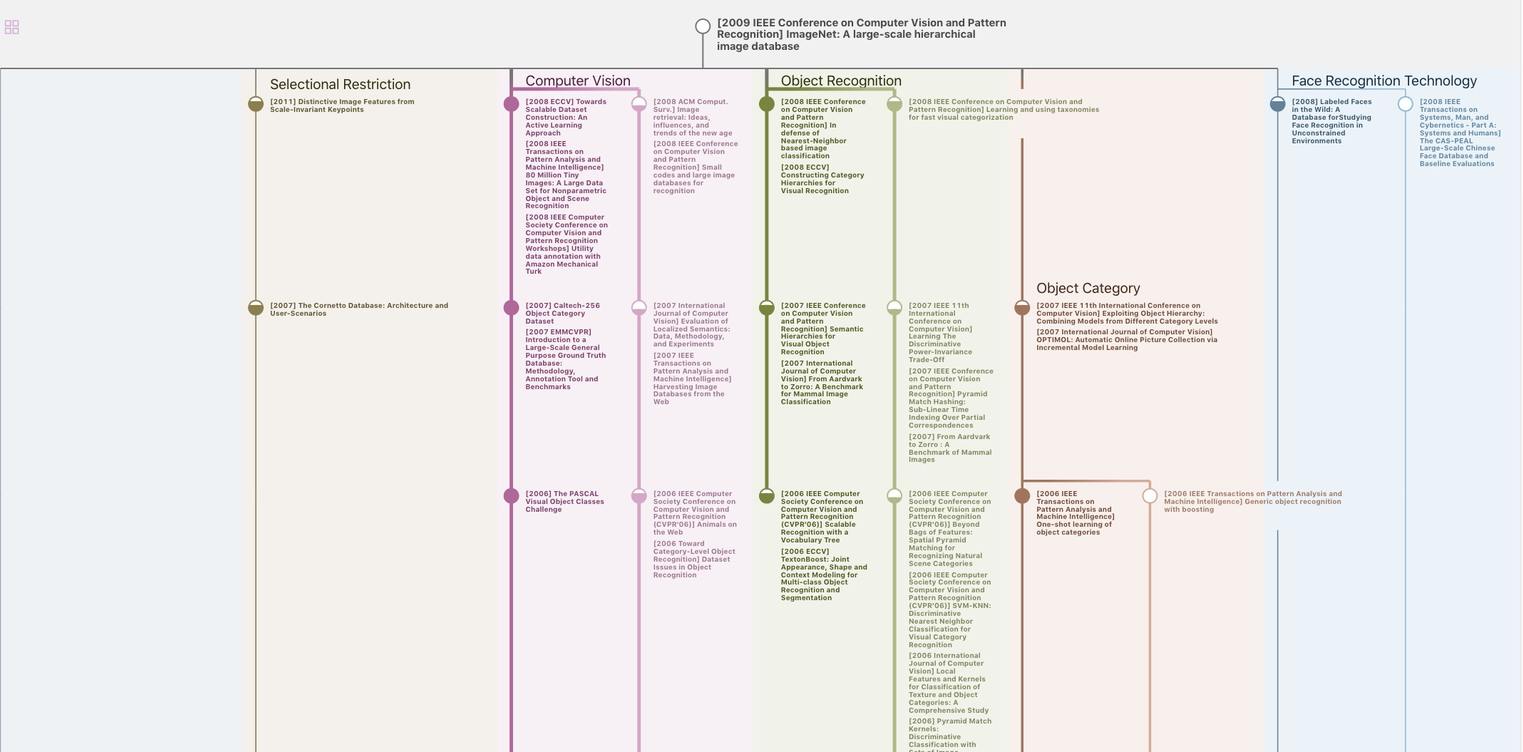
生成溯源树,研究论文发展脉络
Chat Paper
正在生成论文摘要