1NN-DTW ARIMA LSTM: A new ensemble for forecasting multi-domain/multi-context time series
2023 IEEE 10th International Conference on Data Science and Advanced Analytics (DSAA)(2023)
摘要
The measurements of several sensors of multiple machines are collected in real-time at Alfa Laval’s IIoT platform. It is of great interest to make an accurate forecast for upcoming events, such as machine failure or sensor faults, which are critical enablers for predictive maintenance. However, some machines have little historical data, making forecasting challenging for them. Can we use data from other devices to make a forecast on a newly installed machine or improve the forecasting performance on different machines? This is the central question I try to answer in this research. However, another dimension of complexity makes the problem even more difficult. The devices have some differences. They can vary in version, application, and configuration. In addition, at each instant, the machine can have different modes and user-defined parameters that can be changed anytime by the operator or machine auto-control. All these factors make the forecasting problem extremely challenging, so it does not fit into a general computational framework. I propose a new multi-paradigm ensemble framework, aware of context and domain, that significantly improves the popular ensemble methods and non-ensemble state-of-the-art such as ARIMA and LSTM. The improvement mostly comes from adding a new forecasting paradigm based on multi-domain INN-DTW (one-nearest neighbor with dynamic time warping as a similarity measure) to the ensemble architecture, which creates a surprising harmony with ARIMA. INN-DTW is popular in time series classification, but its application is being investigated for the first time in a heterogeneous ensemble. The other novel component is a meta-selection mechanism for aggregating forecasts, which unexpectedly works better than the widely-used meta-learning approach.
更多查看译文
关键词
time series forecasting,contextual/multi-domain time series,heterogeneous ensemble
AI 理解论文
溯源树
样例
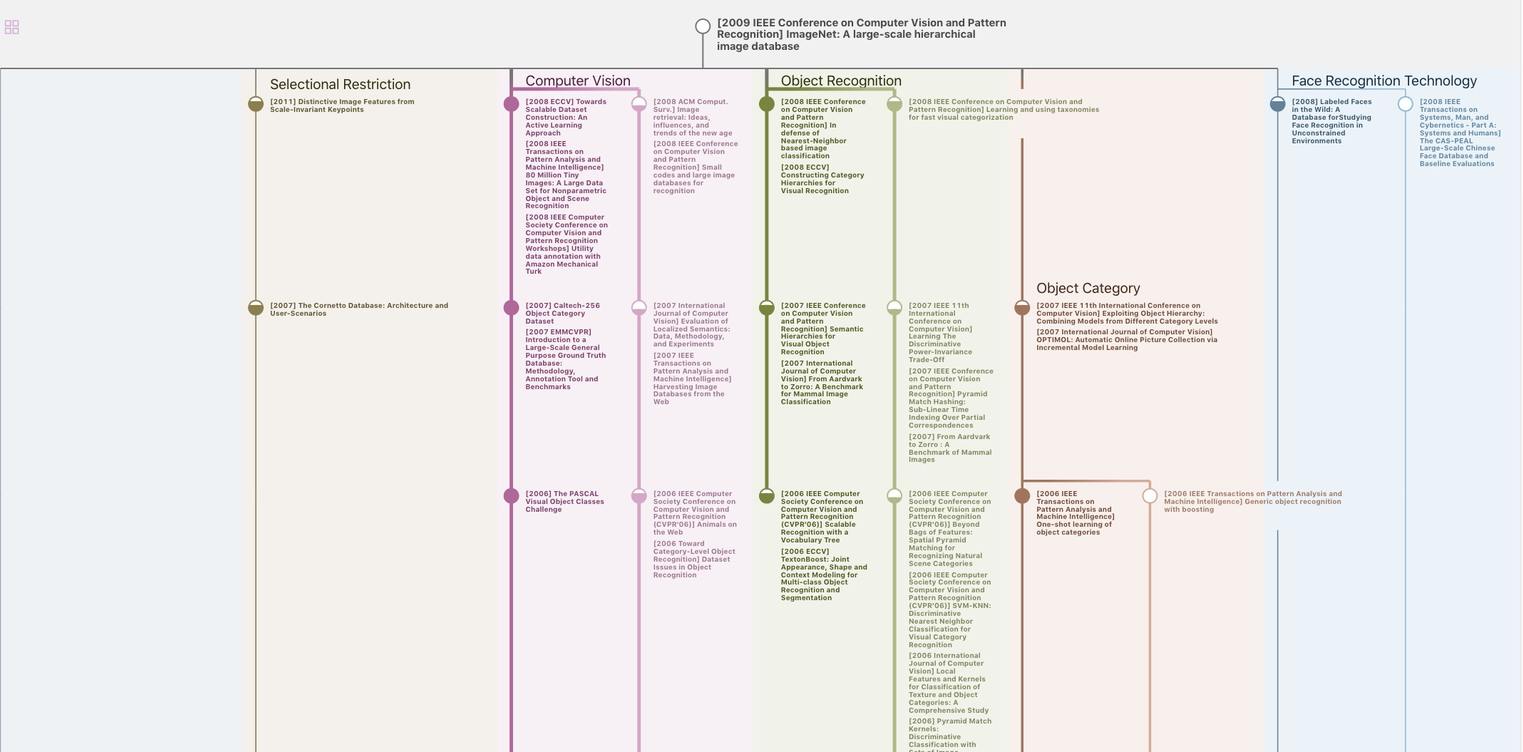
生成溯源树,研究论文发展脉络
Chat Paper
正在生成论文摘要