Improved fascicle length estimates from ultrasound using a U-net-LSTM framework
2023 INTERNATIONAL CONFERENCE ON REHABILITATION ROBOTICS, ICORR(2023)
摘要
Brightness-mode (B-mode) ultrasound has been used to measure in vivo muscle dynamics for assistive devices. Estimation of fascicle length from B-mode images has now transitioned from time-consuming manual processes to automatic methods, but these methods fail to reach pixel-wise accuracy across extended locomotion. In this work, we aim to address this challenge by combining a U-net architecture with proven segmentation abilities with an LSTM component that takes advantage of temporal information to improve validation accuracy in the prediction of fascicle lengths. Using 64,849 ultrasound frames of the medial gastrocnemius, we semi-manually generated ground-truth for training the proposed U-net-LSTM. Compared with a traditional U-net and a CNN-LSTM configuration, the validation accuracy, mean square error (MSE), and mean absolute error (MAE) of the proposed U-net-LSTM show better performance (91.4%, MSE = 0.1 +/- 0.03 mm, MAE = 0.2 +/- 0.05 mm). The proposed framework could be used for real-time, closed-loop wearable control during realworld locomotion.
更多查看译文
AI 理解论文
溯源树
样例
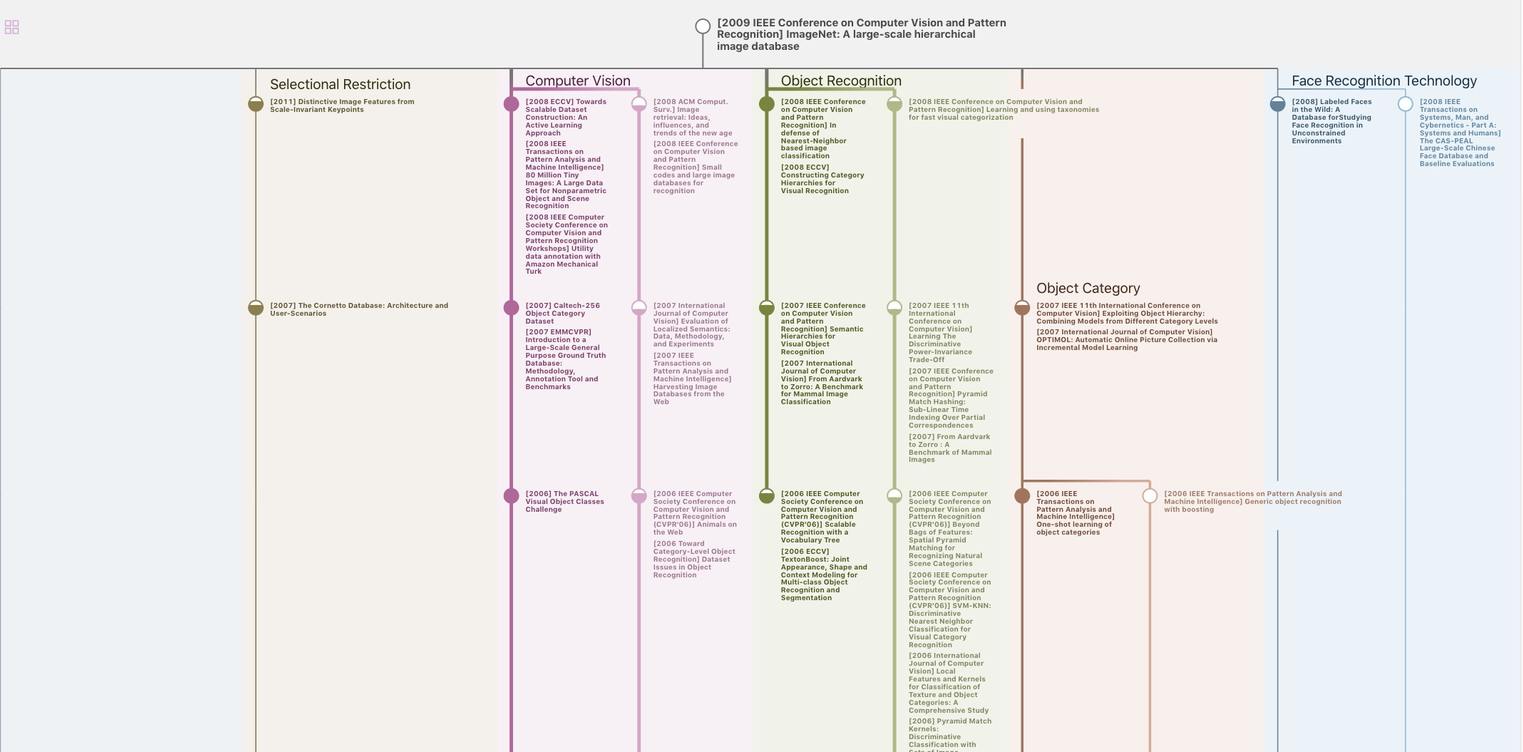
生成溯源树,研究论文发展脉络
Chat Paper
正在生成论文摘要