Neural Network-Based Safety Optimization Control for Constrained Discrete-Time Systems
IECON 2023- 49th Annual Conference of the IEEE Industrial Electronics Society(2023)
摘要
This paper proposes a constraint-aware safety control approach via adaptive dynamic programming (ADP) to address the control optimization issues for discrete-time systems subjected to state constraints. First, the constrained control framework is established via the primal-dual approach with the modified Lagrangian based on the relaxed barrier function. Herein, the Lagrangian multiplier is designed to achieve the trade-off between optimization performance and state constraints. In addition, the sub optimality of the dual method is built by proving that the dual gap can be arbitrarily small. And the value iteration algorithm is utilized to implement the constraint-aware ADP controller. Furthermore, the weight estimation error is proved to be bounded when the learning rate satisfies a given sufficient condition. Finally, numerical simulation proves the effectiveness and superiority of the proposed method.
更多查看译文
关键词
State constraints,safety control,nonlinear discrete- time system,neural networks
AI 理解论文
溯源树
样例
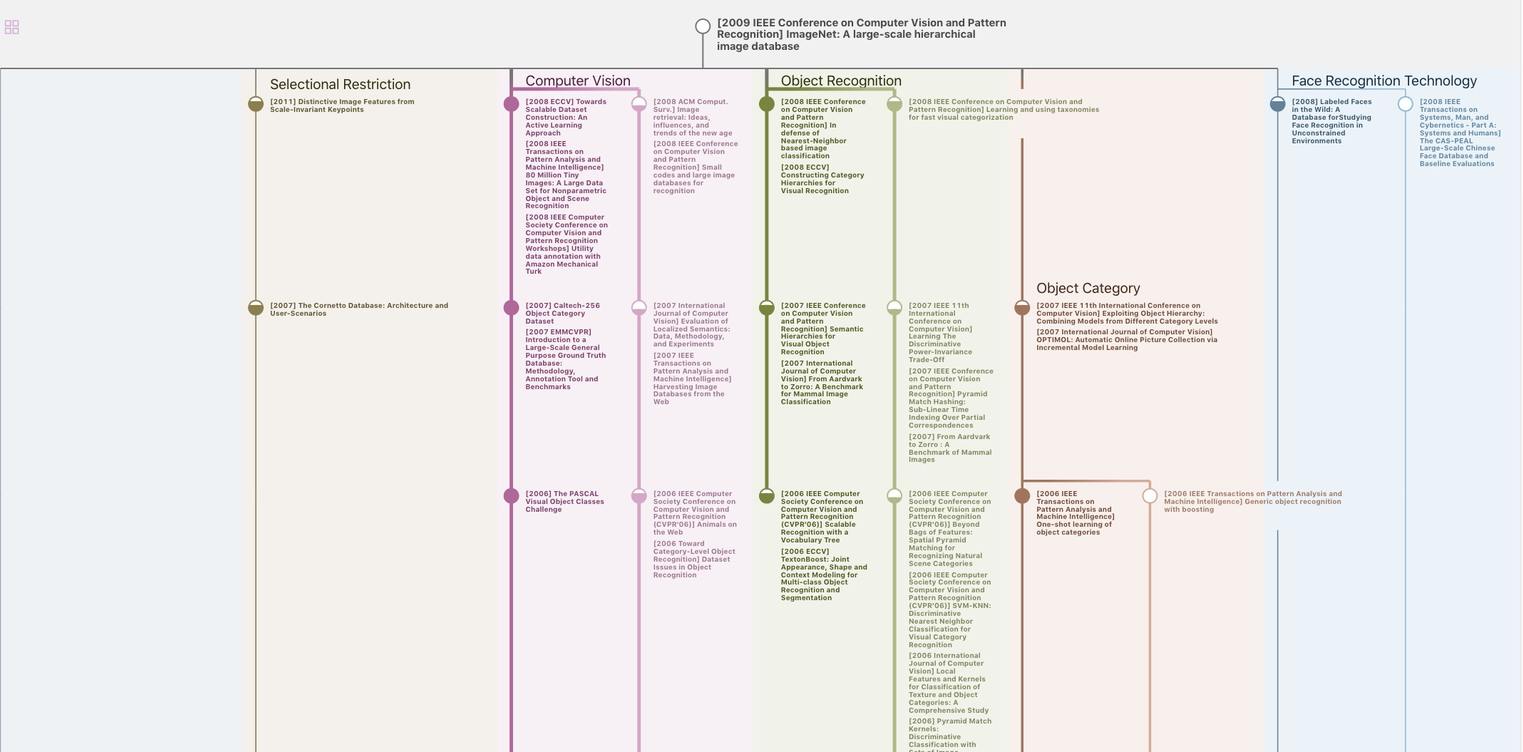
生成溯源树,研究论文发展脉络
Chat Paper
正在生成论文摘要