Efficient and Scalable Parametric High-Order Portfolios Design via the Skew-$t$ Distribution
IEEE Transactions on Signal Processing(2023)
摘要
Since Markowitz's mean-variance framework, optimizing a portfolio that strikes a trade-off between maximizing profit and minimizing risk has been ubiquitous in the financial industry. Initially, profit and risk were measured by the first two moments of the portfolio's return, a.k.a. the mean and variance, which are sufficient to characterize a Gaussian distribution. However, it is broadly believed that the first two moments are not enough to capture the characteristics of the returns’ behavior, which have been recognized to be asymmetric and heavy-tailed. Although portfolio designs involving the third and fourth moments, i.e., skewness and kurtosis, have been demonstrated to outperform the conventional mean-variance framework, they present non-trivial challenges. Specifically, in the classical framework, the memory and computational cost of computing the skewness and kurtosis grow sharply with the number of assets. To alleviate the difficulty in high-dimensional problems, we consider an alternative expression for high-order moments based on parametric representations via a generalized hyperbolic skew-
$\boldsymbol{t}$
distribution. Then, we reformulate the high-order portfolio optimization problem as a fixed-point problem and propose a robust fixed-point acceleration algorithm that solves the problem in an efficient and scalable manner. Empirical experiments also attest to the efficiency of our proposed high-order portfolio optimization framework, which presents low complexity and significantly outperforms the state-of-the-art methods by
$\mathbf{2}\boldsymbol{\sim}\mathbf{4}$
orders of magnitude.
更多查看译文
关键词
High-order portfolios,generalized hyperbolic skew- $t$ distribution,fixed point acceleration
AI 理解论文
溯源树
样例
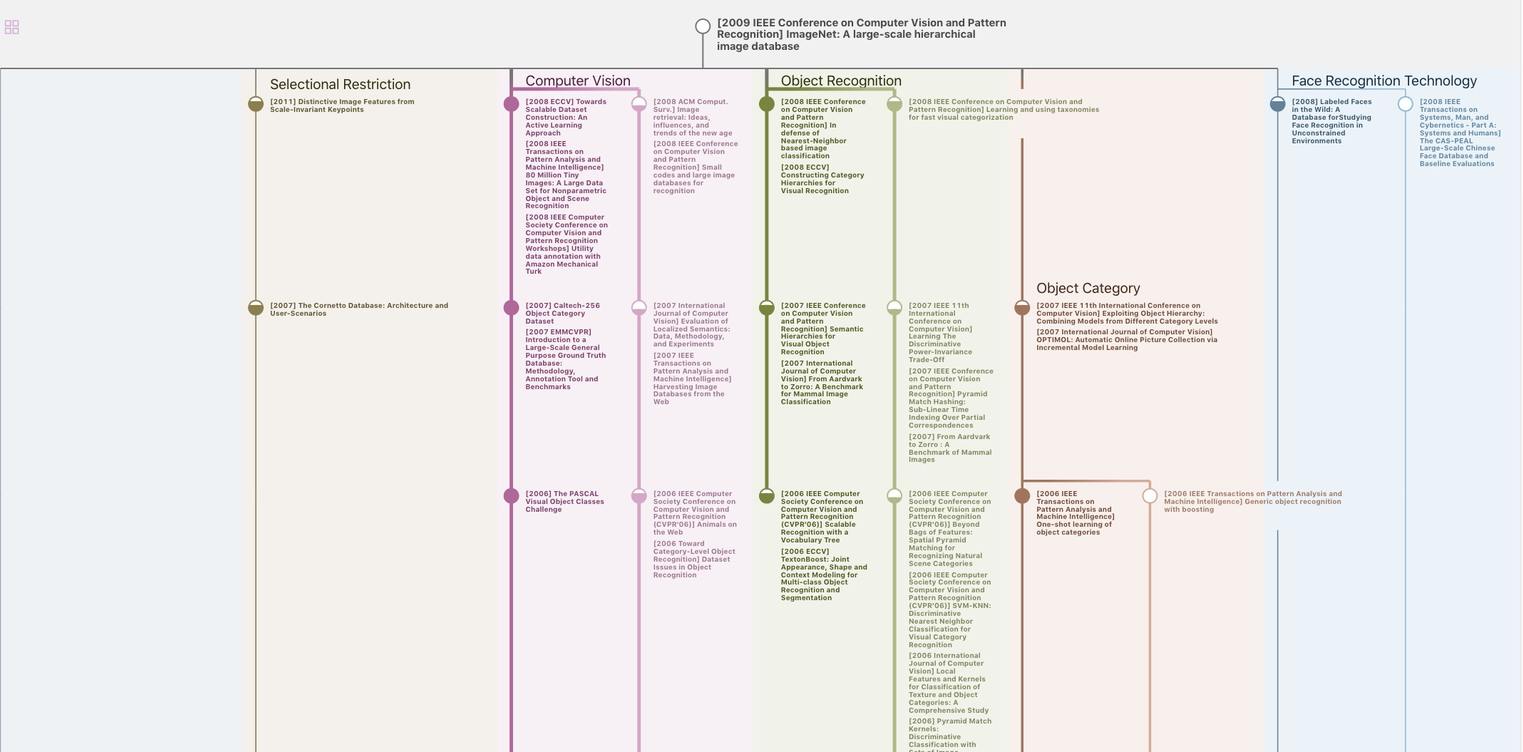
生成溯源树,研究论文发展脉络
Chat Paper
正在生成论文摘要