Robust Near-Optimal Arm Identification With Strongly-Adaptive Adversaries
IEEE TRANSACTIONS ON SIGNAL PROCESSING(2023)
摘要
In this work, we study the best arm identification problem in the adversarial multi-armed bandits framework. We define a strongly-adaptive adversarial model in this framework, based on strongly-adaptive adversaries in security and distributed systems. On the negative side, we show the increased strength of the adversarial model by proving that it is impossible for any best-arm identification algorithm to return an arm with rank <= [ (epsilon K)1 +epsilon 0 ] , where K is the number of arms, c is the adversary's budget and c0 is the breaking point of the robust mean estimation subroutine. On the positive side, we construct a novel sequential elimination algorithm which returns a near-optimal arm (with rank <= [ (1 +lambda) [(epsilon K )(epsilon 0) ] ] where lambda > 0 is a function of epsilon and epsilon(0) and tends to 0 for small epsilon) with high probability. We evaluate our algorithm on both synthetic and real-world datasets and empirically demonstrate that our algorithm returns a near optimal arm under a strongly-adaptive adversarial model.
更多查看译文
关键词
Multi-armed bandit problem,Multi-armed bandits,best arm identification,strongly-adaptive adversaries,sequential decision-making
AI 理解论文
溯源树
样例
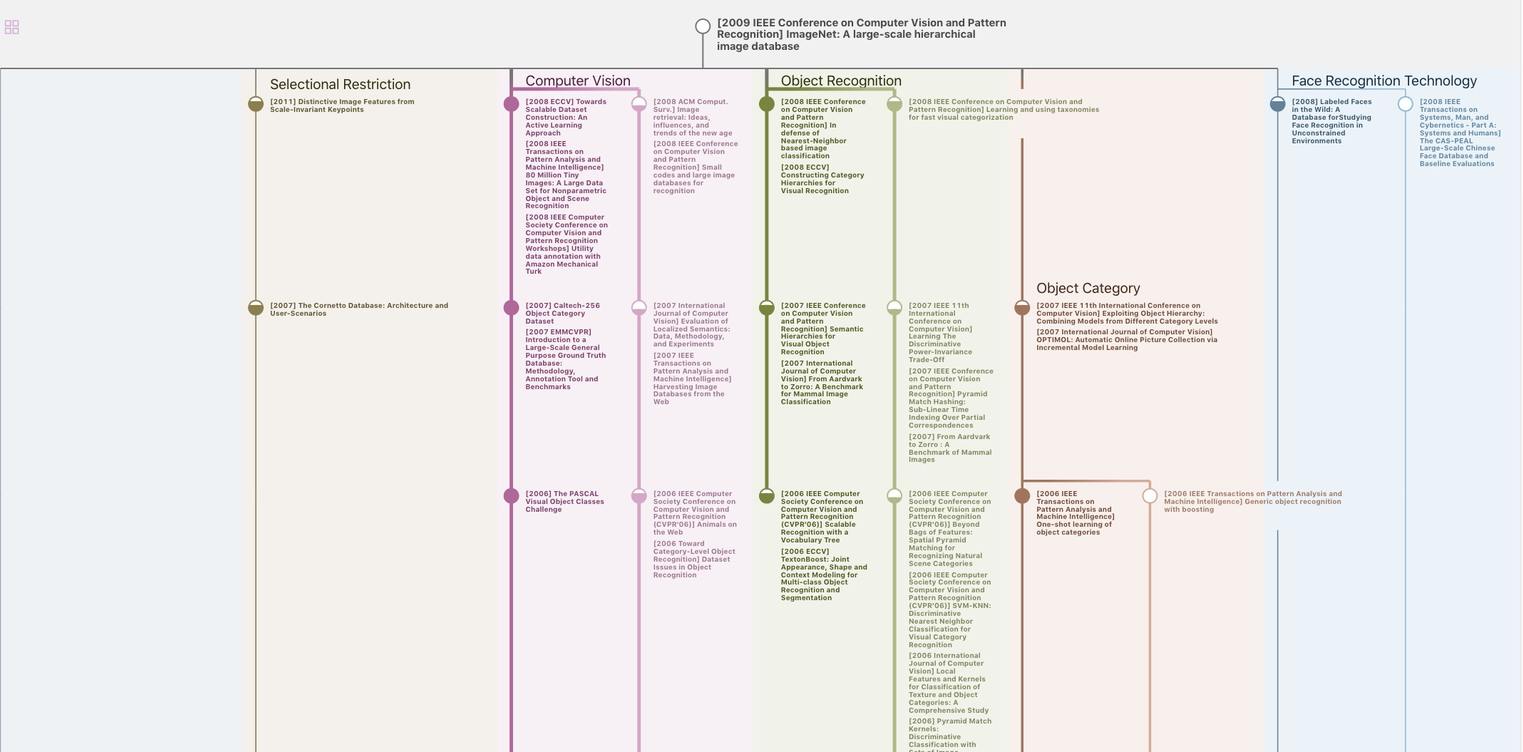
生成溯源树,研究论文发展脉络
Chat Paper
正在生成论文摘要