Seq2Dense U-Net: Analyzing Sequential Inertial Sensor Data for Human Activity Recognition Using Dense Segmentation Model
IEEE Sensors Journal(2023)
摘要
In this article, we have demonstrated a novel, generalized Seq2Dense U-Net model for classifying different activities (time-series data obtained from an accelerometer and gyroscope) and sampling them by considering individual sampling points. For training and classifying activities, the triaxial data from the smart devices are mapped as a linear set of sampling points via the sliding window technique and fed to the model. Our proposed model overcame the challenge of a multiclass window that incorrectly labels different classes of sampling points within a window as a class, as reported by previous studies. The comparative study shows that our method can recognize pixel-level function from corresponding time-series data of human activities. To validate and consolidate the effectiveness of the model, an ablation study has been performed on the components of the classification block. Furthermore, the network depth analysis provides the optimum depth with respect to the number of parameters. We have performed extensive studies on the Sanitation, UCI, and UCI HAPT datasets and calculated various performance metrices to assess the performance of our proposed model. Our proposed model performed significantly better on every individual dataset compared to other models as illustrated in the comparative study and provided the best results on the UCI dataset. For the UCI HAR dataset, our model gives an accuracy score of 0.954781, precision of 0.941473, recall of 0.940618, F1 score of 0.940699 followed by Matthew’s correlation coefficient (MCC), and kappa score of 0.948767 and 0.948630, on test data, respectively.
更多查看译文
关键词
human activity recognition,sequential inertial sensor data,seq2dense segmentation model,u-net
AI 理解论文
溯源树
样例
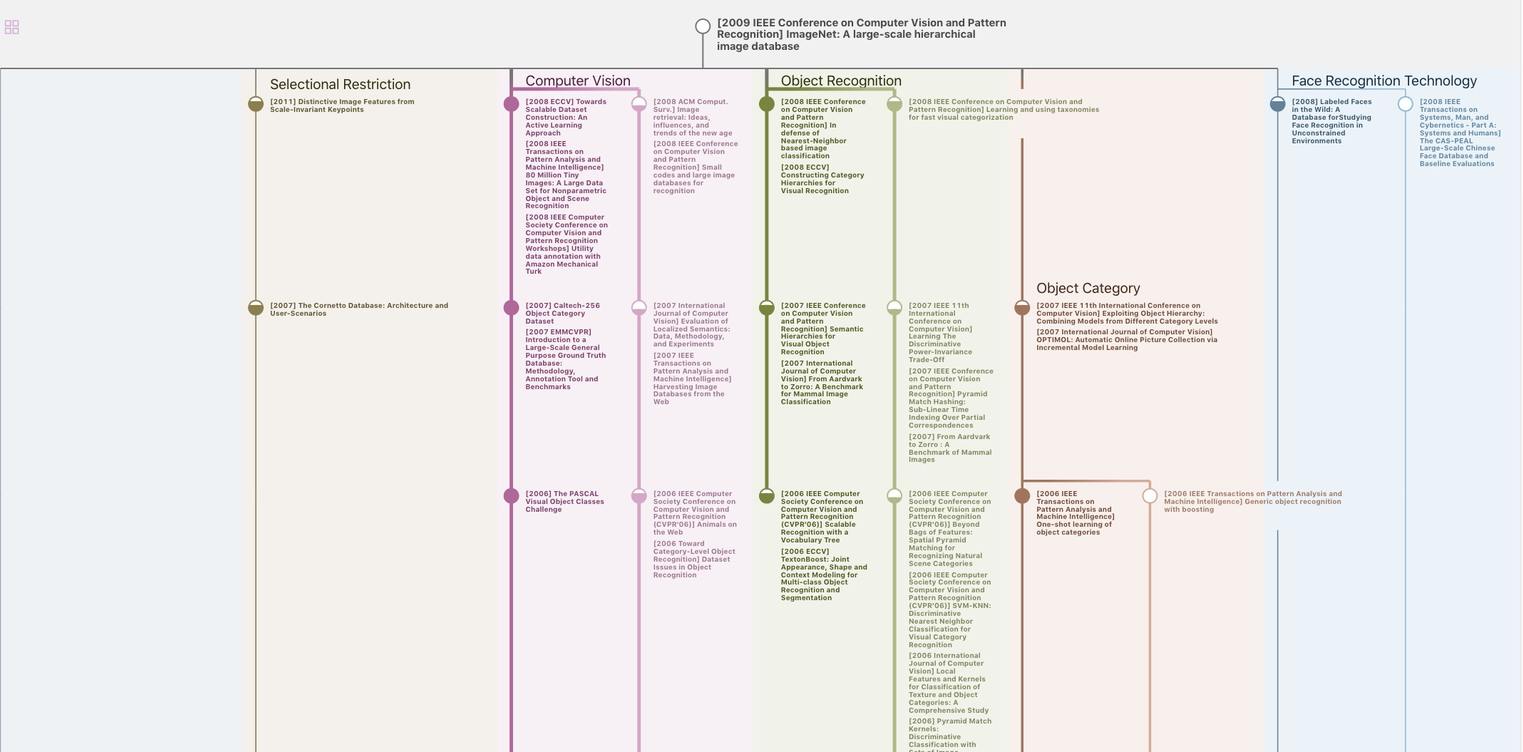
生成溯源树,研究论文发展脉络
Chat Paper
正在生成论文摘要