Reinforcement-and-Alignment Multispectral Object Detection Using Visible-Thermal Vision Sensors in Intelligent Vehicles
IEEE SENSORS JOURNAL(2023)
摘要
Multispectral object detection using visible-thermal vision sensors is a crucial method for enabling intelligent vehicles to perceive their environment accurately. However, fusing and complementing multispectral information is challenging because of problems such as miscalibration resulting from disparate camera fields of view (FOV) and pattern discrepancies in images captured at different wavelengths. To address these challenges, we propose a novel multispectral object detection system named the reinforcement- and alignment-based fusion model (RA-FMDet). This system integrates a cross-modality reinforcement (CMR) module with a hierarchical modality alignment (HMA) strategy. The CMR module uses hybrid transformer-convolutional neural network (CNN) architecture for the effective extraction of high-quality semantic representations from multispectral data. The HMA strategy addresses miscalibration at the pixel and anchor levels. To evaluate RA-FMDet, we established a comprehensive dataset covering various scenarios and conditions. This dataset was obtained by optimizing the hardware configuration of visible-thermal vision sensors and mitigating the shift between image modalities through the use of a modified calibration board. Extensive experiments conducted on the challenging KAIST dataset and the established dataset indicated that the proposed RA-FMDet outperformed state-of-the-art models in object detection and achieved mean average precision values of 92.60%, 93.37%, and 93.60% in the detection of cars, pedestrians, and motorcyclists, respectively.
更多查看译文
关键词
Multilevel sensor fusion,multispectral object detection,visible-thermal camera calibration,visible-thermal vision sensors
AI 理解论文
溯源树
样例
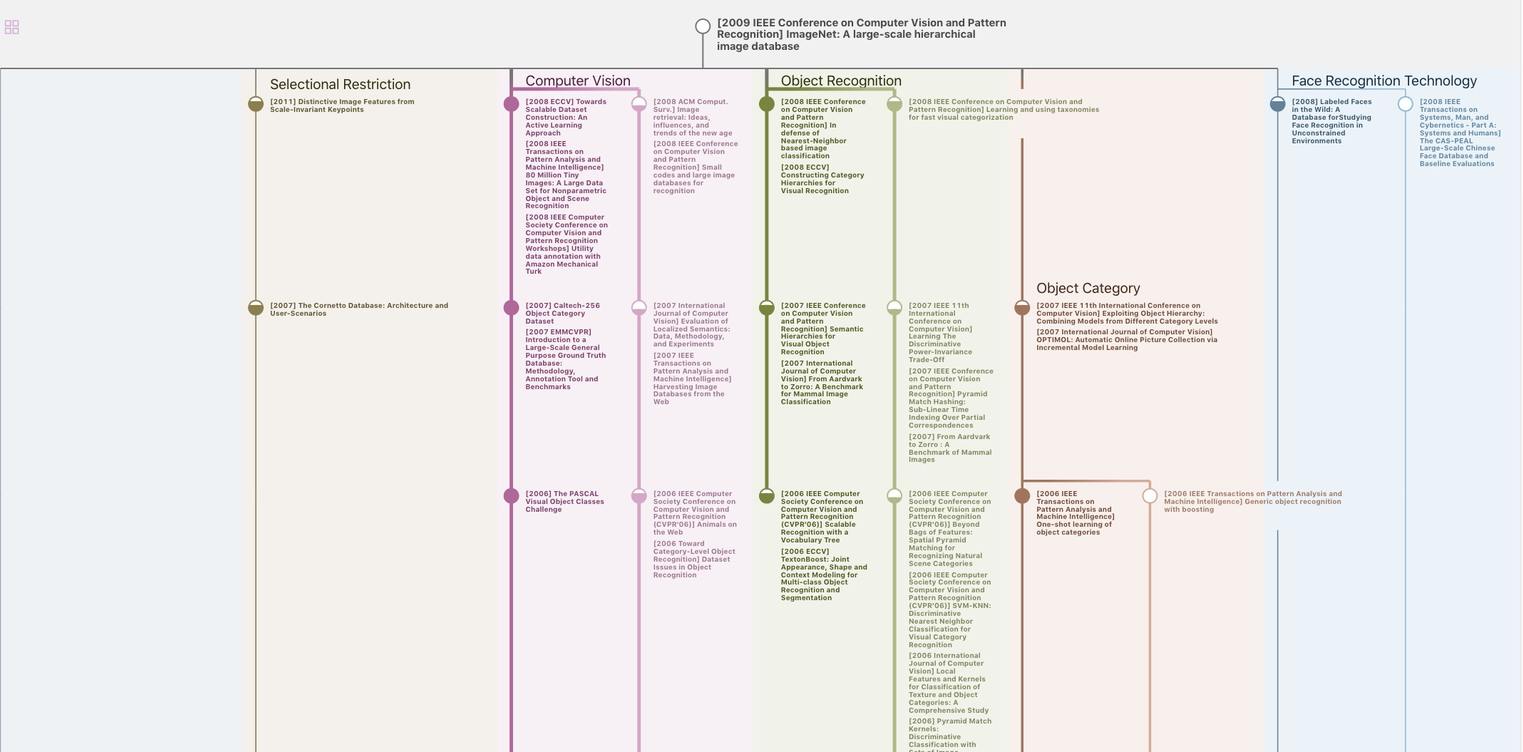
生成溯源树,研究论文发展脉络
Chat Paper
正在生成论文摘要