DSDAN: Dual-Step Domain Adaptation Network Based on Bidirectional Knowledge Distillation for Cross-User Myoelectric Pattern Recognition
IEEE SENSORS JOURNAL(2023)
摘要
Myoelectric control is a typical modality of neural interfaces always driven by pattern recognition of surface electromyogram (sEMG). However, due to great cross-user variability, it suffers from severe performance degradation when it is trained on one set of user data and then tested with new user data. To improve its cross-user usability, a dual-step domain adaptation network (DSDAN) is proposed to fully exploit useful information in the training data (source domain) and efficiently deliver it to the testing data from a new user (target domain). The DSDAN is based on bidirectional knowledge distillation (KD) and uses a teacher-student model to implement the unsupervised domain adaptation (UDA), which allows the classification model to adapt to a new user in the testing phase without any labeled data. A bidirectional KD technique was adopted to facilitate mutual learning between the teacher and student networks to address the problem of balancing adaptation speed and accuracy in the UDA. A novel dual-step domain adaptation (DSDA) strategy was utilized to mitigate knowledge obsolescence and error accumulation problems in KD. We evaluated the performance of the DSDAN framework on both high- and low-density sEMG datasets and achieved 98.96 +/- 4.91% and 98.09 +/- 1.86% recognition accuracies, respectively, which are better than those of mainstream UDA methods (p < 0.01). This study demonstrates the effectiveness and robustness of the DSDAN framework in a cross-user myoelectric control task, which could be applied in gestural interfaces and intelligent robotic control.
更多查看译文
关键词
Cross-user variability,gestural interfaces,knowledge distillation (KD),myoelectric control,unsupervised domain adaptation (UDA)
AI 理解论文
溯源树
样例
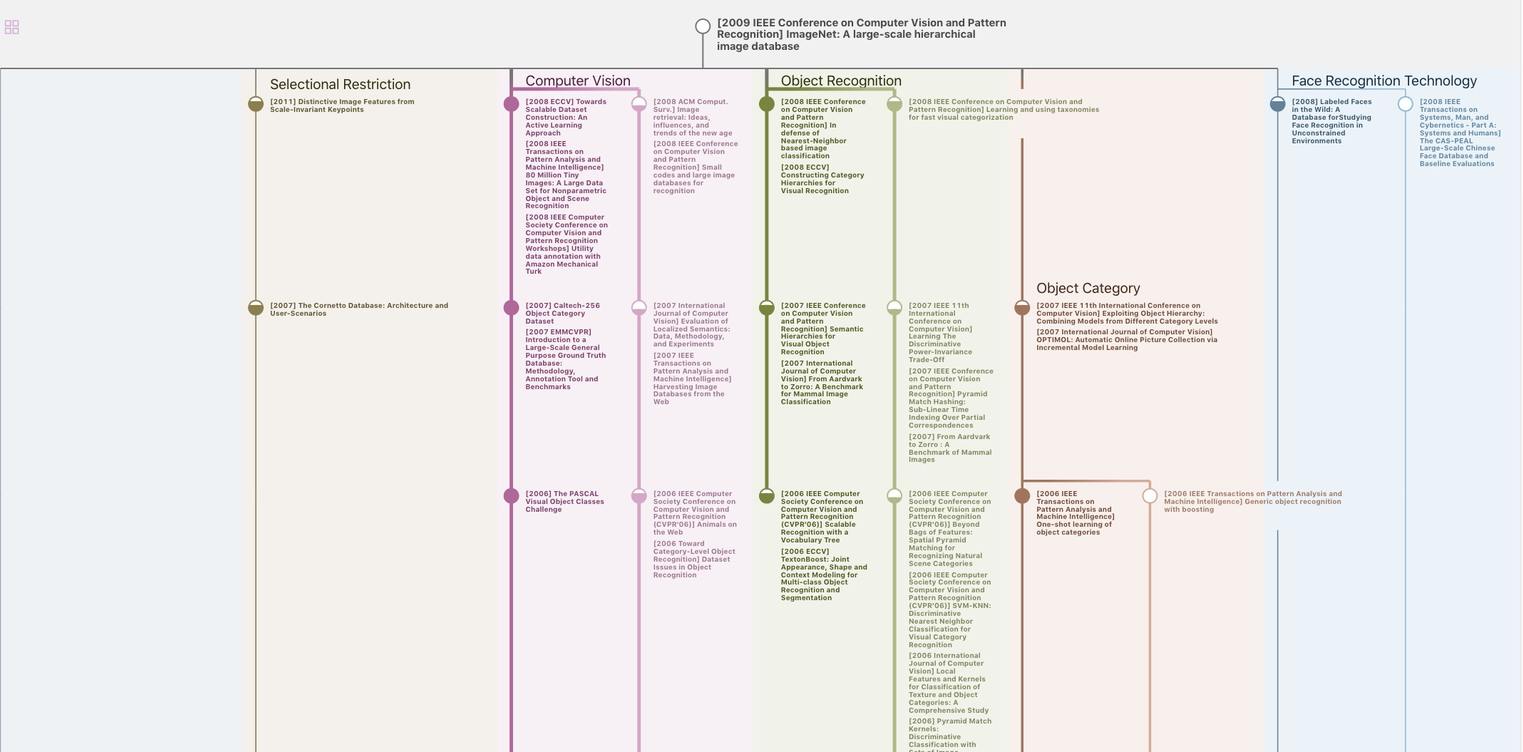
生成溯源树,研究论文发展脉络
Chat Paper
正在生成论文摘要