Low-Density EEG Correction With Multivariate Decomposition and Subspace Reconstruction
IEEE Sensors Journal(2023)
摘要
A hybrid method is proposed for removing artifacts from electroencephalographic (EEG) signals. This relies on the integration of artifact subspace reconstruction (ASR) with multivariate empirical mode decomposition (EMD). The method can be applied when few EEG sensors are available, a condition in which existing techniques are not effective, and it was tested with two public datasets: 1) semisynthetic data and 2) experimental data with artifacts. One to four EEG sensors were taken into account, and the proposal was compared to both ASR and multivariate EMD (MEMD) alone. The proposed method efficiently removed muscular, ocular, or eye-blink artifacts on both semisynthetic and experimental data. Unexpectedly, the ASR alone also showed compatible performance on semisynthetic data. However, ASR did not work properly when experimental data were considered. Finally, MEMD was found less effective than both ASR and MEMD-ASR.
更多查看译文
关键词
Artifact removal, electroencephalography, empirical mode decomposition (EMD), few sensors, low cost
AI 理解论文
溯源树
样例
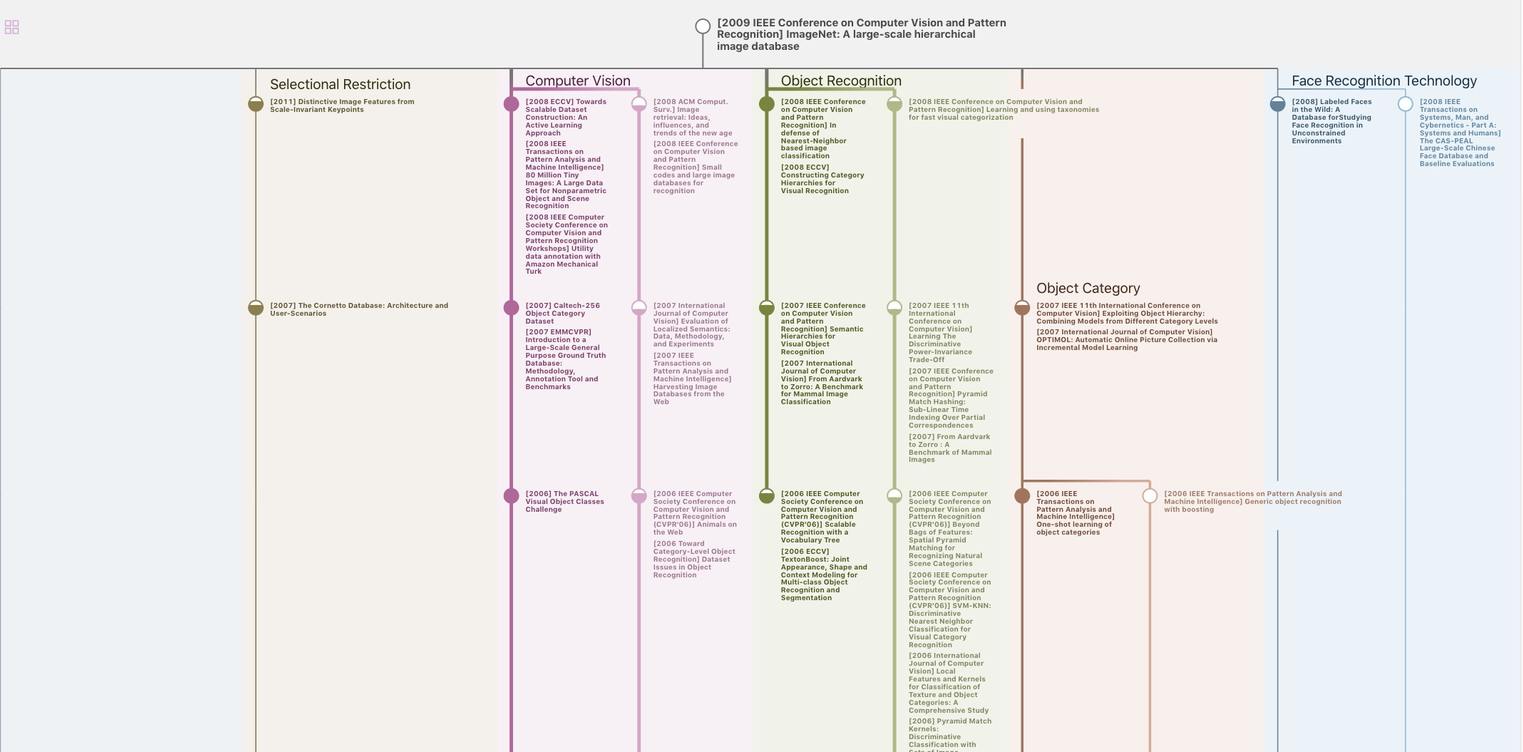
生成溯源树,研究论文发展脉络
Chat Paper
正在生成论文摘要