DNN-Based Legibility Improvement for Air-Writing in Millimeter-Waveband Radar System
IEEE Transactions on Instrumentation and Measurement(2023)
摘要
In radar-based air-writing, continuous measurements of hand movements may result in the addition of unnecessary strokes for certain characters or digits (e.g., 4 and 5), making it difficult to accurately recognize the air-written results when observed by human eyes. Therefore, we propose a deep neural network (DNN)-based classifier designed to identify unnecessary strokes and clutter that arise during the radar-based air-writing. First, while air-writing the digits from 0 to 9, the range, angle, and signal amplitude of the hand movement are obtained through a radar system. Then, we represent the hand’s trajectory in the form of
$x$
and
$y$
coordinates using the information of range and angle. Next, we train the DNN-based classifier using the acquired
$x$
and
$y$
coordinates, signal amplitude, and frame index as input features. To ensure the classifier’s performance would not be impacted by the changes in the position and size of the air-writing area, we apply the normalization to the
$x$
and
$y$
coordinates. Finally, the performance of the classifier is verified using the results of air-writing digits from 0 to 9. The proposed method identifies unnecessary strokes and clutter regardless of the position and size of the air-writing area, demonstrating an average classification accuracy of 94.57%. Furthermore, when the classifier was validated with different individuals conducting the air-writing, the classifier exhibited an average classification accuracy of 93.9%.
更多查看译文
关键词
Radar, Discrete Fourier transforms, Radar antennas, Radar imaging, Clutter, Writing, Vision sensors, Air-writing, deep neural network (DNN), digit recognition, frequency-modulated continuous wave (FMCW) radar, legibility improvement, target classification
AI 理解论文
溯源树
样例
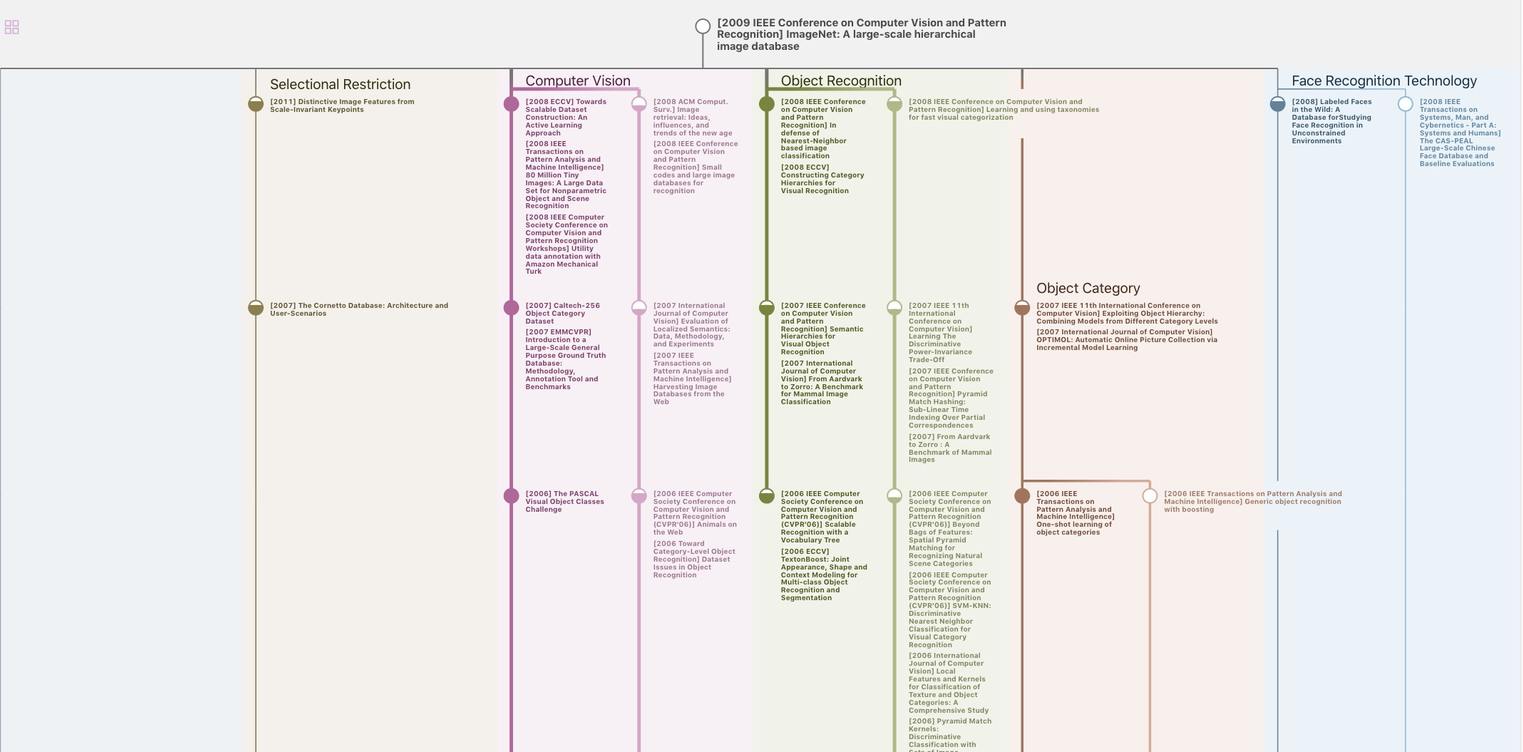
生成溯源树,研究论文发展脉络
Chat Paper
正在生成论文摘要