Classifier Discrepancy Guided Soft-Weight Adaptation Network for Machinery Fault Diagnosis Under Domain and Category Shift
IEEE TRANSACTIONS ON INSTRUMENTATION AND MEASUREMENT(2023)
摘要
Cross-domain diagnosis approaches based on transfer learning have received considerable attention in the past few years. Conventional closed-set diagnosis tasks, where the fault modes of source and target machines are consistent, are effectively addressed by existing methods. However, the closed-set assumption is difficult to hold in actual applications because different machines usually have different fault modes in real industries. To handle the fault diagnosis problem with category shift, this study proposes a novel classifier discrepancy-guided soft-weight adaptation network (CDSAN). The network incorporates two different state classifiers to extract domain-invariant discriminative features from different domains. An outlier classifier is constructed to perform pseudolabel learning on target samples, effectively separating shared-class and unknown-class samples between domains. On the basis of the entropy of the class likelihood, a sample-wise soft-weight term, which can adaptatively measure the similarity of target samples attributed to shared label space, is constructed. After that, a weighted classifier discrepancy loss is designed to bridge the domain shift and to achieve known-class alignment across domains. The experimental results conducted on two real datasets with diverse diagnostic tasks demonstrate that the proposed network offers a promising solution for fault diagnosis problems in the presence of domain and category shift.
更多查看译文
关键词
Fault diagnosis,Task analysis,Machinery,Feature extraction,Industries,Employee welfare,Adaptation models,Classifier discrepancy,deep learning (DL),domain adaptation (DA),domain and category shift,machinery fault diagnosis
AI 理解论文
溯源树
样例
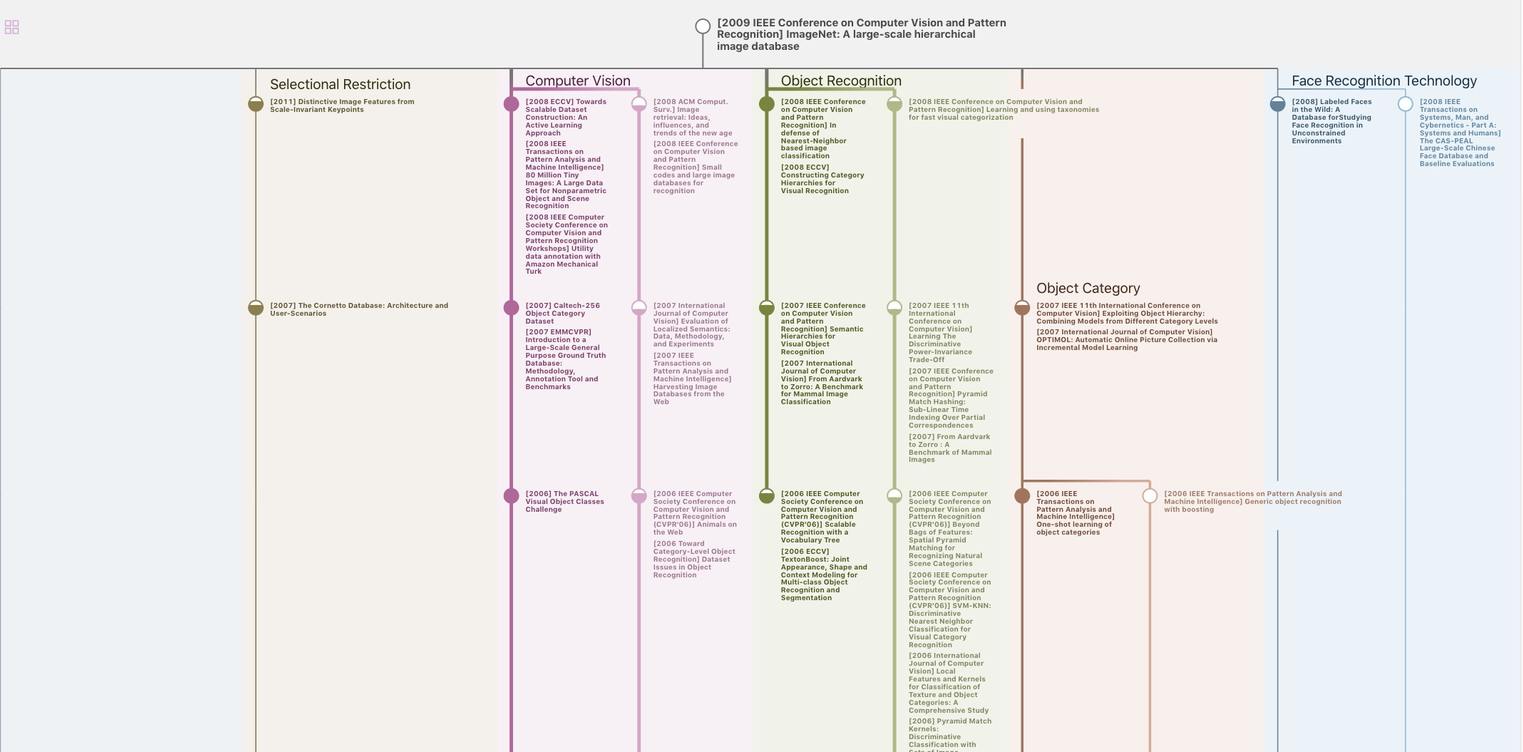
生成溯源树,研究论文发展脉络
Chat Paper
正在生成论文摘要