Constrained Adaptive Distillation Based on Topological Persistence for Wearable Sensor Data
IEEE TRANSACTIONS ON INSTRUMENTATION AND MEASUREMENT(2023)
摘要
Wearable sensor data analysis with persistence features generated by topological data analysis (TDA) has achieved great success in various applications, and however, it suffers from large computational and time resources for extracting topological features. In this article, our approach utilizes knowledge distillation (KD) that involves the use of multiple teacher networks trained with the raw time series and persistence images (PIs) generated by TDA. However, direct transfer of knowledge from the teacher models utilizing different characteristics as inputs to the student model results in a knowledge gap and limited performance. To address this problem, we introduce a robust framework that integrates multimodal features from two different teachers and enables a student to learn desirable knowledge effectively. To account for statistical differences in multimodalities, an entropy-based constrained adaptive weighting mechanism is leveraged to automatically balance the effects of teachers and encourage the student model to adequately adopt the knowledge from two teachers. To assimilate dissimilar structural information generated by different style models for distillation, batch and channel similarities within a mini-batch are used. We demonstrate the effectiveness of the proposed method on wearable sensor data.
更多查看译文
关键词
Machine learning,Wearable sensors,Data analysis,Knowledge distillation (KD),topological data analysis (TDA),wearable sensor data
AI 理解论文
溯源树
样例
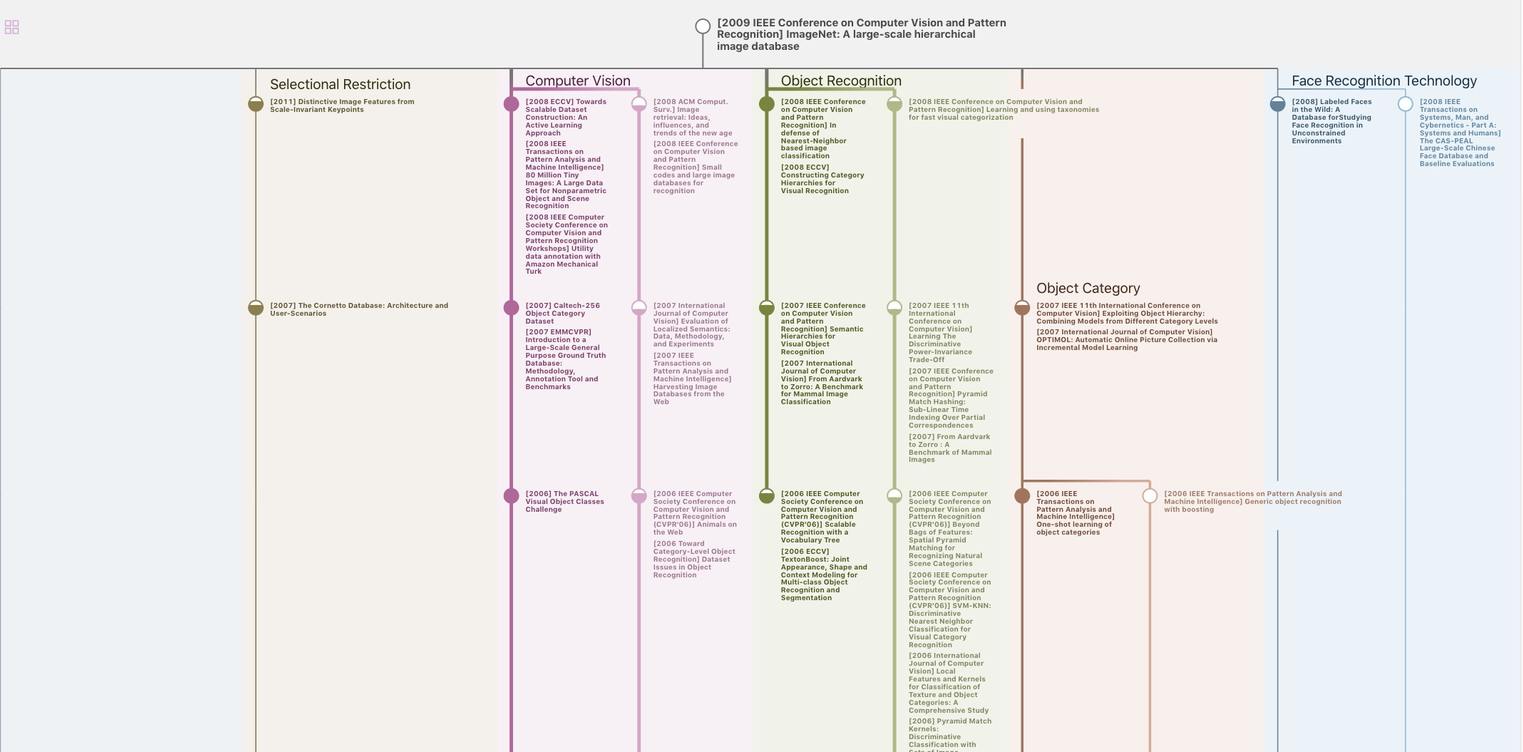
生成溯源树,研究论文发展脉络
Chat Paper
正在生成论文摘要