3-D Joint Inversion of Gravity and Magnetic Data Based on a Deep Learning Network With Automatic Recognition of Structural Similarity
IEEE TRANSACTIONS ON GEOSCIENCE AND REMOTE SENSING(2023)
摘要
The data-driven inversion methods based on deep learning (DL) for gravity and magnetic data have been well studied in existing literature due to their advantages such as less constraint requirements and extremely high efficiency compared with various conventional model-driven inversion methods. However, some issues should be further addressed when these methods are applied to the joint inversion, such as how to determine the structural similarity of the divided cells and low depth resolution of the inversion results. To solve the above problems, this article proposes an optimized DL network for the joint inversion of gravity and magnetic data. We designed a network with combined modules, which can extract features of structural similarity and the mapping relationship between raw potential field data and physical parameters at multiple scales. Based on this function, the network improves the accuracy of the joint inversion results by adopting different inversion strategies (independent or joint) in different areas. In addition, the proposed method uses the 3-D convolution operator to screen and reconstruct the physical parameters, with which the depth resolution of the results can be improved. Numerical examples using synthetic and real data of a metallic deposit area in Northwest China illustrate the effectiveness and advantage of the proposed method.
更多查看译文
关键词
Deep learning (DL),gravity,joint inversion,magnetic
AI 理解论文
溯源树
样例
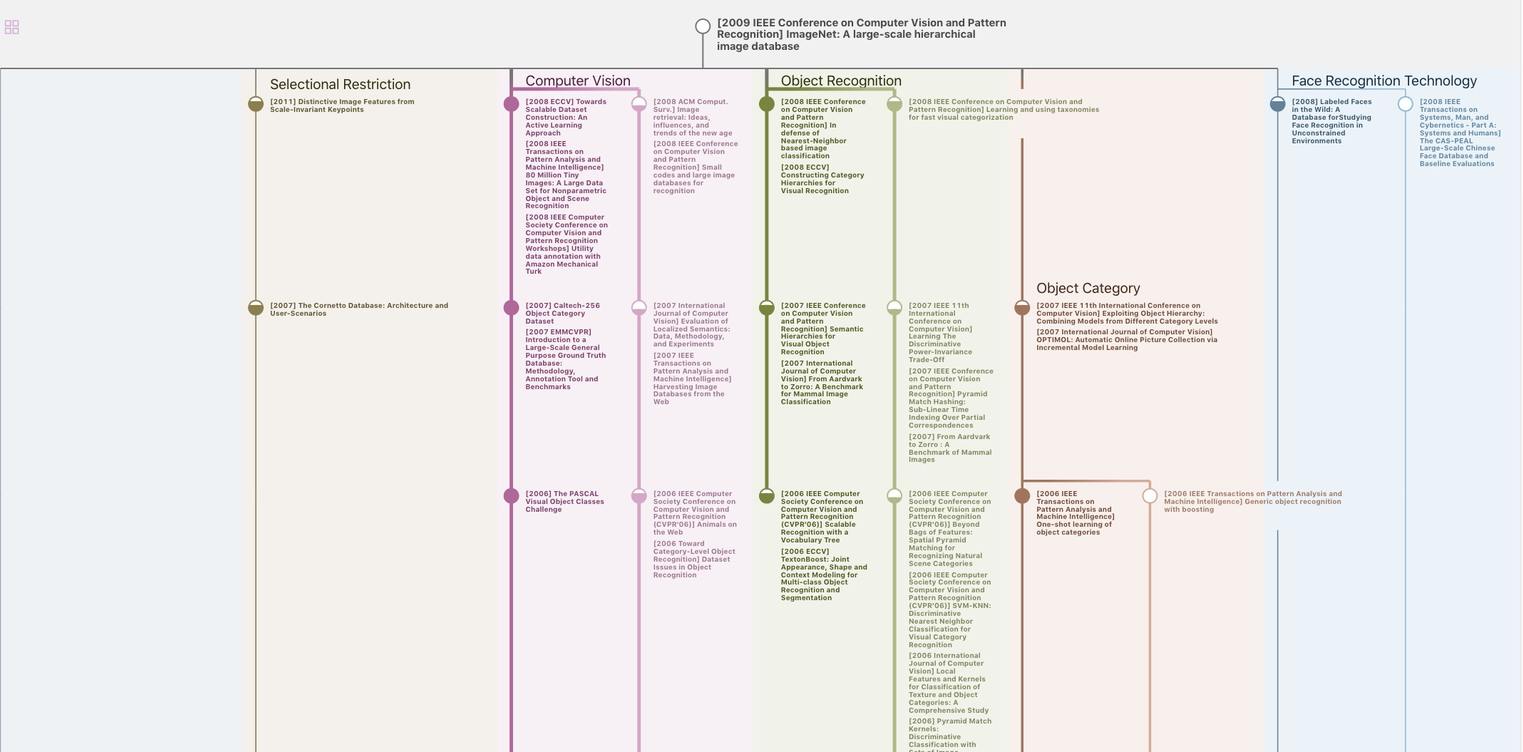
生成溯源树,研究论文发展脉络
Chat Paper
正在生成论文摘要