Fully Sparse Transformer 3-D Detector for LiDAR Point Cloud
IEEE TRANSACTIONS ON GEOSCIENCE AND REMOTE SENSING(2023)
摘要
The 3-D object detector usually uses a framework similar to 2-D detection and benefits from the advancements of 2-D detection tasks. In these frameworks, it is necessary to make the unstructured, sparse point cloud features into dense grids to be compatible with popular 2-D operators, such as convolution and transformers, which also causes extra computational costs. In this article, we propose a simple and efficient Fully Sparse TRansformer (FSTR) for light detection and ranging (LiDAR)-based 3-D object detection, which is able to combine with state-of-the-art (SOTA) sparse backbones to form a fully sparse, end-to-end, simple, and efficient detection framework. FSTR uses the sparse voxel feature from the sparse backbone as the input token without any custom operators. Furthermore, we introduce the dynamic queries (DQs) to provide a priori location and context of the foreground for the decoder and drop the high-confidence background tokens to further reduce redundant computations. We propose Gaussian denoising queries to speed up the decoder training and make it more adaptable to the distribution of sparse voxel features. Extensive experiments on the nuScenes benchmark and the Argoverse2 (AV2) benchmark validate the effectiveness of the proposed method. FSTR outperforms all LiDAR real-time methods by 69.5 mean average precision (mAP) and 72.9 nuScenes detection score (NDS) on the official benchmark of nuScenes dataset. On the long-range detection benchmark AV2, the proposed method achieves a new SOTA performance of 39.9 mAP, which outperforms the existing LiDAR detectors, even the LiDAR-camera detectors by a large margin (+9.4 and +7.5 mAP), showing the great advantage of the proposed method for long-range detection.
更多查看译文
关键词
3-D detection,light detection and ranging (LiDAR),point cloud,sparse,transformer
AI 理解论文
溯源树
样例
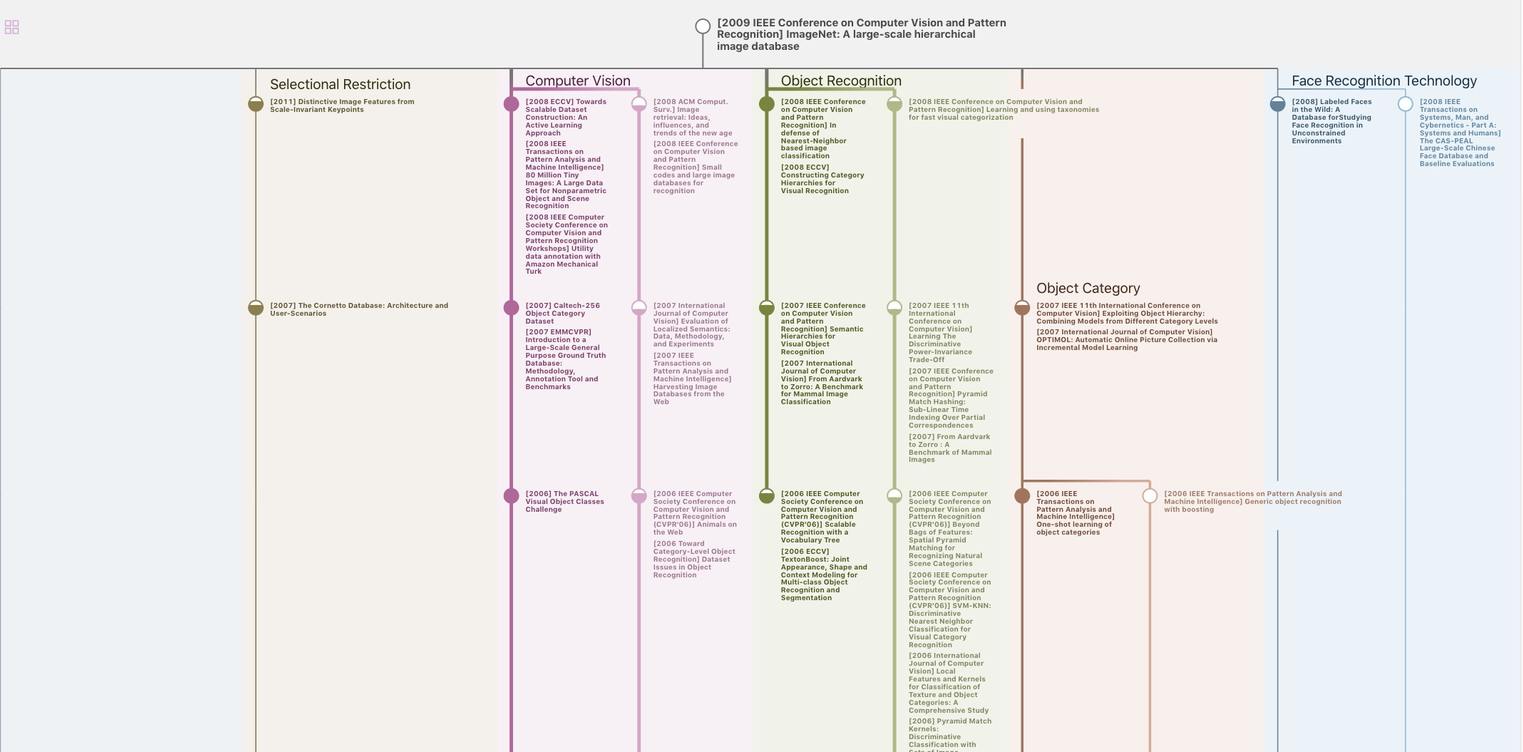
生成溯源树,研究论文发展脉络
Chat Paper
正在生成论文摘要