Robust Tensor Low-Rank Sparse Representation With Saliency Prior for Hyperspectral Anomaly Detection
IEEE TRANSACTIONS ON GEOSCIENCE AND REMOTE SENSING(2023)
摘要
Recently, hyperspectral anomaly detection (HAD) methods based on tensor low-rank representation (TLRR) have received widespread attention. However, most of them tend to emphasize the utilization of multiple types of prior knowledge to characterize background components, while the prior information about anomaly components is limited. In addition, the constructed background dictionary is also susceptible to noise and outliers. To address these challenges, this article focuses on both the background and abnormal components, proposing a robust tensor low-rank sparse representation with saliency prior (RTLSR-SP) method for HAD. Specifically, for the background component described by the dictionary tensor and the corresponding coefficient tensor, tensor nuclear norm (TNN) constraint and sparsity constraint are imposed on the coefficient tensor simultaneously to capture the global and local spatial-spectral structure information of the hyperspectral image (HSI), respectively. For the anomalous component, we design a sparse saliency prior weight tensor to enhance the saliency of anomalous targets. Meanwhile, the tensor l(F,1)-norm is also integrated into the model to better separate abnormal targets from the background. Furthermore, combining tensor robust principal component analysis (TRPCA) and skinny tensor singular value decomposition (skinny t-SVD), a robust background dictionary is constructed. Finally, an efficient iterative algorithm based on the alternating direction method of multipliers (ADMM) is derived to optimize the RTLSR-SP model. Comprehensive experimental findings on one simulated dataset and six real hyperspectral datasets demonstrate the effectiveness and superiority of the proposed algorithm compared with eight state-of-the-art HAD algorithms.
更多查看译文
关键词
Anomaly detection,hyperspectral image (HSI),sparse saliency prior weight tensor,tensor low-rank sparse representation (SR),tensor robust principal component analysis (TRPCA)
AI 理解论文
溯源树
样例
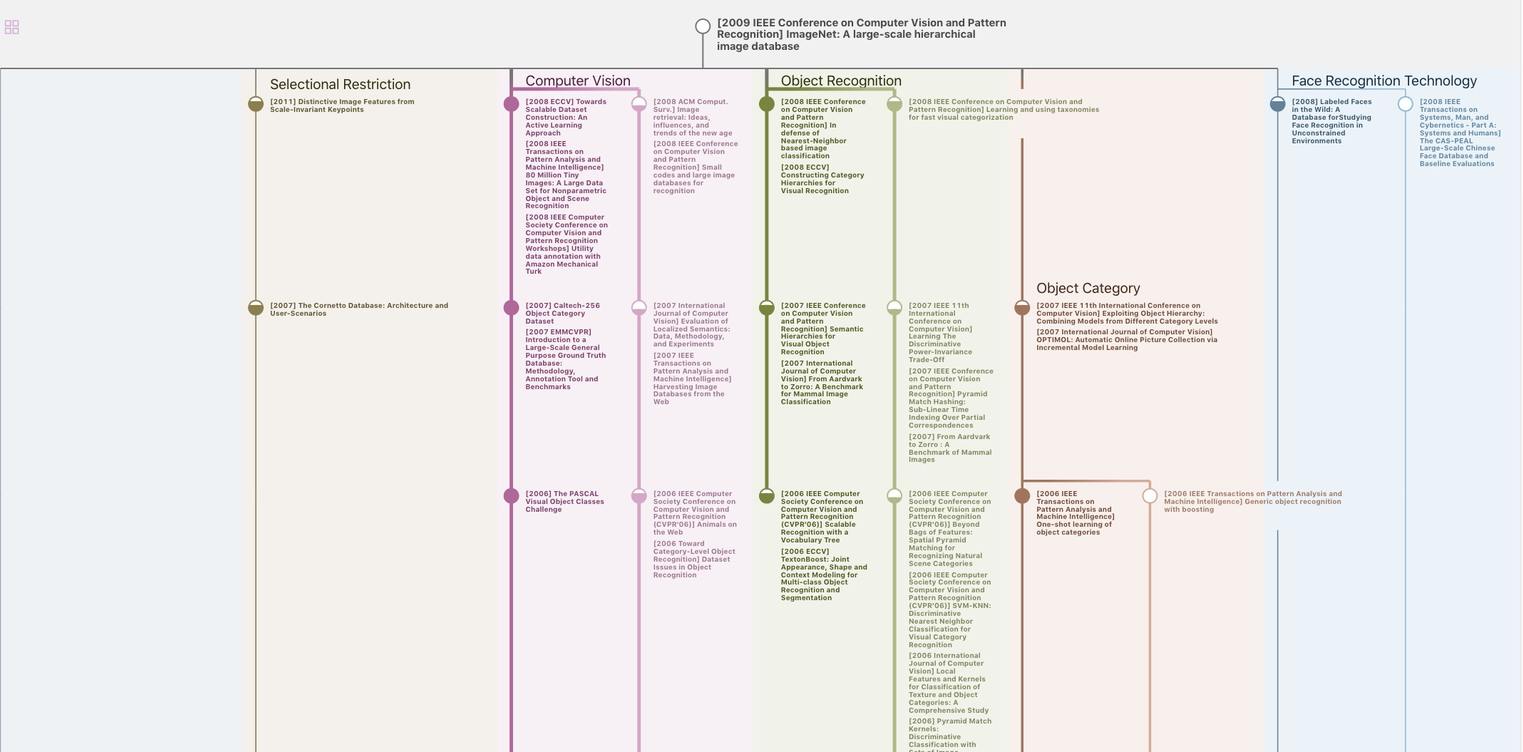
生成溯源树,研究论文发展脉络
Chat Paper
正在生成论文摘要